Progressive residual learning for single image dehazing
arxiv(2021)
摘要
The recent physical model-free dehazing methods have achieved state-of-the-art performances. However, without the guidance of physical models, the performances degrade rapidly when applied to real scenarios due to the unavailable or insufficient data problems. On the other hand, the physical model-based methods have better interpretability but suffer from multi-objective optimizations of parameters, which may lead to sub-optimal dehazing results. In this paper, a progressive residual learning strategy has been proposed to combine the physical model-free dehazing process with reformulated scattering model-based dehazing operations, which enjoys the merits of dehazing methods in both categories. Specifically, the global atmosphere light and transmission maps are interactively optimized with the aid of accurate residual information and preliminary dehazed restorations from the initial physical model-free dehazing process. The proposed method performs favorably against the state-of-the-art methods on public dehazing benchmarks with better model interpretability and adaptivity for complex hazy data.
更多查看译文
关键词
single image dehazing,progressive residual learning
AI 理解论文
溯源树
样例
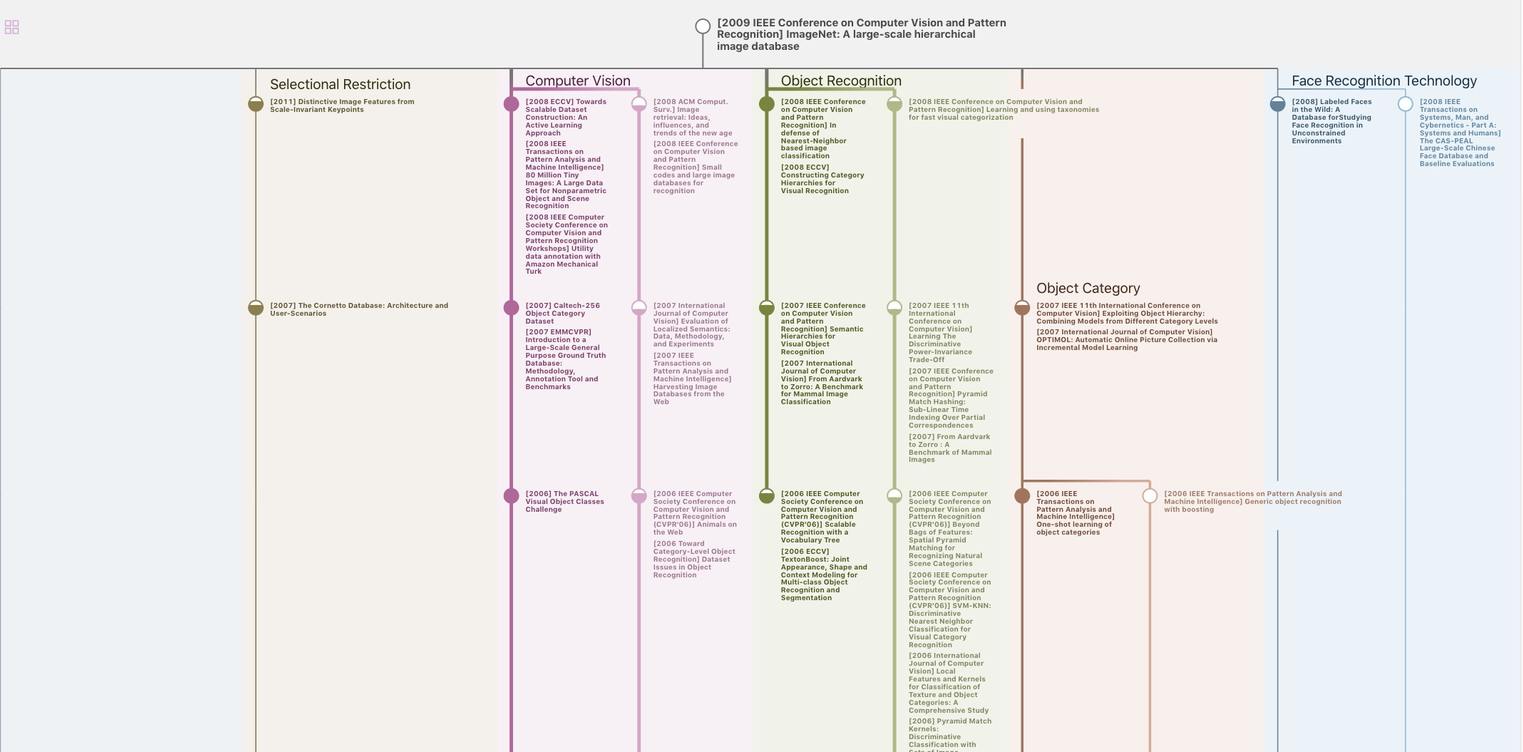
生成溯源树,研究论文发展脉络
Chat Paper
正在生成论文摘要