Ontology-Based Retrieval & Neural Approaches for BioASQ Ideal Answer Generation
user-5edf3a5a4c775e09d87cc848(2018)
摘要
The ever-increasing magnitude of biomedical information sources makes it difficult and time-consuming for a human researcher to find the most relevant documents and pinpointed answers for a specific question or topic when using only a traditional search engine. Biomedical Question Answering systems automatically identify the most relevant documents and pinpointed answers, given an information need expressed as a natural language question. Generating a non-redundant, human-readable summary that satisfies the information need of a given biomedical question is the focus of the Ideal Answer Generation task, part of the BioASQ challenge. This paper presents a system for ideal answer generation (using ontology-based retrieval and a neural learning-to-rank approach, combined with extractive and abstractive summarization techniques) which achieved the highest ROUGE score of 0.659 on the BioASQ 5b batch 2 test.
更多查看译文
关键词
Question answering,Automatic summarization,Information needs,Ontology,Natural language,Information retrieval,Computer science,Search engine,Biomedical information
AI 理解论文
溯源树
样例
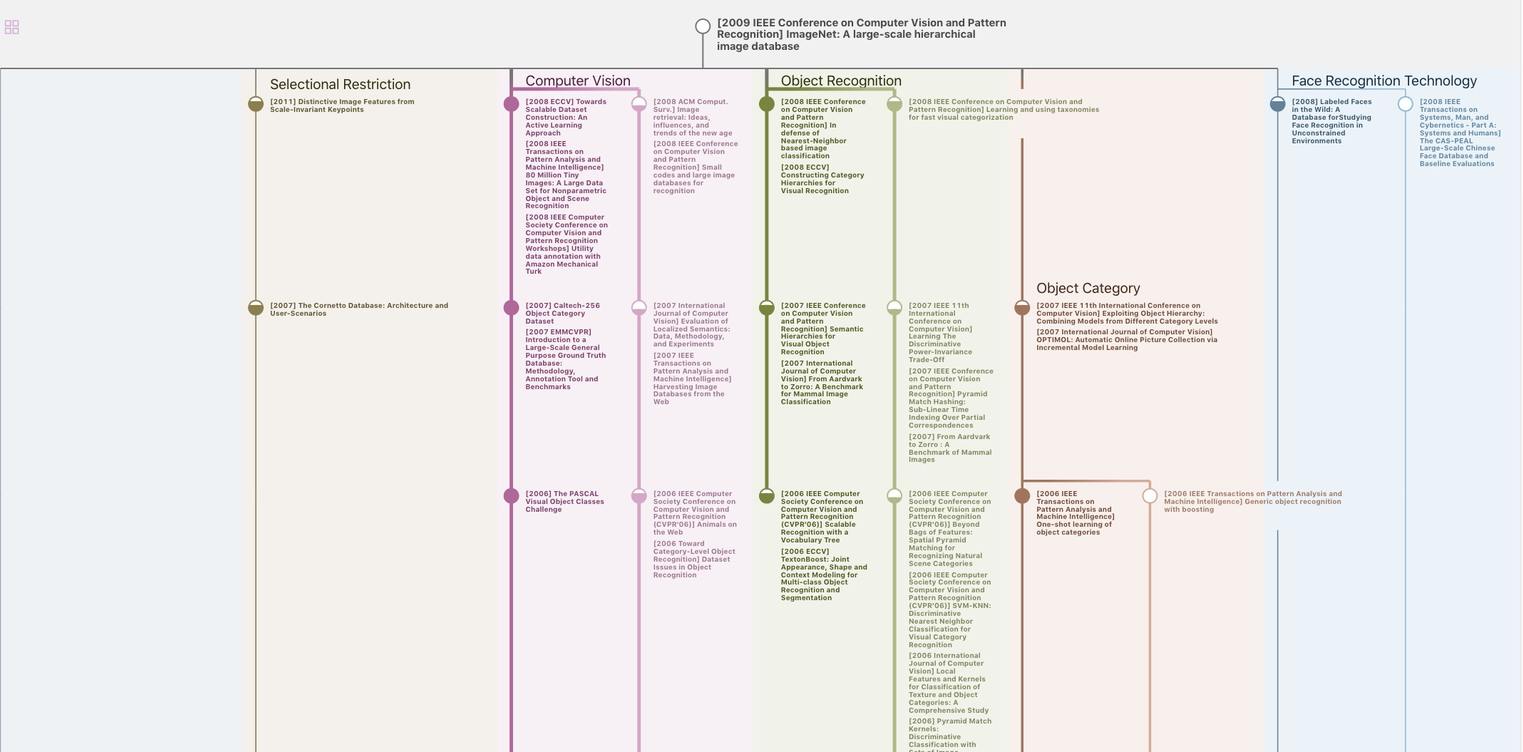
生成溯源树,研究论文发展脉络
Chat Paper
正在生成论文摘要