WheatNet: A lightweight convolutional neural network for high-throughput image-based wheat head detection and counting
Neurocomputing(2022)
摘要
For a globally recognized plant breeding organization, manually recorded field observation data is crucial for plant breeding decision making. However, certain phenotypic traits such as plant color, height, kernel counts, etc. can only be recorded during a specific time-window of a crop’s growth cycle. Due to labor-intensive requirements, only a small subset of possible field observations are recorded each season. To help mitigate this data collection bottleneck in wheat breeding, we propose a novel deep learning framework to accurately and efficiently count wheat heads to aid in the gathering of real-time data for decision making. We call our model WheatNet and show that our approach is robust and accurate for a wide range of environmental conditions of the wheat field. WheatNet uses a truncated MobileNetV2 as a lightweight backbone feature extractor which merges feature maps with different scales to counter image scale variations. Then, extracted multi-scale features go to two parallel sub-networks for simultaneous density-based counting and localization tasks. The proposed method uses only point-level annotations for both counting and localization which is less labor-intensive compared to box-level annotations. Our proposed method achieves an MAE and RMSE of 3.85 and 5.19 in our wheat head counting task, respectively, while having significantly fewer parameters when compared to other state-of-the-art methods. Our experiments and comparisons with other state-of-the-art methods demonstrate the superiority and effectiveness of our proposed method.
更多查看译文
关键词
Convolutional neural network,Deep learning,Agriculture,Yield estimation
AI 理解论文
溯源树
样例
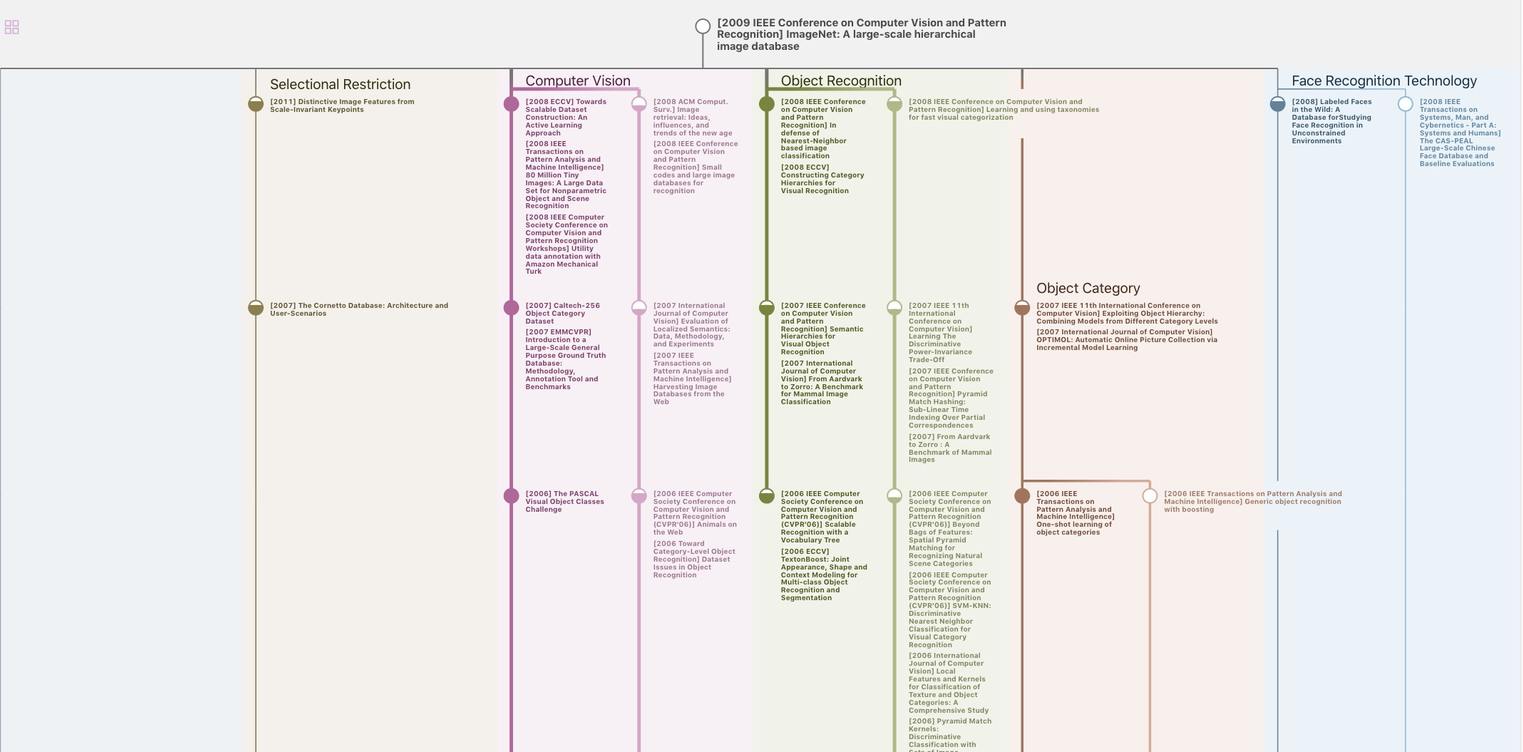
生成溯源树,研究论文发展脉络
Chat Paper
正在生成论文摘要