Support Vector Machines For Oil Classification Link With Polyaromatic Hydrocarbon Contamination In The Environment
WATER SCIENCE AND TECHNOLOGY(2021)
摘要
The main focus of this study is exploring the spatial distribution of polyaromatics hydrocarbon links between oil spills in the environment via Support Vector Machines based on Kernel-Radial Basis Function (RBF) approach for high precision classification of oil spill type from its sample fingerprinting in Peninsular Malaysia. The results show the highest concentrations of sigma Alkylated PAHs and sigma EPA PAHs in sigma TAH concentration in diesel from the oil samples PP3_liquid and GP6_Jetty achieving 100% classification output, corresponding to coherent decision boundary and projective subspace estimation. The high dimensional nature of this approach has led to the existence of a perfect separability of the oil type classification from four clustered oil type components; i.e diesel, bunker C, Mixture Oil (MO), lube oil and Waste Oil (WO) with the slack variables of xi not equal 0. Of the four clusters, only the SVs of two are correctly predicted, namely diesel and MO. The kernel-RBF approach provides efficient and reliable oil sample classification, enabling the oil classification to be optimally performed within a relatively short period of execution and a faster dataset classification where the slack variables xi are non-zero.
更多查看译文
关键词
classifier, fingerprints, oil classification, support vector machines
AI 理解论文
溯源树
样例
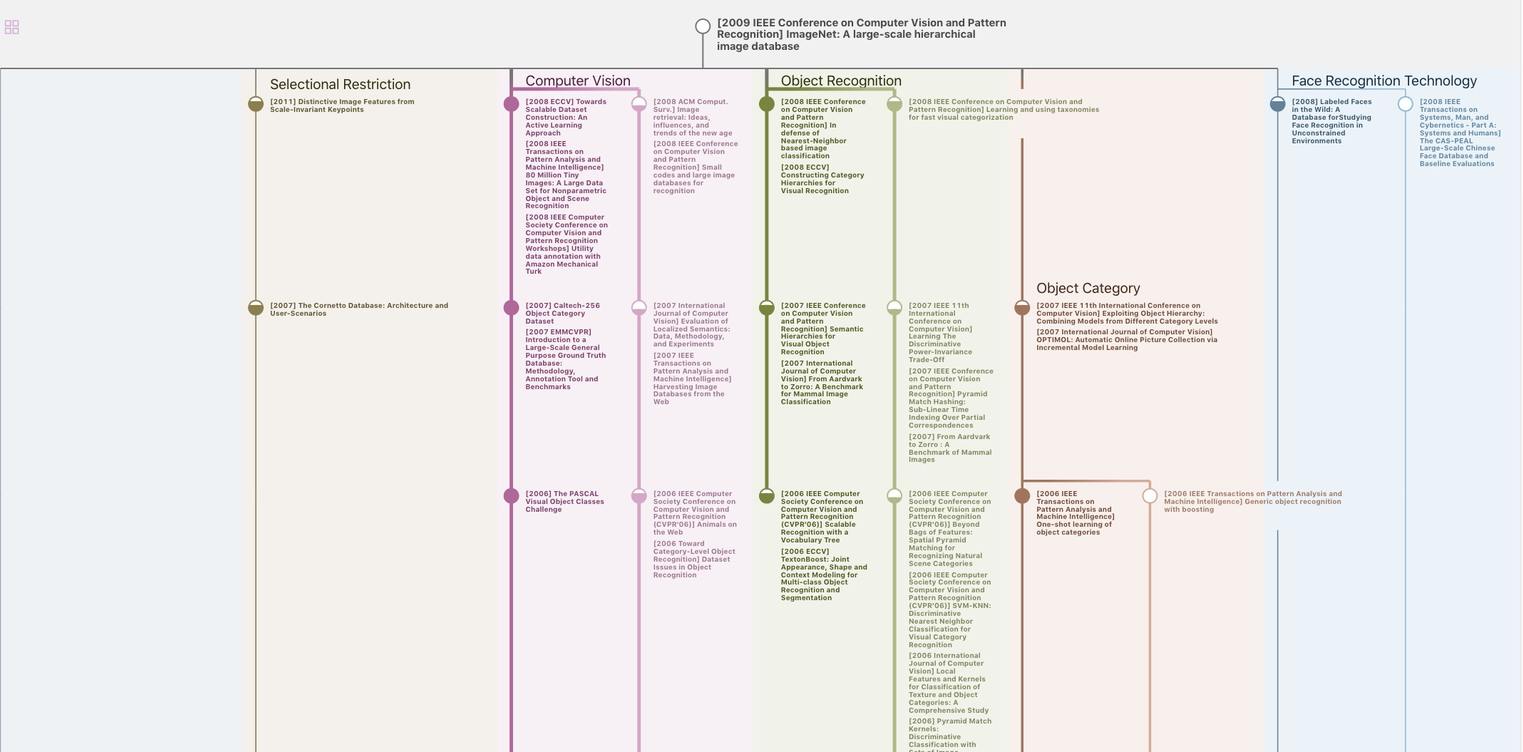
生成溯源树,研究论文发展脉络
Chat Paper
正在生成论文摘要