Leveraging Affective Hashtags for Ranking Music Recommendations
IEEE Transactions on Affective Computing(2021)
摘要
Mood and emotion play an important role when it comes to choosing musical tracks to listen to. In the field of music information retrieval and recommendation, emotion is considered contextual information that is hard to capture, albeit highly influential. In this study, we analyze the connection between users` emotional states and their musical choices. Particularly, we perform a large-scale study based on two data sets containing 560,000 and 90,000 #nowplaying tweets, respectively. We extract affective contextual information from hashtags contained in these tweets by applying an unsupervised sentiment dictionary approach. Subsequently, we utilize a state-of-the-art network embedding method to learn latent feature representations of users, tracks and hashtags. Based on both the affective information and the latent features, a set of eight ranking methods is proposed. We find that relying on a ranking approach that incorporates the latent representations of users and tracks allows for capturing a user's general musical preferences well (regardless of used hashtags or affective information). However, for capturing context-specific preferences (a more complex and personal ranking task), we find that ranking strategies that rely on affective information and that leverage hashtags as context information outperform the other ranking strategies.
更多查看译文
关键词
Emotion in music,emotion regulation,sentiment detection,ranking,music recommendation,microblogging,hashtags
AI 理解论文
溯源树
样例
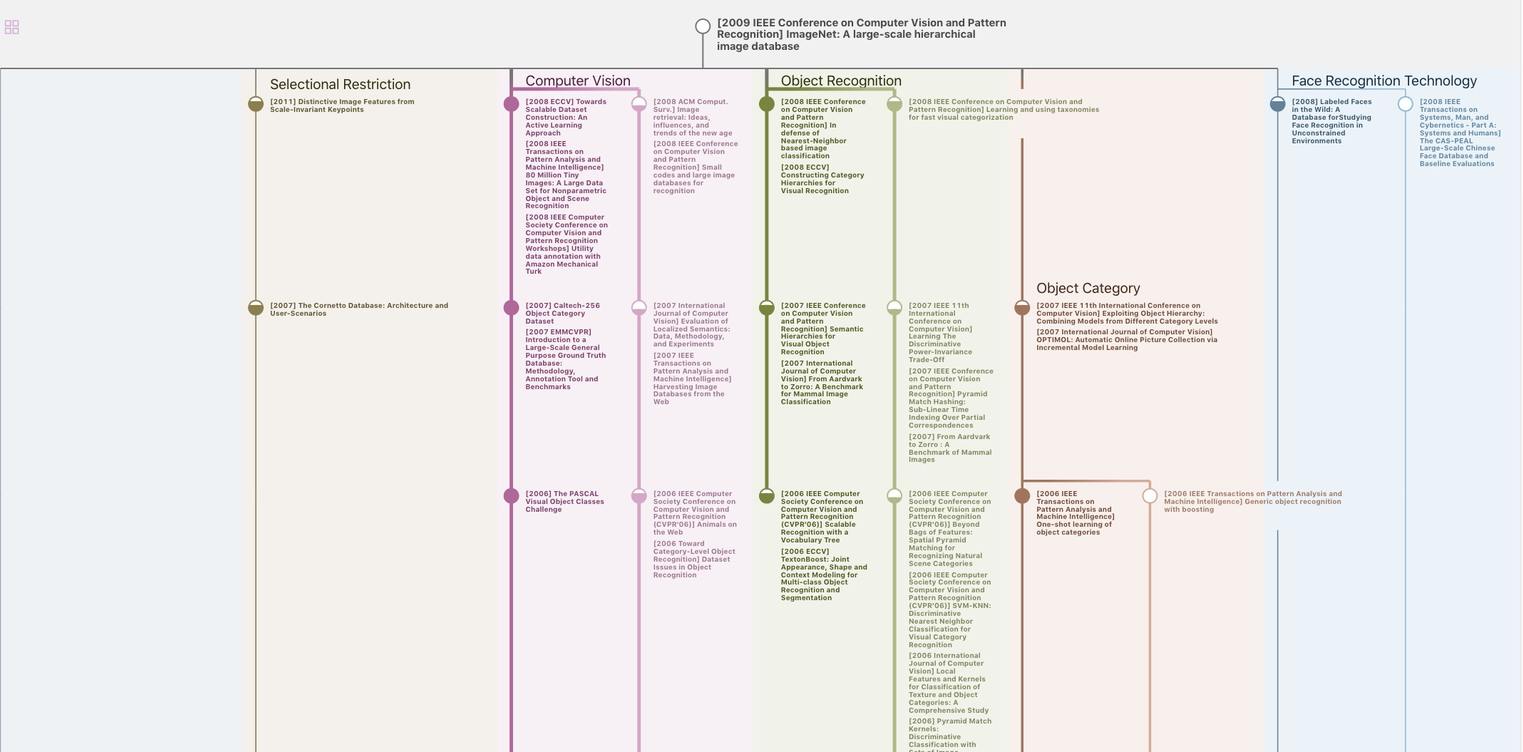
生成溯源树,研究论文发展脉络
Chat Paper
正在生成论文摘要