Neural Networks Trained on Natural Scenes Exhibit Gestalt Closure
Computational Brain & Behavior(2021)
摘要
The Gestalt laws of perceptual organization, which describe how visual elements in an image are grouped and interpreted, have traditionally been thought of as innate. Given past research showing that these laws have ecological validity, we investigate whether deep learning methods infer Gestalt laws from the statistics of natural scenes. We examine the law of closure , which asserts that human visual perception tends to “close the gap” by assembling elements that can jointly be interpreted as a complete figure or object. We demonstrate that a state-of-the-art convolutional neural network, trained to classify natural images, exhibits closure on synthetic displays of edge fragments, as assessed by similarity of internal representations. This finding provides further support for the hypothesis that the human perceptual system is even more elegant than the Gestaltists imagined: a single law—adaptation to the statistical structure of the environment—might suffice as fundamental.
更多查看译文
关键词
Gestalt laws, Closure, Deep learning, Natural scene statistics
AI 理解论文
溯源树
样例
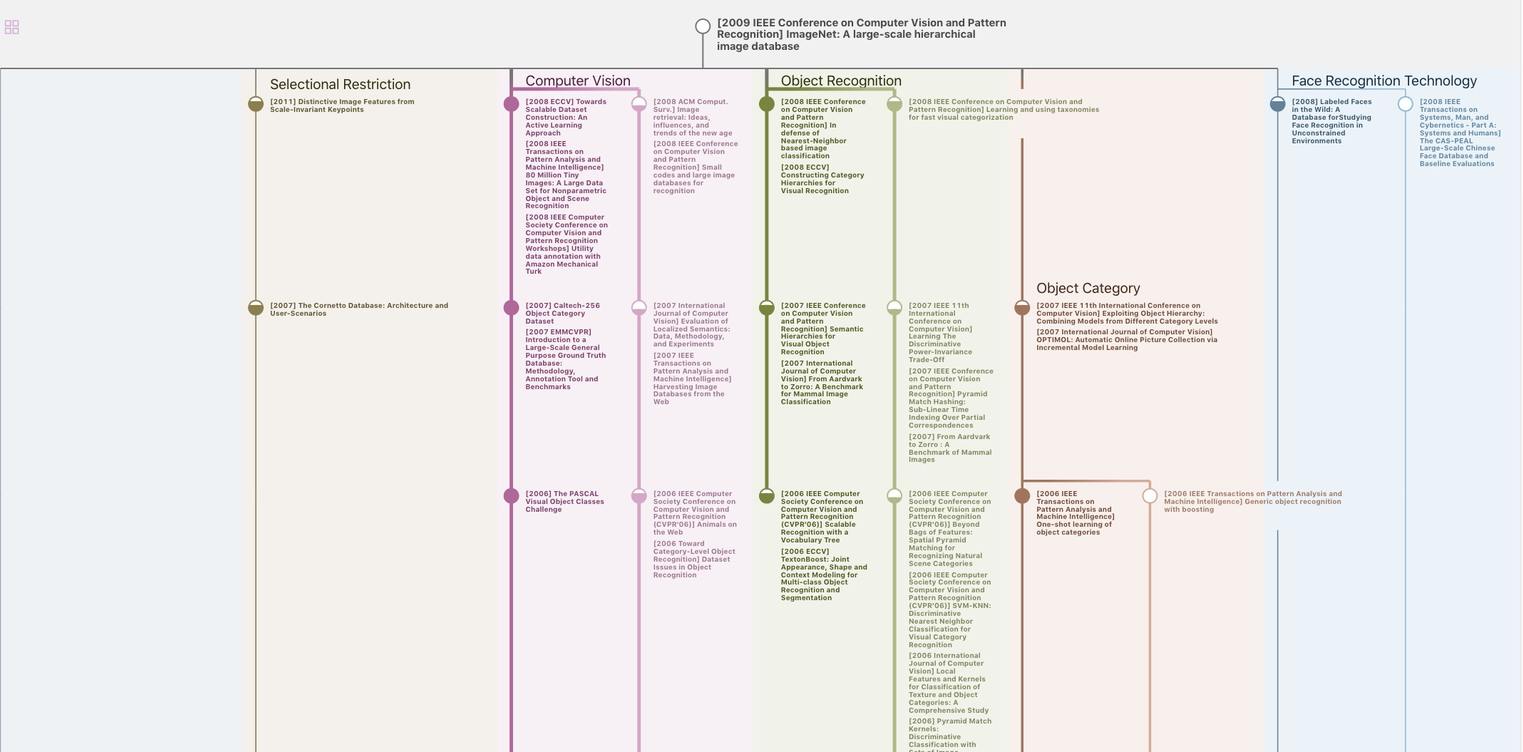
生成溯源树,研究论文发展脉络
Chat Paper
正在生成论文摘要