Reconsidering Representation Alignment for Multi-view Clustering
2021 IEEE/CVF CONFERENCE ON COMPUTER VISION AND PATTERN RECOGNITION, CVPR 2021(2021)
摘要
Aligning distributions of view representations is a core component of today's state of the art models for deep multi-view clustering. However, we identify several drawbacks with naively aligning representation distributions. We demonstrate that these drawbacks both lead to less separable clusters in the representation space, and inhibit the model's ability to prioritize views. Based on these observations, we develop a simple baseline model for deep multi-view clustering. Our baseline model avoids representation alignment altogether, while performing similar to, or better than, the current state of the art. We also expand our baseline model by adding a contrastive learning component. This introduces a selective alignment procedure that preserves the model's ability to prioritize views. Our experiments show that the contrastive learning component enhances the baseline model, improving on the current state of the art by a large margin on several datasets(1).
更多查看译文
关键词
representation,multi-view
AI 理解论文
溯源树
样例
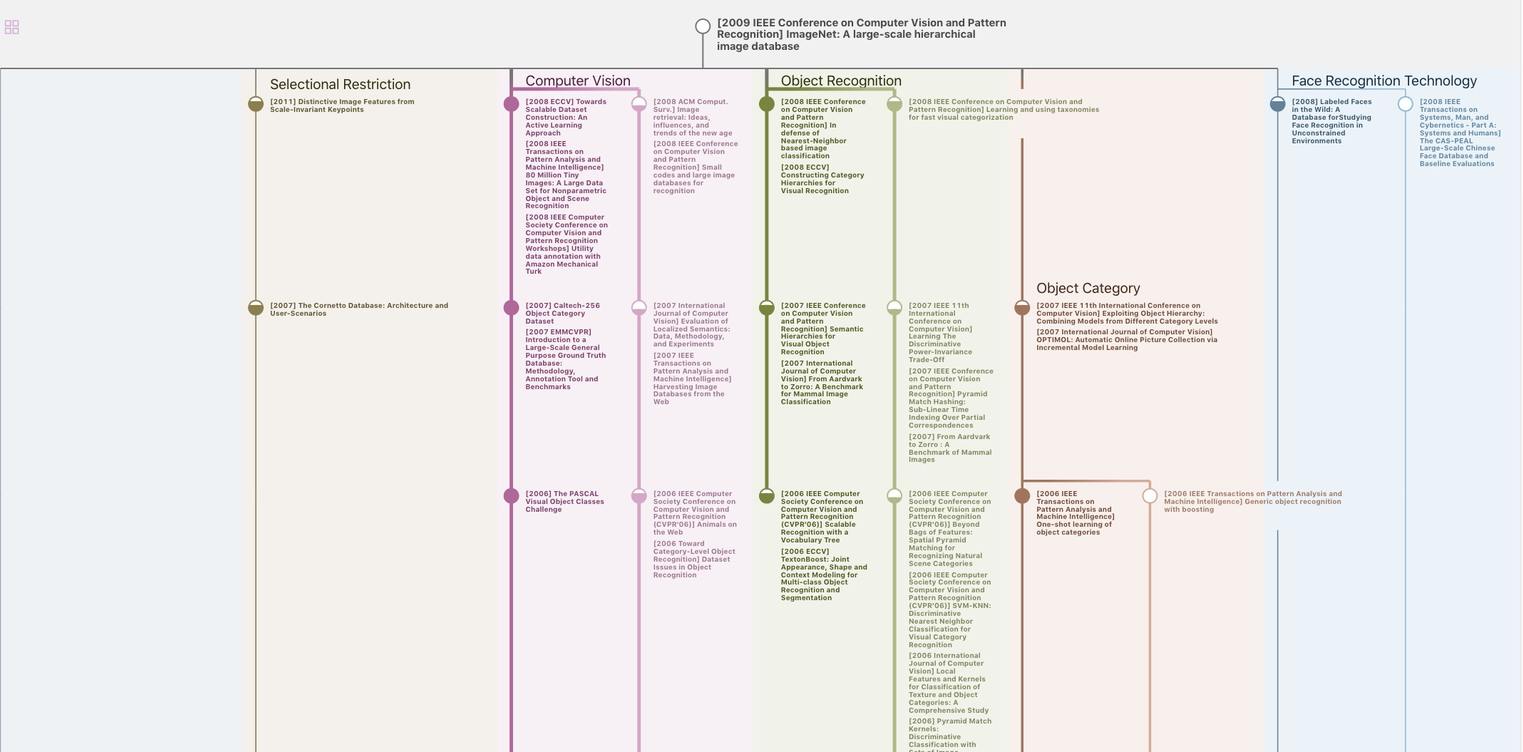
生成溯源树,研究论文发展脉络
Chat Paper
正在生成论文摘要