Differentially private inference via noisy optimization
ANNALS OF STATISTICS(2023)
摘要
We propose a general optimization-based framework for computing differentially private M-estimators and a new method for constructing differentially private confidence regions. First, we show that robust statistics can be used in conjunction with noisy gradient descent or noisy Newton methods in order to obtain optimal private estimators with global linear or quadratic convergence, respectively. We establish local and global convergence guarantees, under both local strong convexity and self-concordance, showing that our private estimators converge with high probability to a small neighborhood of the nonprivate M-estimators. Second, we tackle the problem of parametric inference by constructing differentially private estimators of the asymptotic variance of our private M-estimators. This naturally leads to approximate pivotal statistics for constructing confidence regions and conducting hypothesis testing. We demonstrate the effectiveness of a bias correction that leads to enhanced small-sample empirical performance in simulations. We illustrate the benefits of our methods in several numerical examples.
更多查看译文
关键词
Differential privacy,M -estimation,statistical inference,gradient descent,Newton's method
AI 理解论文
溯源树
样例
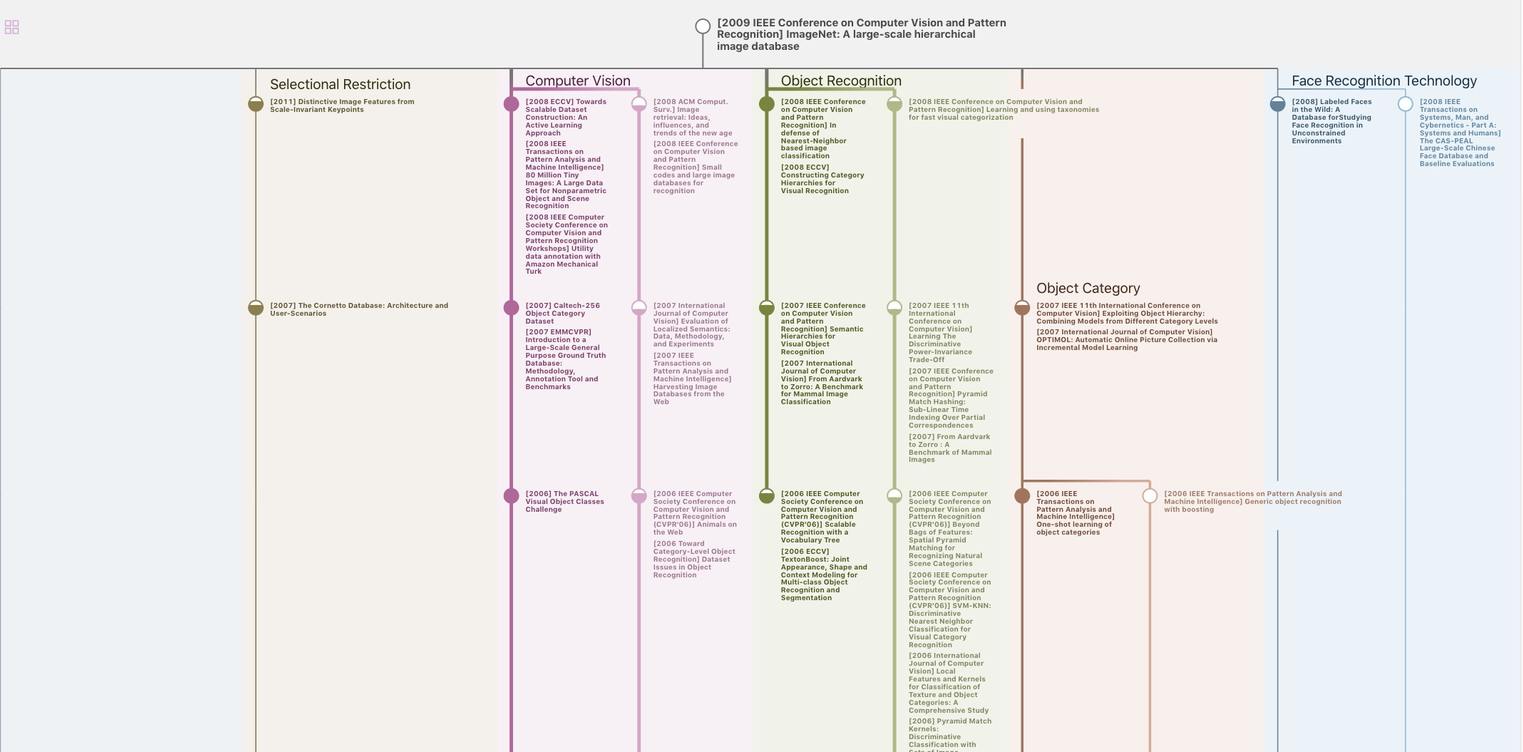
生成溯源树,研究论文发展脉络
Chat Paper
正在生成论文摘要