Privacy Preserving Misbehavior Detection in IoV Using Federated Machine Learning
2021 IEEE 18th Annual Consumer Communications & Networking Conference (CCNC)(2021)
摘要
Data falsification attack in Vehicular Ad hoc Networks (VANET) for the Internet of Vehicles (IoV) is achieved by corrupting the data exchanged between nodes with false information. Data is the most valuable asset these days from which many analyses and results can be drawn out. But the privacy concern raised by users has become the greatest hindrance in performing data analysis. In IoV, misbehavior detection can be performed by creating a machine learning model from basic safety message (BSM) dataset of vehicles. We propose a privacy-preserving misbehavior detecting system for IoV using Federated Machine Learning. Vehicles in VANET for IoV are given the initial dull model to locally train using their own local data. On doing this we get a collective smart model that can classify Position Falsification attack in VANET using the data generated by each vehicle. All this is done without actually sharing the data with any third party to perform analysis. In this paper, we compare the performance of the attack detection model trained by using a federated and central approach. This training method trains the model on a different kind of position falsification attack by using local BSM data generated on each vehicle.
更多查看译文
关键词
VANET,Federate Learning,Data Falsification Attack,Privacy
AI 理解论文
溯源树
样例
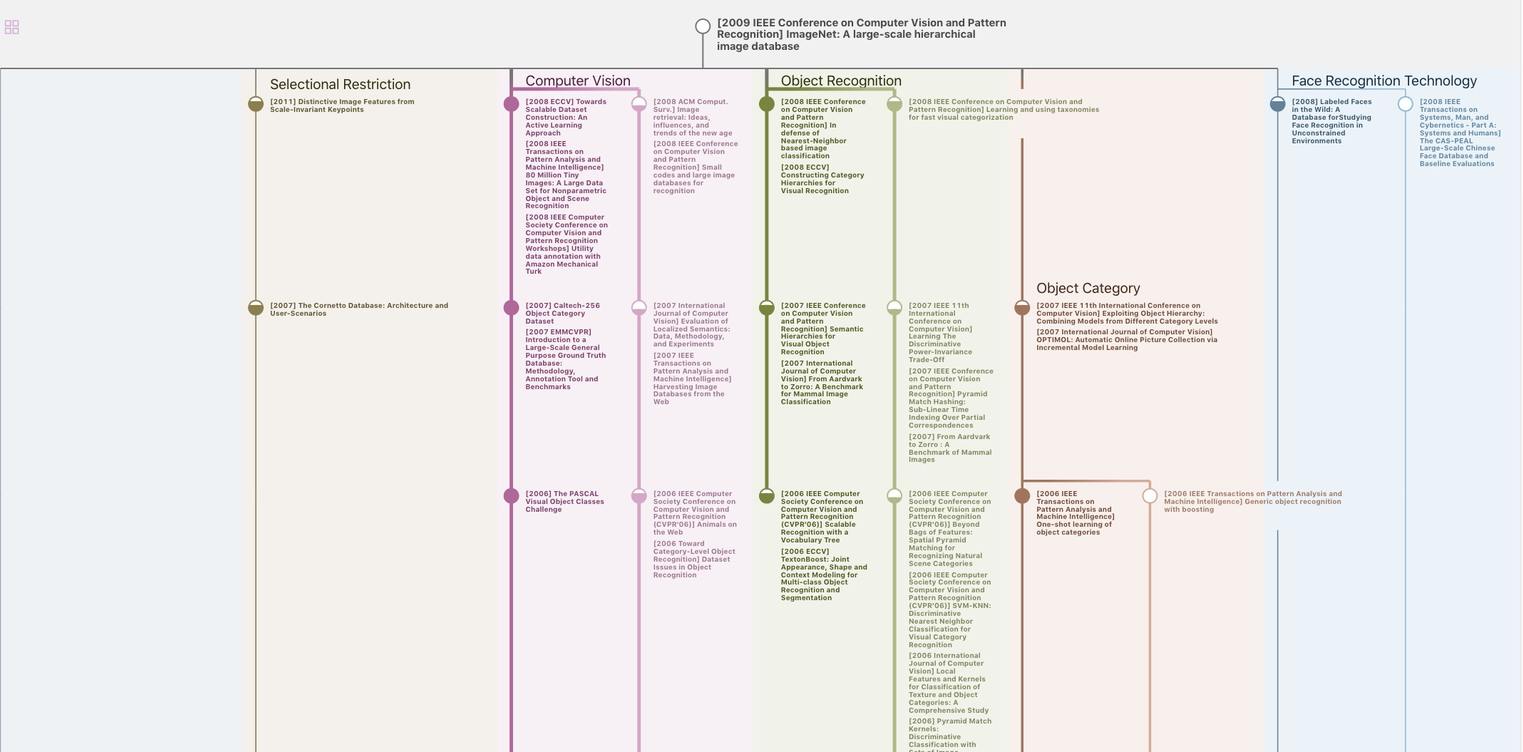
生成溯源树,研究论文发展脉络
Chat Paper
正在生成论文摘要