Radio Frequency Fingerprinting on the Edge
IEEE Transactions on Mobile Computing(2022)
摘要
Deep learning methods have been very successful at radio frequency fingerprinting tasks, predicting the identity of transmitting devices with high accuracy. We study radio frequency fingerprinting deployments at resource-constrained edge devices. We use structured pruning to jointly train and sparsify neural networks tailored to edge hardware implementations. We compress convolutional layers by a
$27.2\times$
factor while incurring a negligible prediction accuracy decrease (less than 1 percent). We demonstrate the efficacy of our approach over multiple edge hardware platforms, including a Samsung Gallaxy S10 phone and a Xilinx-ZCU104 FPGA. Our method yields significant inference speedups,
$11.5\times$
on the FPGA and
$3\times$
on the smartphone, as well as high efficiency: the FPGA processing time is
$17\times$
smaller than in a V100 GPU. To the best of our knowledge, we are the first to explore the possibility of compressing networks for radio frequency fingerprinting; as such, our experiments can be seen as a means of characterizing the informational capacity associated with this specific learning task.
更多查看译文
关键词
Radio frequency fingerprinting,edge computing,model pruning
AI 理解论文
溯源树
样例
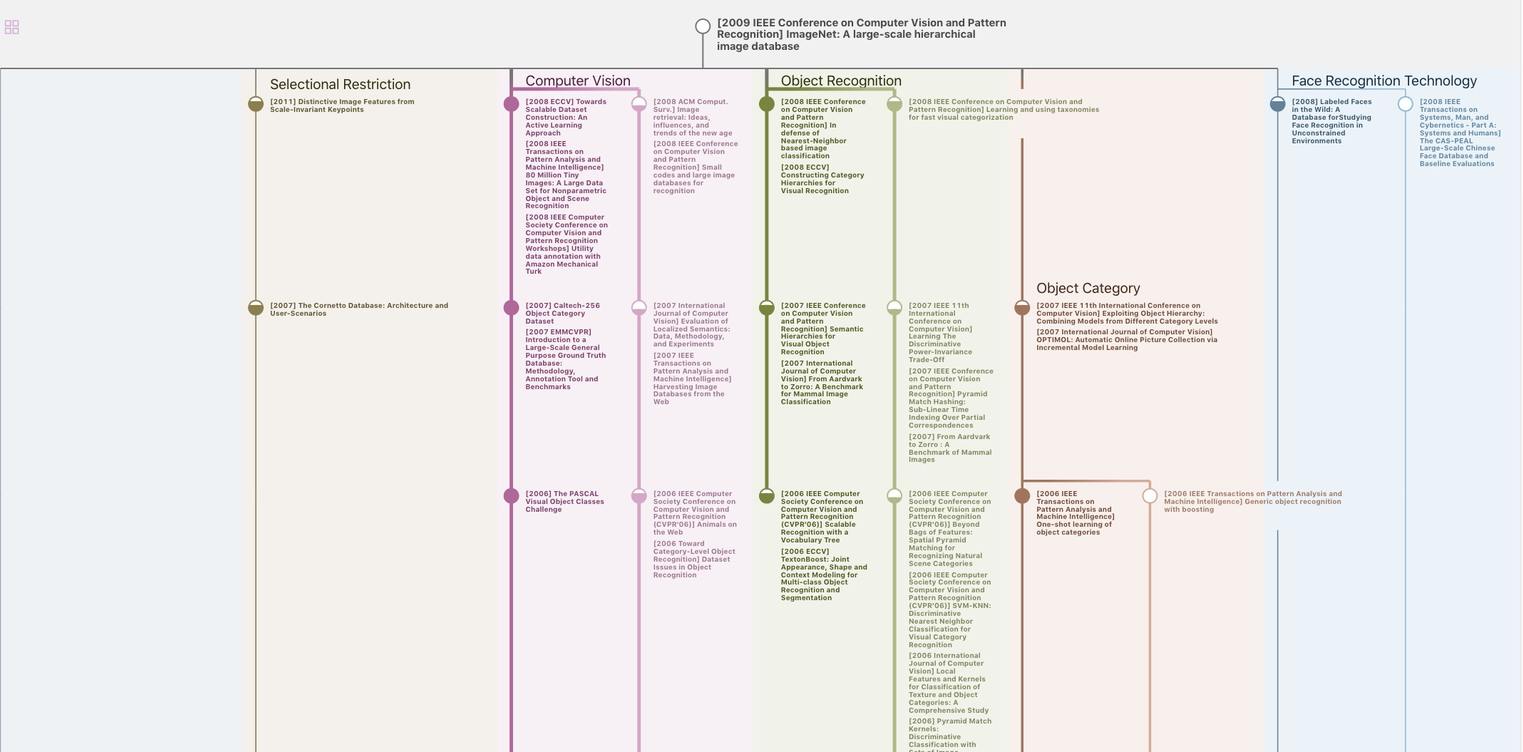
生成溯源树,研究论文发展脉络
Chat Paper
正在生成论文摘要