Detecting Label Noise via Leave-One-Out Cross Validation
arxiv(2021)
摘要
We present a simple algorithm for identifying and correcting real-valued noisy labels from a mixture of clean and corrupted samples using Gaussian process regression. A heteroscedastic noise model is employed, in which additive Gaussian noise terms with independent variances are associated with each and all of the observed labels. Thus, the method effectively applies a sample-specific Tikhonov regularization term, generalizing the uniform regularization prevalent in standard Gaussian process regression. Optimizing the noise model using maximum likelihood estimation leads to the containment of the GPR model's predictive error by the posterior standard deviation in leave-one-out cross-validation. A multiplicative update scheme is proposed for solving the maximum likelihood estimation problem under non-negative constraints. While we provide a proof of monotonic convergence for certain special cases, the multiplicative scheme has empirically demonstrated monotonic convergence behavior in virtually all our numerical experiments. We show that the presented method can pinpoint corrupted samples and lead to better regression models when trained on synthetic and real-world scientific data sets.
更多查看译文
关键词
label noise,leave-one-out,cross-validation
AI 理解论文
溯源树
样例
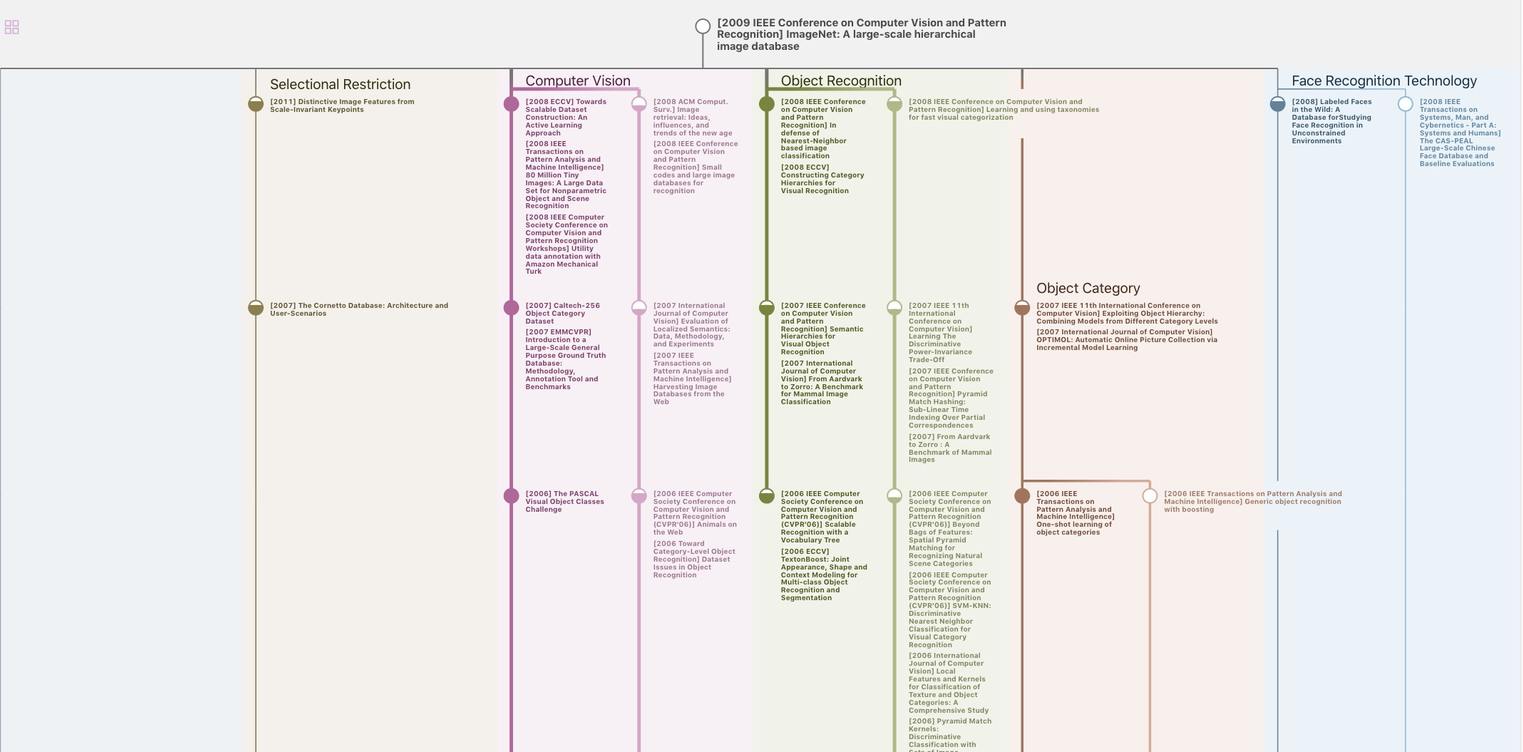
生成溯源树,研究论文发展脉络
Chat Paper
正在生成论文摘要