DivCo: Diverse Conditional Image Synthesis via Contrastive Generative Adversarial Network
2021 IEEE/CVF CONFERENCE ON COMPUTER VISION AND PATTERN RECOGNITION, CVPR 2021(2021)
摘要
Conditional generative adversarial networks (cGANs) target at synthesizing diverse images given the input conditions and latent codes, but unfortunately, they usually suffer from the issue of mode collapse. To solve this issue, previous works [47, 22] mainly focused on encouraging the correlation between the latent codes and their generated images, while ignoring the relations between images generated from various latent codes. The recent MSGAN [27] tried to encourage the diversity of the generated image but only considers "negative" relations between the image pairs. In this paper, we propose a novel DivCo framework to properly constrain both "positive" and "negative" relations between the generated images specified in the latent space. To the best of our knowledge, this is the first attempt to use contrastive learning for diverse conditional image synthesis. A novel latent-augmented contrastive loss is introduced, which encourages images generated from adjacent latent codes to be similar and those generated from distinct latent codes to be dissimilar. The proposed latent-augmented contrastive loss is well compatible with various cGAN architectures. Extensive experiments demonstrate that the proposed DivCo can produce more diverse images than state-of-the-art methods without sacrificing visual quality in multiple unpaired and paired image generation tasks. Training code and pretrained models are available at https://github.com/ruiliu-ai/DivCo.
更多查看译文
关键词
DivCo,diverse conditional image synthesis,contrastive generative adversarial network,conditional generative adversarial networks target,synthesizing diverse images,input conditions,negative relations,image pairs,latent space,novel latent-augmented contrastive loss,adjacent latent codes,distinct latent codes,multiple unpaired paired image generation tasks
AI 理解论文
溯源树
样例
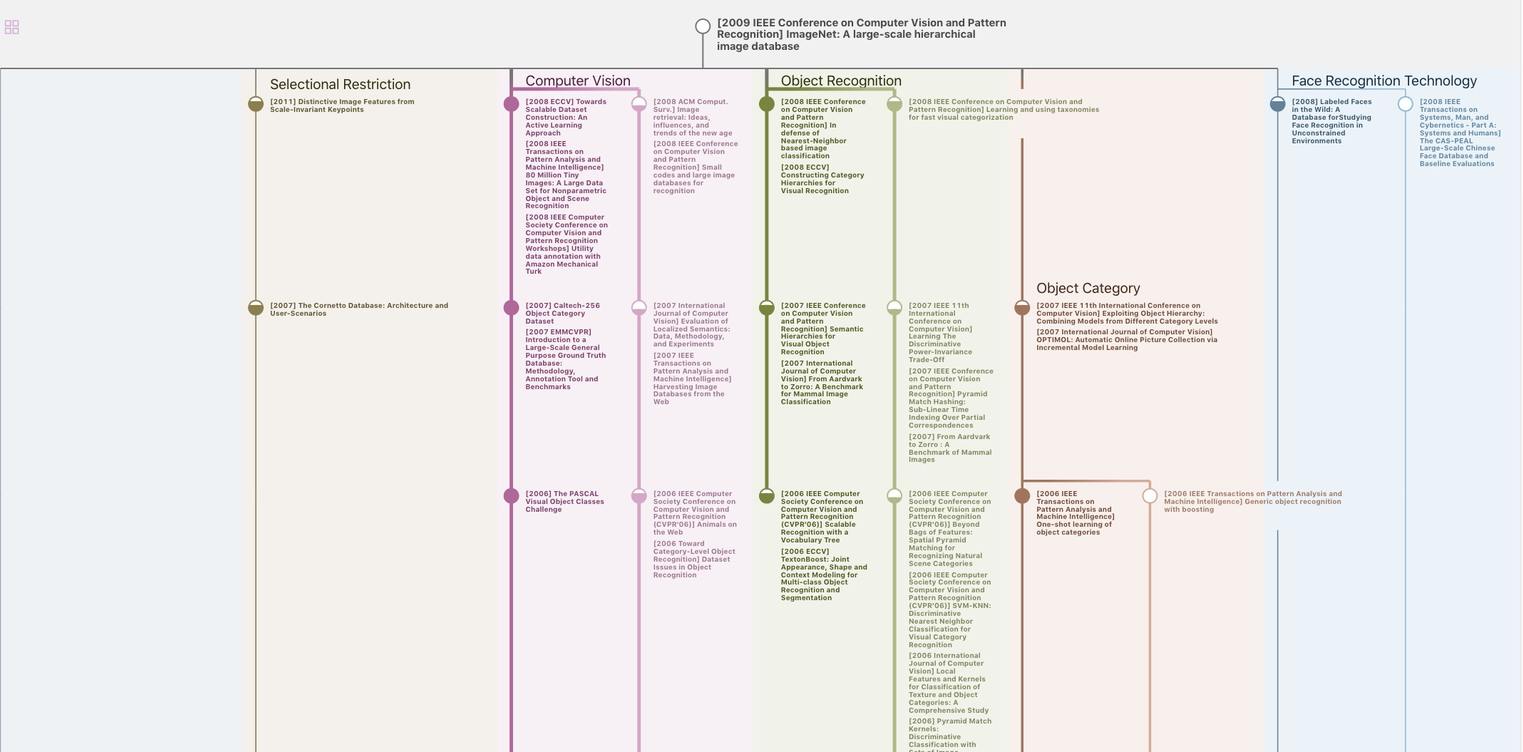
生成溯源树,研究论文发展脉络
Chat Paper
正在生成论文摘要