Co-generation and Segmentation for Generalized Surgical Instrument Segmentation on Unlabelled Data
MEDICAL IMAGE COMPUTING AND COMPUTER ASSISTED INTERVENTION - MICCAI 2021, PT IV(2021)
摘要
Surgical instrument segmentation for robot-assisted surgery is needed for accurate instrument tracking and augmented reality overlays. Therefore, the topic has been the subject of a number of recent papers in the CAI community. Deep learning-based methods have shown state-of-the-art performance for surgical instrument segmentation, but their results depend on labelled data. However, labelled surgical data is of limited availability and is a bottleneck in surgical translation of these methods. In this paper, we demonstrate the limited generalizability of these methods on different datasets, including robot-assisted surgeries on human subjects. We then propose a novel joint generation and segmentation strategy to learn a segmentation model with better generalization capability to domains that have no labelled data. The method leverages the availability of labelled data in a different domain. The generator does the domain translation from the labelled domain to the unlabelled domain and simultaneously, the segmentation model learns using the generated data while regularizing the generative model. We compared our method with state-of-the-art methods and showed its generalizability on publicly available datasets and on our own recorded video frames from robot-assisted prostatectomies. Our method shows consistently high mean Dice scores on both labelled and unlabelled domains when data is available only for one of the domains.
更多查看译文
关键词
Surgical instrument segmentation, Unpaired image to image translation, Generative adversarial learning
AI 理解论文
溯源树
样例
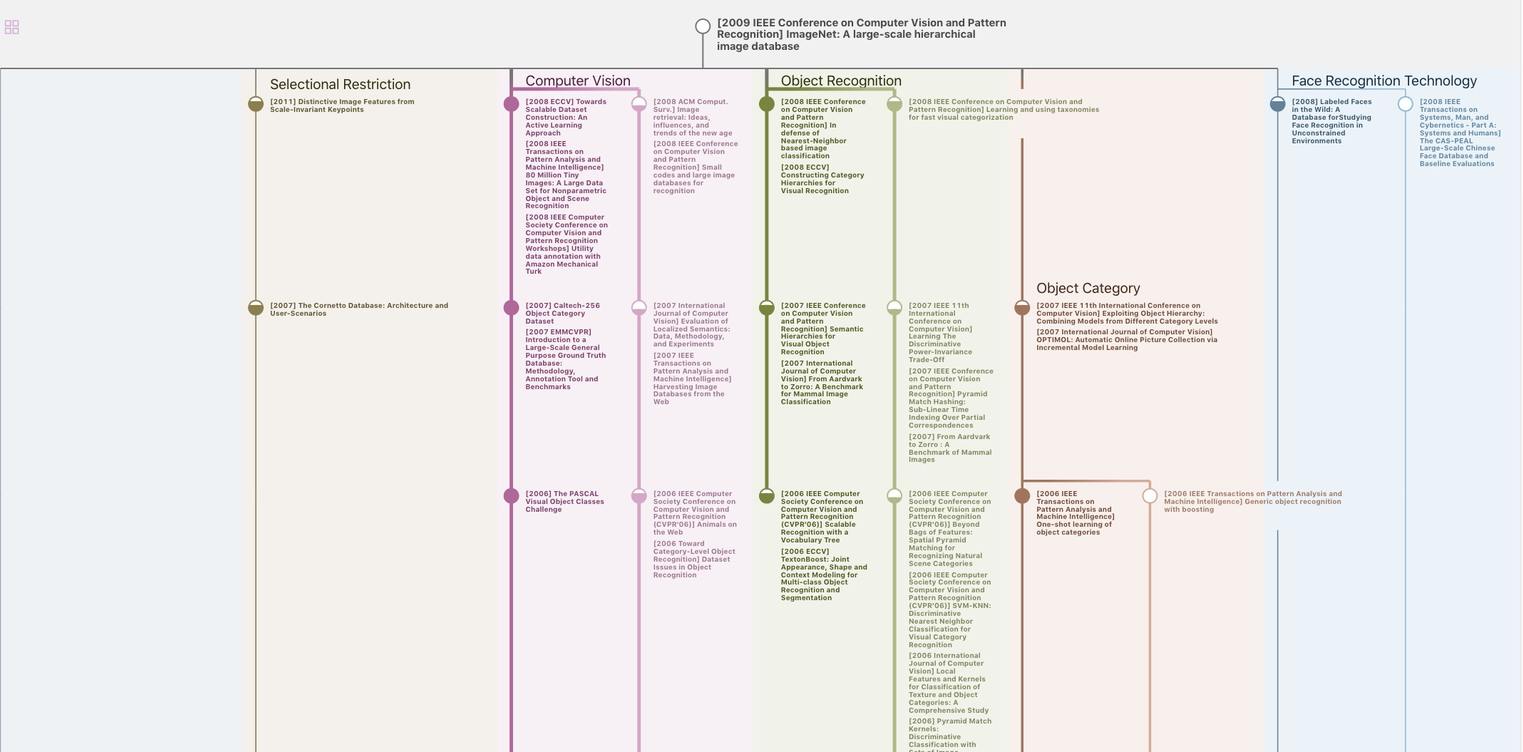
生成溯源树,研究论文发展脉络
Chat Paper
正在生成论文摘要