Multilingual Speech Translation From Efficient Finetuning Of Pretrained Models
59TH ANNUAL MEETING OF THE ASSOCIATION FOR COMPUTATIONAL LINGUISTICS AND THE 11TH INTERNATIONAL JOINT CONFERENCE ON NATURAL LANGUAGE PROCESSING, VOL 1 (ACL-IJCNLP 2021)(2021)
摘要
We present a simple yet effective approach to build multilingual speech-to-text (ST) translation through efficient transfer learning from a pretrained speech encoder and text decoder. Our key finding is that a minimalistic LNA (LayerNorm and Attention) finetuning can achieve zero-shot crosslingual and cross-modality transfer ability by only finetuning 10 similar to 50% of the pretrained parameters. This effectively leverages large pretrained models at low training cost such as wav2vec 2.0 for acoustic modeling, and mBART for multilingual text generation. This sets a new state-of-the-art for 36 translation directions (and surpassing cascaded ST for 30 of them) on the large-scale multilingual ST benchmark CoV-oST 2 (Wang et al., 2020b) (+6:4 BLEU on average for En-X directions and +6:7 BLEU for X-En directions). Our approach demonstrates strong zero-shot performance in a many-to-many multilingual model (+5:6 BLEU on average across 28 directions), making it an appealing approach for attaining high-quality speech translation with improved parameter and data efficiency.
更多查看译文
关键词
Speech translation,Transfer of learning,Encoder,Data efficiency,Speech recognition,Computer science,BLEU,Text generation,Transfer Ability
AI 理解论文
溯源树
样例
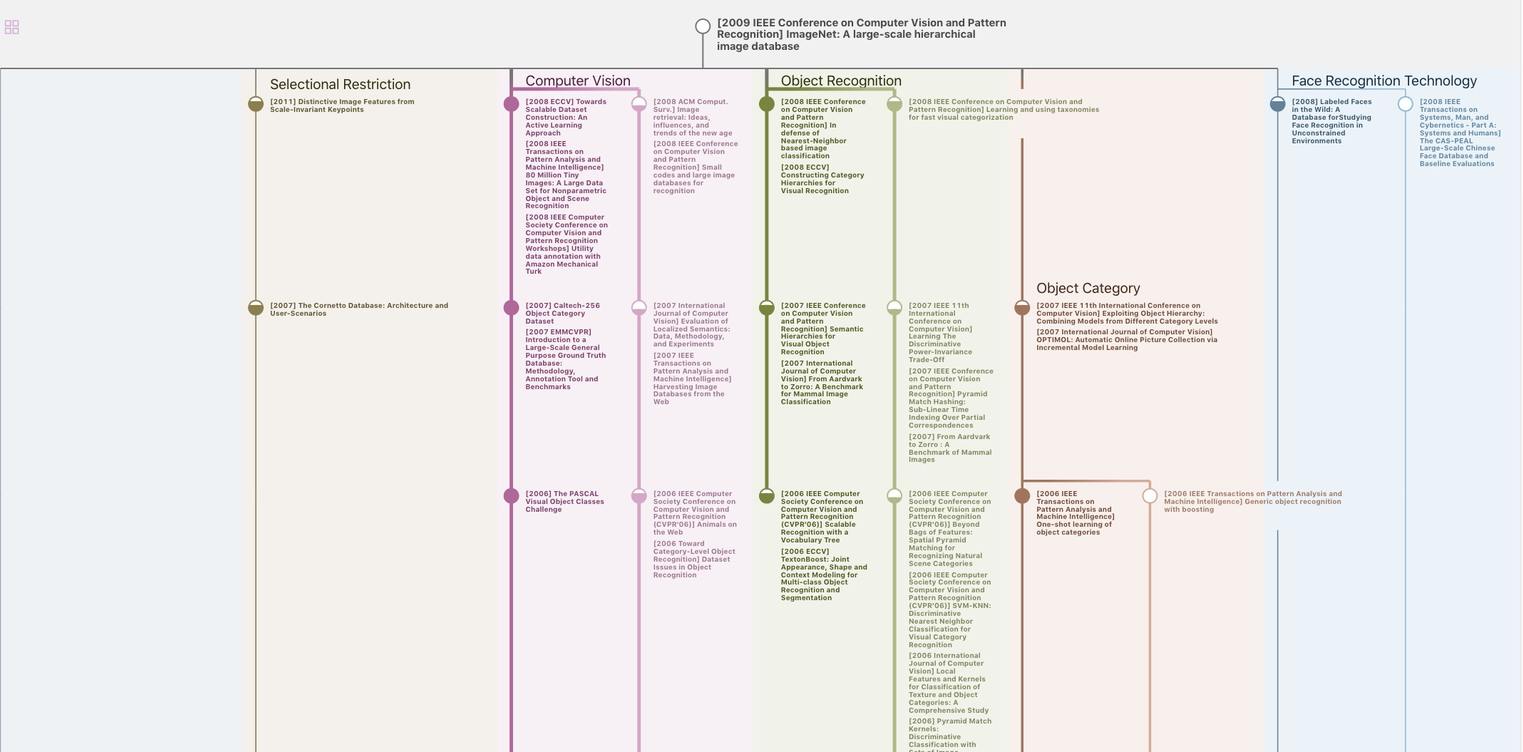
生成溯源树,研究论文发展脉络
Chat Paper
正在生成论文摘要