Learning Dynamics and Heterogeneity of Spatial-Temporal Graph Data for Traffic Forecasting
IEEE Transactions on Knowledge and Data Engineering(2022)
摘要
Accurate traffic forecasting is critical in improving safety, stability, and efficiency of intelligent transportation systems. Despite years of studies, accurate traffic prediction still faces the following challenges, including modeling the dynamics of traffic data along both temporal and spatial dimensions, and capturing the periodicity and the spatial heterogeneity of traffic data, and the problem is more difficult for long-term forecast. In this paper, we propose an Attention based Spatial-Temporal Graph Neural Network (ASTGNN) for traffic forecasting. Specifically, in the temporal dimension, we design a novel self-attention mechanism that is capable of utilizing the local context, which is specialized for numerical sequence representation transformation. It enables our prediction model to capture the temporal dynamics of traffic data and to enjoy global receptive fields that is beneficial for long-term forecast. In the spatial dimension, we develop a dynamic graph convolution module, employing self-attention to capture the spatial correlations in a dynamic manner. Furthermore, we explicitly model the periodicity and capture the spatial heterogeneity through embedding modules. Experiments on five real-world traffic flow datasets demonstrate that ASTGNN outperforms the state-of-the-art baselines.
更多查看译文
关键词
Traffic forecasting,spatial-temporal graph data,self-attention,graph convolution
AI 理解论文
溯源树
样例
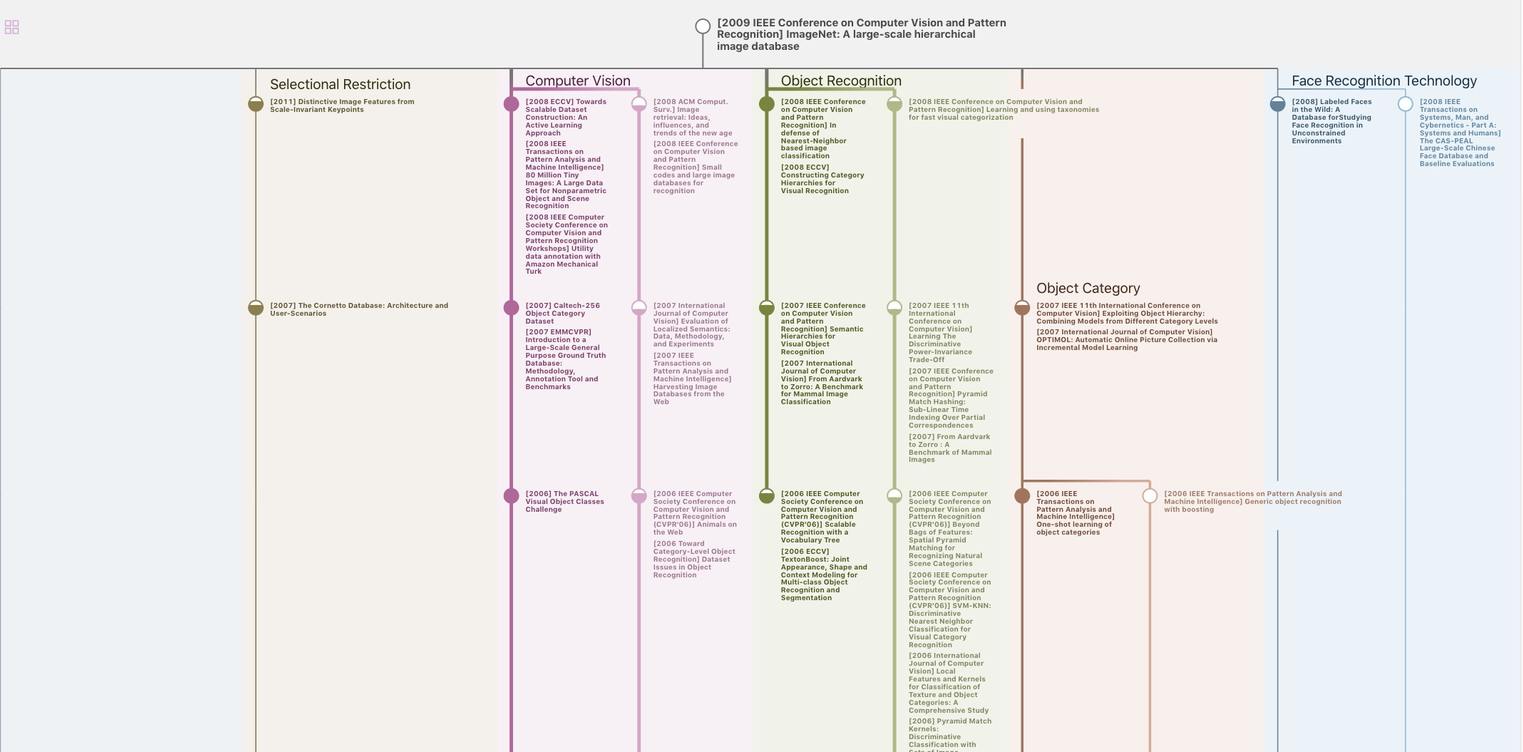
生成溯源树,研究论文发展脉络
Chat Paper
正在生成论文摘要