Efficient Visual Pretraining with Contrastive Detection.
ICCV(2021)
摘要
Self-supervised pretraining has been shown to yield powerful representations for transfer learning. These performance gains come at a large computational cost however, with state-of-the-art methods requiring an order of magnitude more computation than supervised pretraining. We tackle this computational bottleneck by introducing a new self-supervised objective, contrastive detection, which tasks representations with identifying object-level features across augmentations. This objective extracts a rich learning signal per image, leading to state-of-the-art transfer performance from ImageNet to COCO, while requiring up to 5x less pretraining. In particular, our strongest ImageNet-pretrained model performs on par with SEER, one of the largest self-supervised systems to date, which uses 1000x more pretraining data. Finally, our objective seamlessly handles pretraining on more complex images such as those in COCO, closing the gap with supervised transfer learning from COCO to PASCAL.
更多查看译文
关键词
contrastive detection,visual
AI 理解论文
溯源树
样例
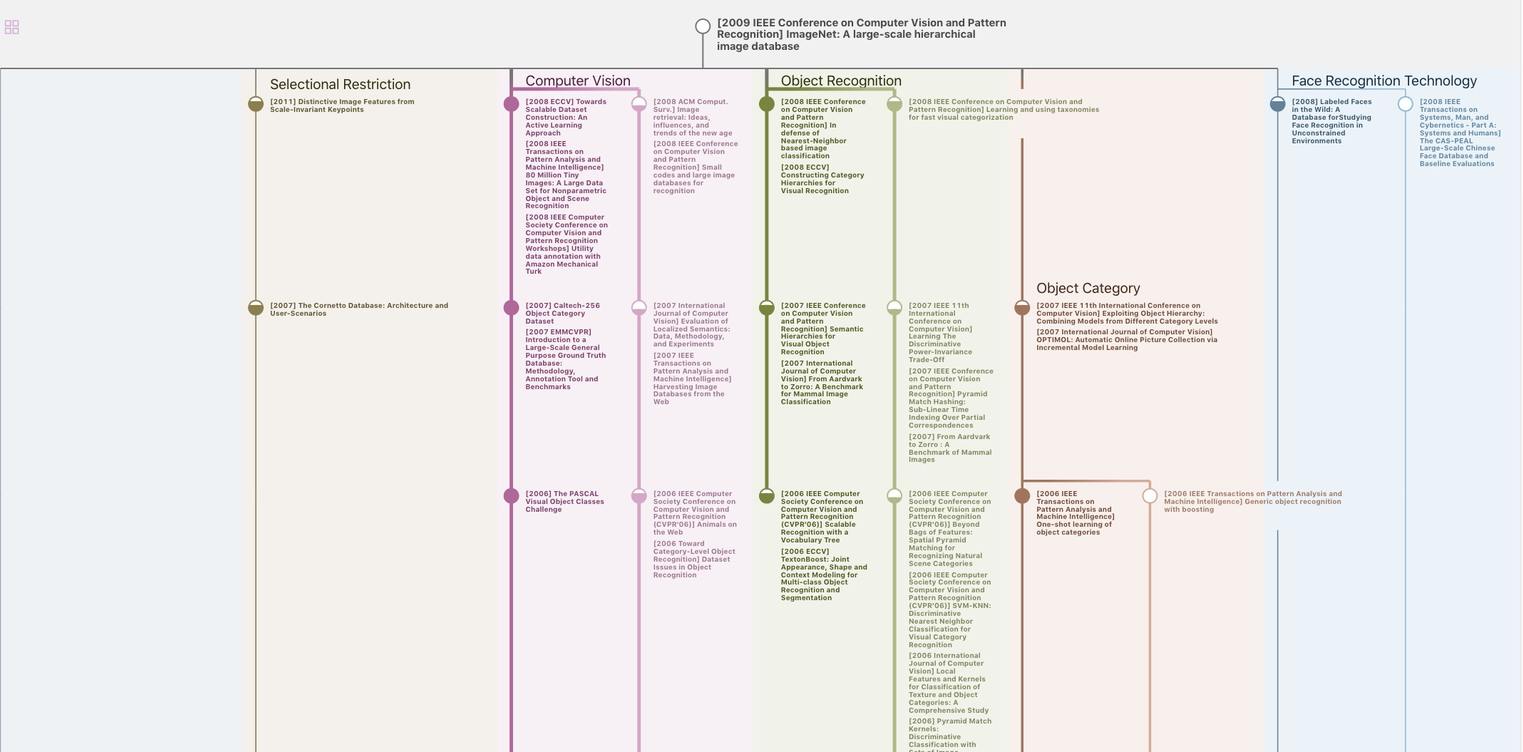
生成溯源树,研究论文发展脉络
Chat Paper
正在生成论文摘要