Dependency Structure Discovery from Interventions
user-5f8cf9244c775ec6fa691c99(2021)
摘要
Promising results have driven a recent surge of interest in continuous optimization methods for Bayesian network structure learning from observational data. However, there are theoretical limitations on the identifiability of underlying structures obtained from observational data alone. Interventional data provides much richer information about the underlying data-generating process. However, the extension and application of methods designed for observational data to include interventions is not straightforward and remains an open problem. In this paper we provide a general framework based on continuous optimization and neural networks to create models for the combination of observational and interventional data. The proposed method is applicable even in the challenging and realistic case that the identity of the intervened upon variable is unknown. We examine the proposed method in the setting of graph recovery both de novo and from a partially-known edge set. We establish strong benchmark results on several structure learning tasks, including structure recovery of both synthetic graphs as well as standard graphs from the Bayesian Network Repository.
更多查看译文
关键词
Bayesian network,Deep learning,Artificial neural network,Continuous optimization,Identifiability,Machine learning,Observational study,Open problem,Computer science,Psychological intervention,Artificial intelligence
AI 理解论文
溯源树
样例
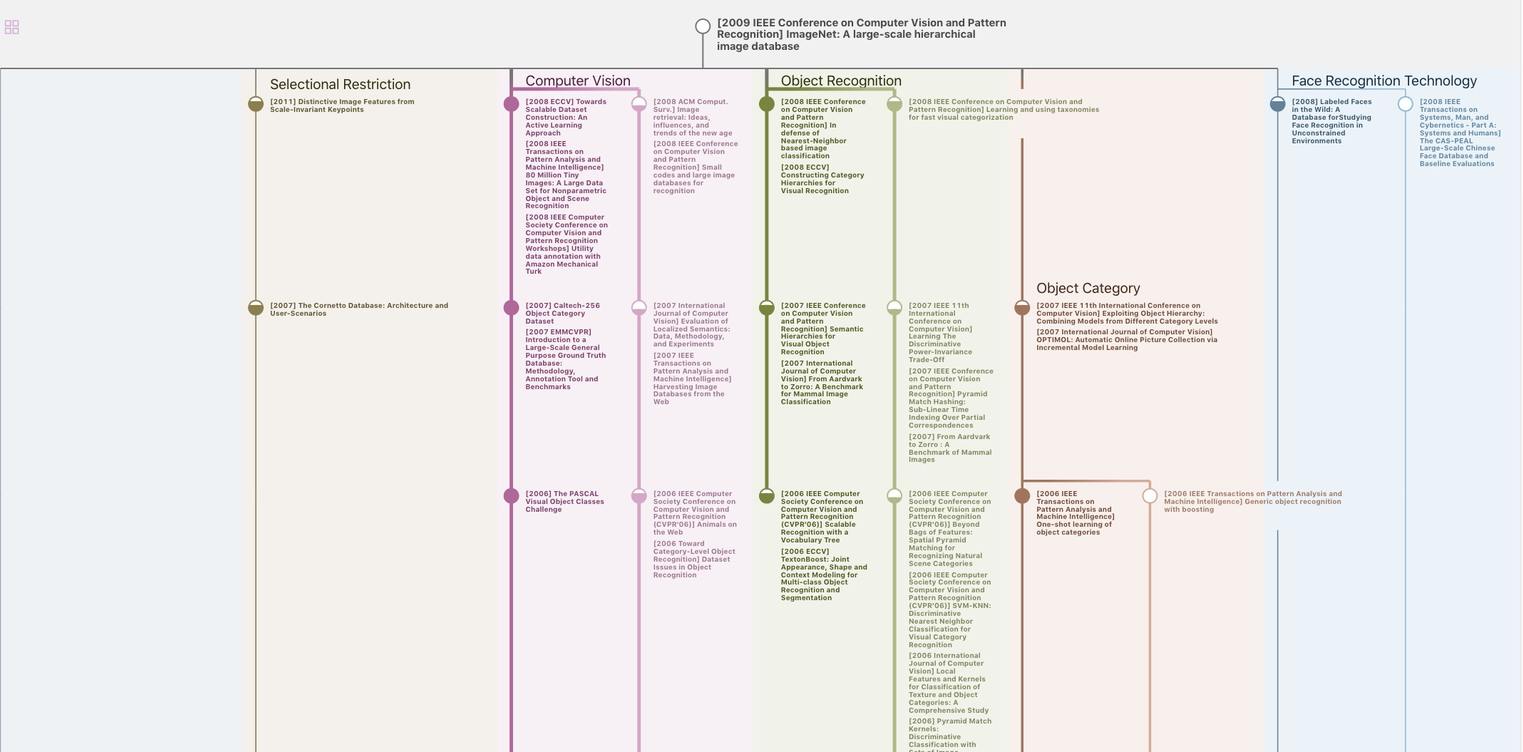
生成溯源树,研究论文发展脉络
Chat Paper
正在生成论文摘要