Box-To-Box Transformations For Modeling Joint Hierarchies
REPL4NLP 2021: PROCEEDINGS OF THE 6TH WORKSHOP ON REPRESENTATION LEARNING FOR NLP(2021)
摘要
Learning representations of entities and relations in structured knowledge bases is an active area of research, with much emphasis placed on choosing the appropriate geometry to capture the hierarchical structures exploited in, for example, ISA or HASPART relations. Box embeddings (Vilnis et al., 2018; Li et al., 2019; Dasgupta et al., 2020), which represent concepts as n-dimensional hyperrectangles, are capable of embedding hierarchies when training on a subset of the transitive closure. In Patel et al. (2020), the authors demonstrate that only the transitive reduction is required and further extend box embeddings to capture joint hierarchies by augmenting the graph with new nodes. While it is possible to represent joint hierarchies with this method, the parameters for each hierarchy are decoupled, making generalization between hierarchies infeasible. In this work, we introduce a learned box-to-box transformation that respects the structure of each hierarchy. We demonstrate that this not only improves the capability of modeling cross-hierarchy compositional edges but is also capable of generalizing from a subset of the transitive reduction.
更多查看译文
关键词
Transitive reduction,Transitive closure,Embedding,Hierarchy,Statistical relational learning,Feature learning,Generalization,Theoretical computer science,Computer science,Graph
AI 理解论文
溯源树
样例
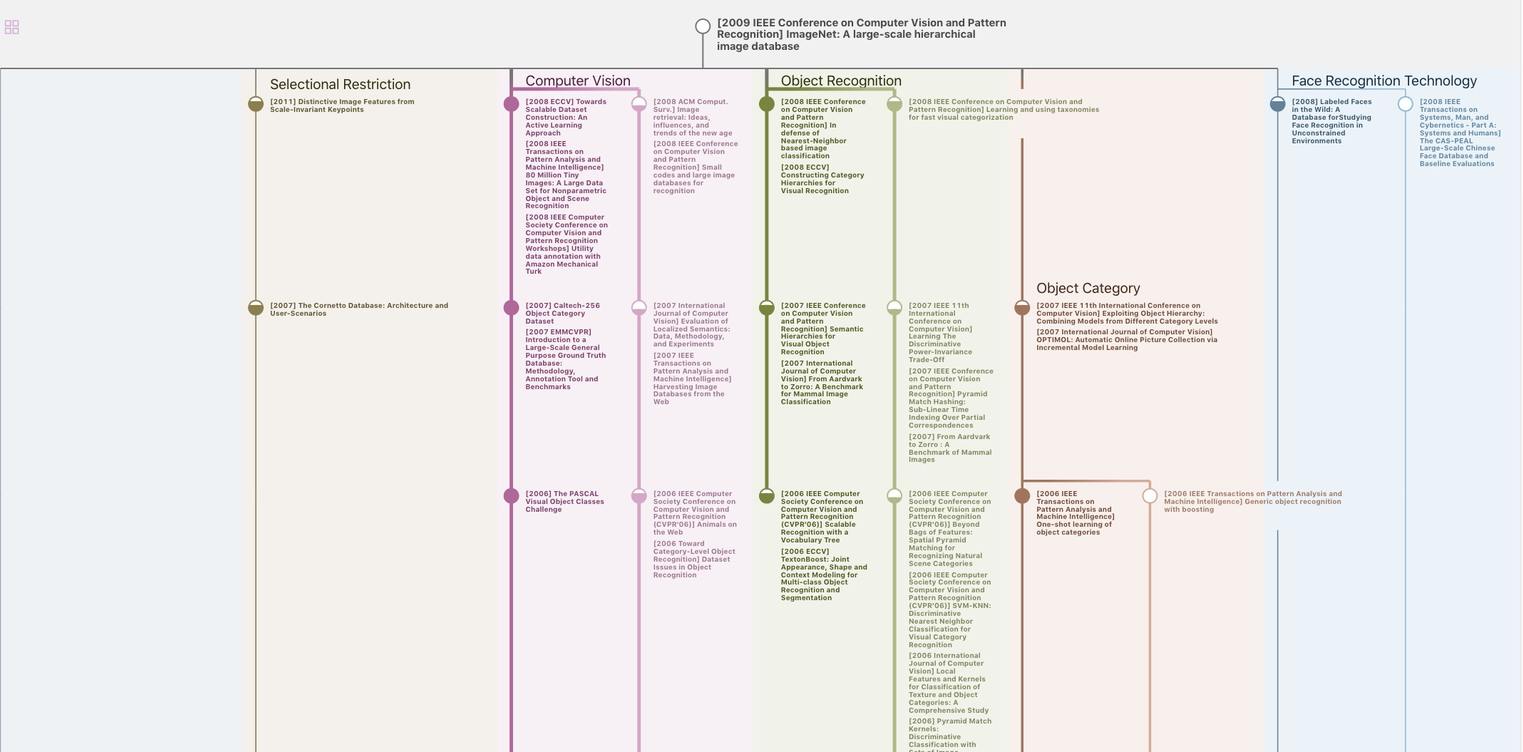
生成溯源树,研究论文发展脉络
Chat Paper
正在生成论文摘要