Discrete Predictive Representation for Long-horizon Planning
user-5f8cf9244c775ec6fa691c99(2021)
摘要
Discrete representations have been key in enabling robots to plan at more abstract levels and solve temporally-extended tasks more efficiently for decades. However, they typically require expert specifications. On the other hand, deep reinforcement learning aims to learn to solve tasks end-to-end, but struggles with long-horizon tasks. In this work, we propose Discrete Object-factorized Representation Planning (DORP), which learns temporally-abstracted discrete representations from exploratory video data in an unsupervised fashion via a mutual information maximization objective. DORP plans a sequence of abstract states for a low-level model-predictive controller to follow. In our experiments, we show that DORP robustly solves unseen long-horizon tasks. Interestingly, it discovers independent representations per object and binary properties such as a key-and-door.
更多查看译文
关键词
Reinforcement learning,Maximization,Mutual information,Artificial intelligence,Robot,Control theory,Computer science,Binary number,Horizon,Discrete representation
AI 理解论文
溯源树
样例
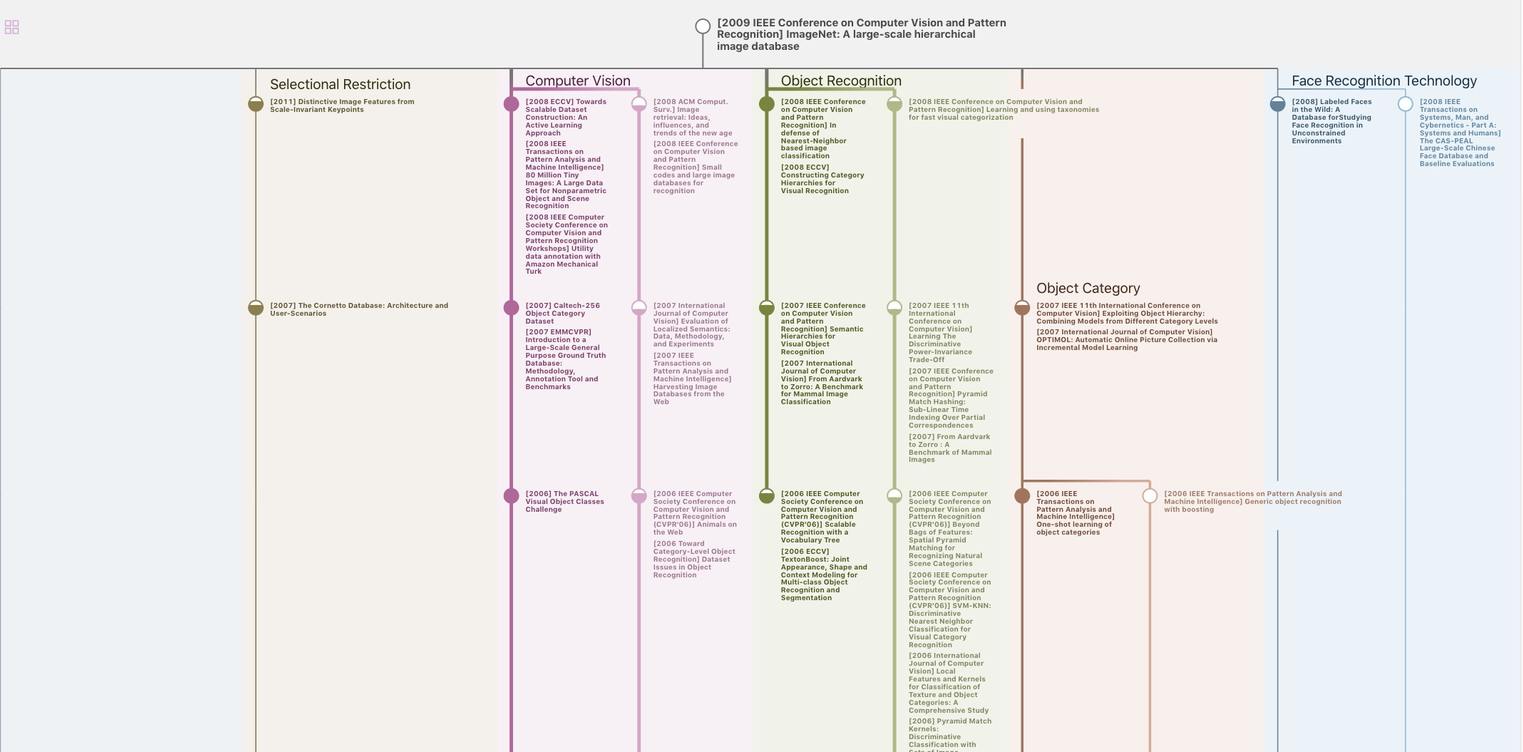
生成溯源树,研究论文发展脉络
Chat Paper
正在生成论文摘要