Adversarial Curiosity
user-5bd69975530c70d56f390249(2020)
摘要
Model-based curiosity combines active learning approaches to optimal sampling with the information gain based incentives for exploration presented in the curiosity literature. Existing model-based curiosity methods look to approximate prediction uncertainty with approaches which struggle to scale to many prediction-planning pipelines used in robotics tasks. We address these scalability issues with an adversarial curiosity method minimizing a score given by a discriminator network. This discriminator is optimized jointly with a prediction model and enables our active learning approach to sample sequences of observations and actions which result in predictions considered the least realistic by the discriminator. We demonstrate progressively increasing advantages as compute is restricted of our adversarial curiosity approach over leading model-based exploration strategies in simulated environments. We further demonstrate the ability of our adversarial curiosity method to scale to a robotic manipulation prediction-planning pipeline where we improve sample efficiency and prediction performance for a domain transfer problem.
更多查看译文
关键词
Curiosity,Adversarial system,Epistemology,Psychology
AI 理解论文
溯源树
样例
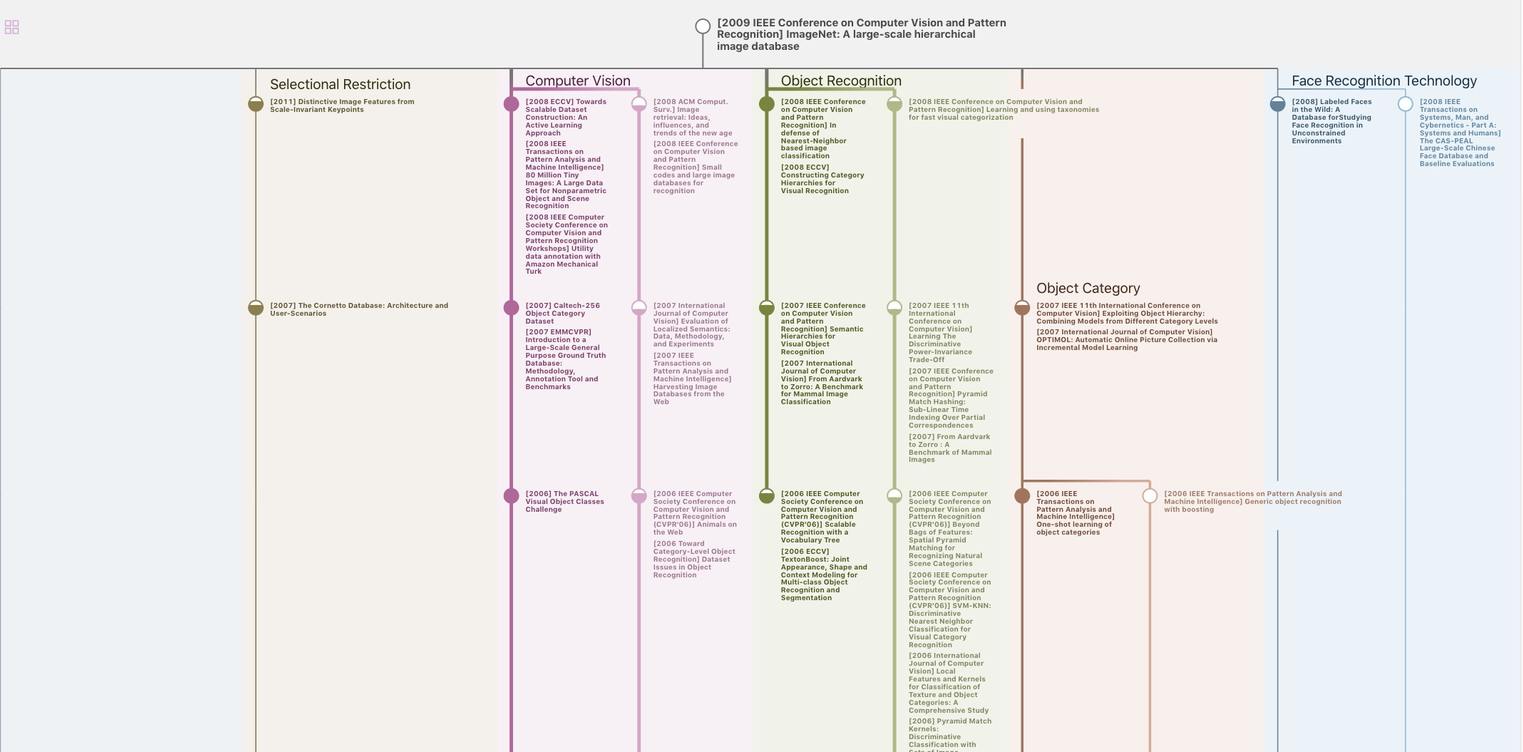
生成溯源树,研究论文发展脉络
Chat Paper
正在生成论文摘要