Feature Pooling of Modulation Spectrum Features for Improved Speech Emotion Recognition in the Wild
IEEE Transactions on Affective Computing(2021)
摘要
Interest in affective computing is burgeoning, in great part due to its role in emerging affective human-computer interfaces (HCI). To date, the majority of existing research on automated emotion analysis has relied on data collected in controlled environments. With the rise of HCI applications on mobile devices, however, so-called “in-the-wild” settings have posed a serious threat for emotion recognition systems, particularly those based on voice. In this case, environmental factors such as ambient noise and reverberation severely hamper system performance. In this paper, we quantify the detrimental effects that the environment has on emotion recognition and explore the benefits achievable with speech enhancement. Moreover, we propose a modulation spectral feature pooling scheme that is shown to outperform a state-of-the-art benchmark system for environment-robust prediction of spontaneous arousal and valence emotional primitives. Experiments on an environment-corrupted version of the RECOLA dataset of spontaneous interactions show the proposed feature pooling scheme, combined with speech enhancement, outperforming the benchmark across different noise-only, reverberation-only and noise-plus-reverberation conditions. Additional tests with the SEWA database show the benefits of the proposed method for in-the-wild applications.
更多查看译文
关键词
Affective computing,speech emotion recognition,modulation spectrum,in-the-wild
AI 理解论文
溯源树
样例
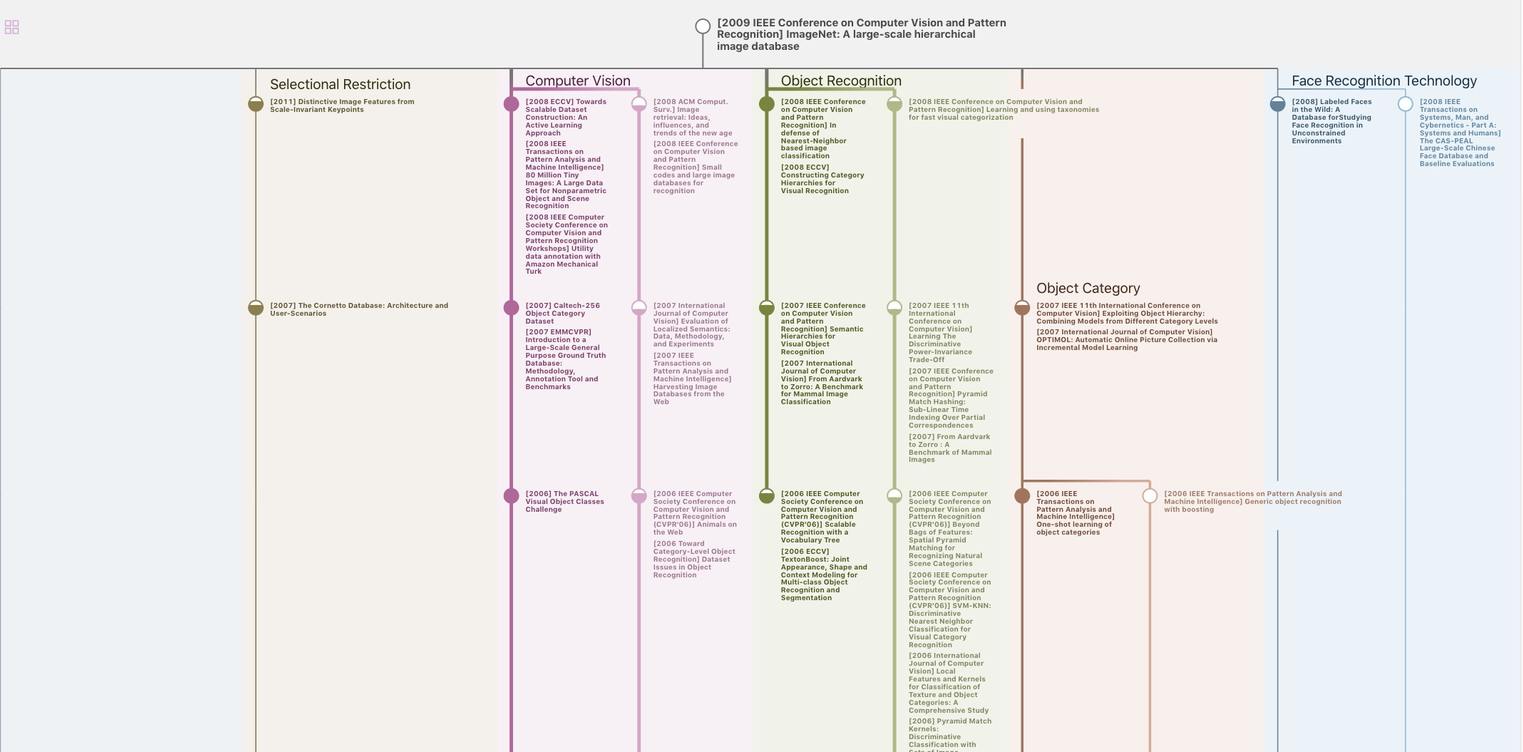
生成溯源树,研究论文发展脉络
Chat Paper
正在生成论文摘要