Hyperparameter Optimization Across Problem Tasks
user-5ed732bc4c775e09d87b4c18(2018)
摘要
Hyperparameter Optimization is a task that is generally hard to accomplish as the correct setting of hyperparameters cannot be learned from the data directly. However, finding the right hyperparameters is necessary as the performance on test data can differ a lot under various hyperparameter settings. Many researchers rely on search techniques such as grid-search, having the downside that they require a lot of computation time, as prediction models are learned for a wide range of possible hyperparameter configurations which is only feasible in a parallel computing environment. Recently, search methods based on Bayesian optimization such as SMAC have been proposed and extended to include hyperparameter performance of the same model on another data set. These meta learning approaches show that the search for well-performing hyperparameters can be steered in a more intelligent manner. In this work, we aim to accomplish hyperparameter optimization across problem tasks where we specifically target regression and classification problems. We show, that the incorporation of hyperparameter performance on a classification task is helpful when optimizing hyperparameters for a regression task and vice versa.
更多查看译文
关键词
Hyperparameter optimization,Hyperparameter,Bayesian optimization,Test data,Machine learning,Computer science,Computation,Predictive modelling,Regression,Artificial intelligence
AI 理解论文
溯源树
样例
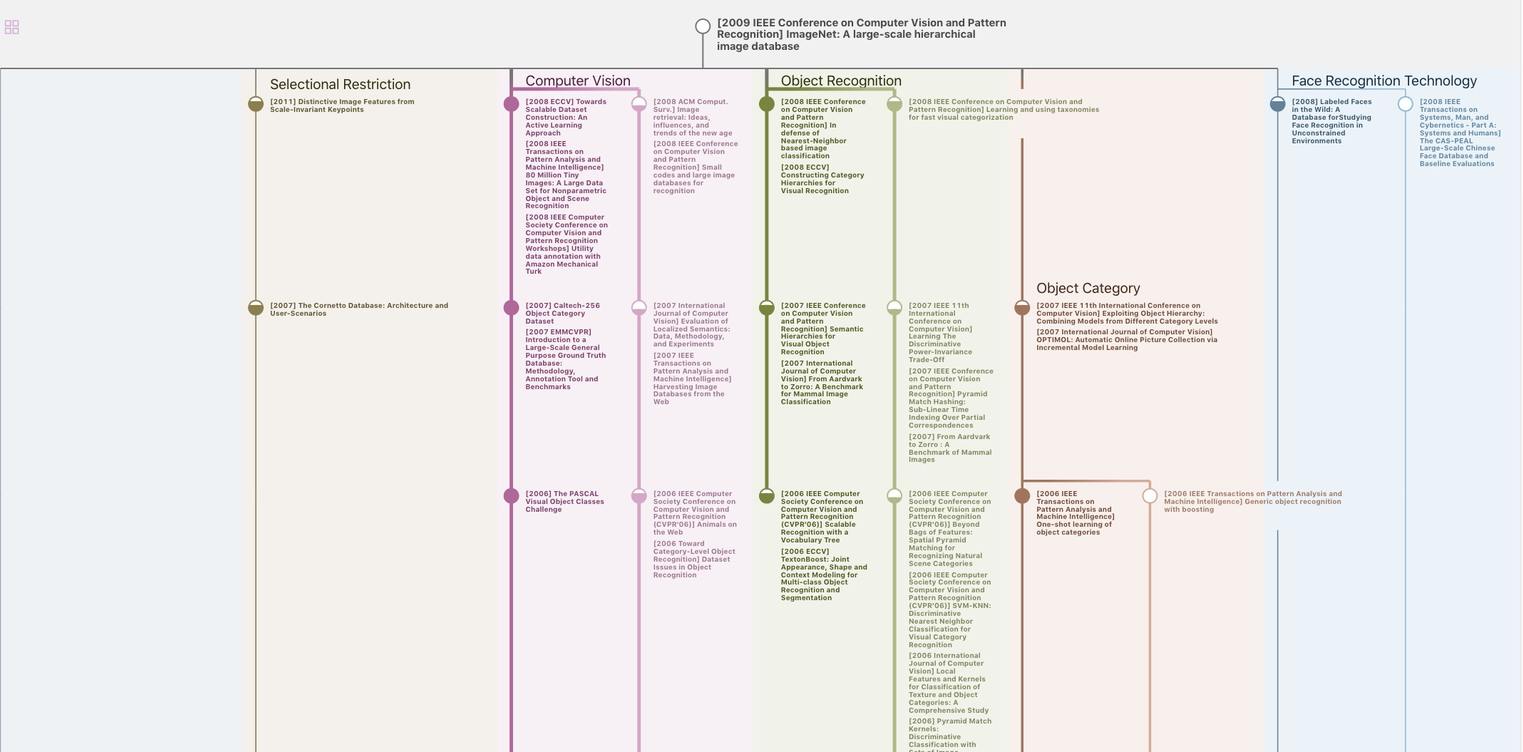
生成溯源树,研究论文发展脉络
Chat Paper
正在生成论文摘要