A Bayesian Multiscale Deep Learning Framework For Flows In Random Media
FOUNDATIONS OF DATA SCIENCE(2021)
摘要
Fine-scale simulation of complex systems governed by multiscale partial differential equations (PDEs) is computationally expensive and various multiscale methods have been developed for addressing such problems. In addition, it is challenging to develop accurate surrogate and uncertainty quantification models for high-dimensional problems governed by stochastic multiscale PDEs using limited training data. In this work to address these challenges, we introduce a novel hybrid deep-learning and multiscale approach for stochastic multiscale PDEs with limited training data. For demonstration purposes, we focus on a porous media flow problem. We use an image-to-image supervised deep learning model to learn the mapping between the input permeability field and the multiscale basis functions. We introduce a Bayesian approach to this hybrid framework to allow us to perform uncertainty quantification and propagation tasks. The performance of this hybrid approach is evaluated with varying intrinsic dimensionality of the permeability field. Numerical results indicate that the hybrid network can efficiently predict well for high-dimensional inputs.
更多查看译文
关键词
Deep Learning, Neural Networks, Bayesian, Uncertainty Quantification, Multiscale, High-Dimensionality
AI 理解论文
溯源树
样例
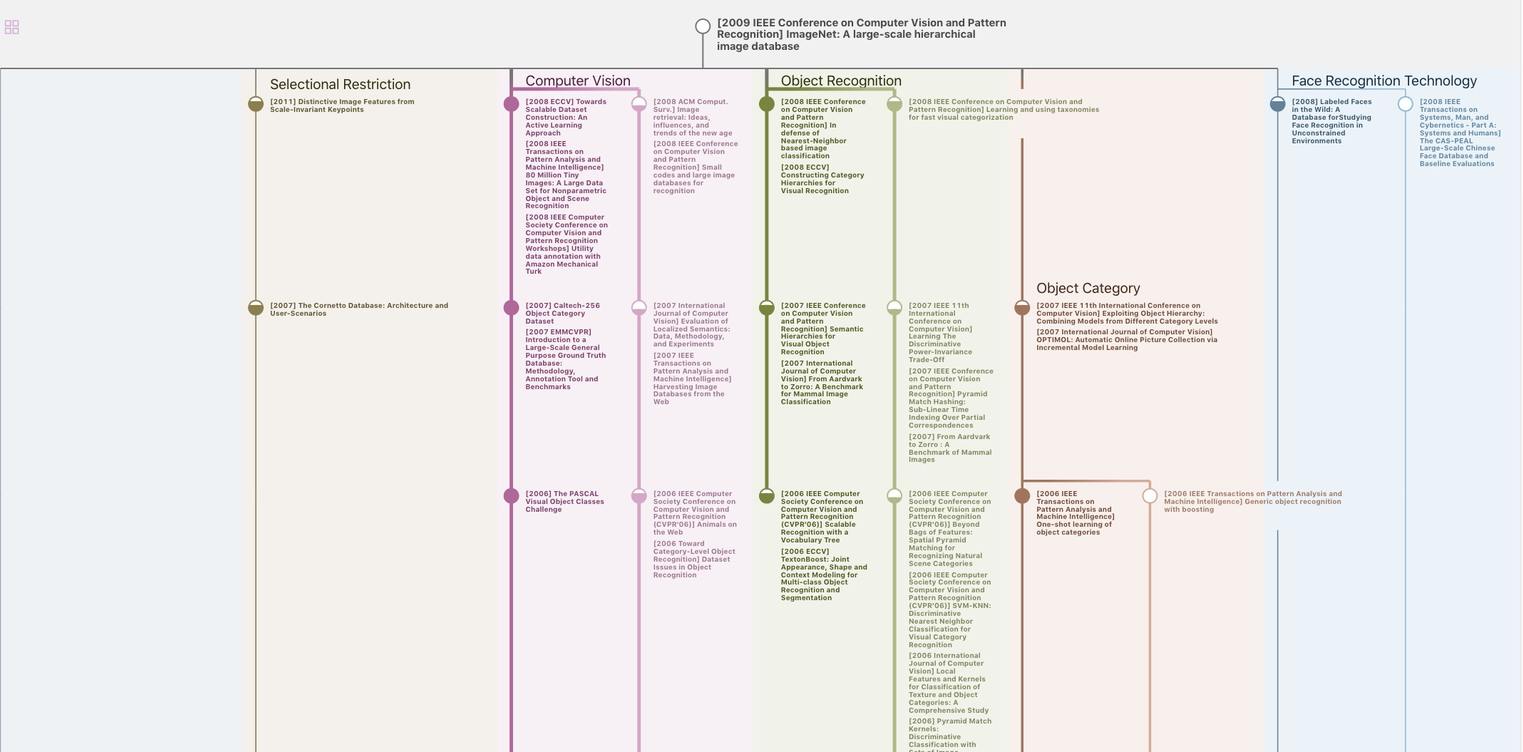
生成溯源树,研究论文发展脉络
Chat Paper
正在生成论文摘要