Data To Controller For Nonlinear Systems: An Approximate Solution
IEEE CONTROL SYSTEMS LETTERS(2022)
摘要
This letter considers the problem of determining an optimal control action based on observed data. We formulate the problem assuming that the system can be modeled by a nonlinear state-space model, but where the model parameters, state and future disturbances are not known and are treated as random variables. Central to our formulation is that the joint distribution of these unknown objects is conditioned on the observed data. Crucially, as new measurements become available, this joint distribution continues to evolve so that control decisions are made accounting for uncertainty as evidenced in the data. The resulting problem is intractable which we obviate by providing approximations that result in finite dimensional deterministic optimization problems. The proposed approach is demonstrated in simulation on a nonlinear system.
更多查看译文
关键词
Optimization, Monte Carlo methods, Markov processes, Uncertainty, Random variables, Measurement uncertainty, Data models, Identification for control, nonlinear systems identification, predictive control for nonlinear systems, stochastic optimal control
AI 理解论文
溯源树
样例
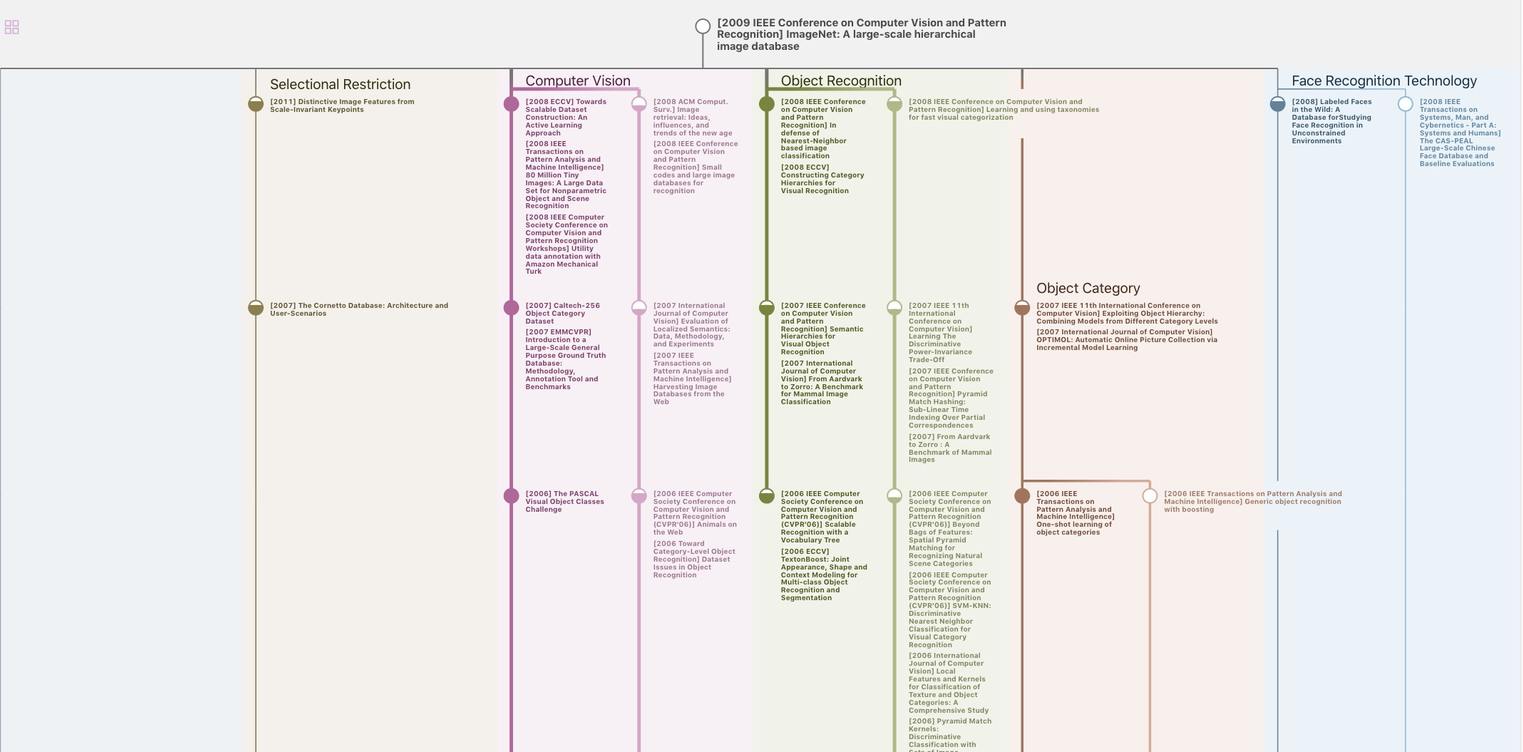
生成溯源树,研究论文发展脉络
Chat Paper
正在生成论文摘要