ForceNet: A Graph Neural Network for Large-Scale Quantum Chemistry Simulation
user-5d8054e8530c708f9920ccce(2021)
摘要
Machine Learning (ML) has a potential to dramatically accelerate large-scale physics-based simulations. However, practical models for real large-scale and complex problems remain out of reach. Here we present ForceNet, a model for accurate and fast quantum chemistry simulations to accelerate catalyst discovery for renewable energy applications. ForceNet is a graph neural network that uses surrounding 3D molecular structure to estimate per-atom forces---a central capability for performing atomic simulations. The key challenge is to accurately capture highly complex and non-linear quantum interactions of atoms in 3D space, on which forces are dependent. To this end, ForceNet adopts (1) expressive message passing architecture, (2) appropriate choice of basis and non-linear activation functions, and (3) model scaling in terms of network depth and width. We show ForceNet reduces the estimation error of atomic forces by 30% compared to existing ML models, and generalizes well to out-of-distribution structures. Finally, we apply ForceNet to the large-scale catalyst dataset, OC20. We use ForceNet to perform quantum chemistry simulations, where ForceNet is able to achieve 4x higher success rate than existing ML models. Overall, we demonstrate the potential for ML-based simulations to achieve practical usefulness while being orders of magnitude faster than physics-based simulations.
更多查看译文
关键词
Computational science,Scaling,Quantum,Orders of magnitude (numbers),Quantum chemistry,3d space,Complex problems,Graph neural networks,Message passing architecture
AI 理解论文
溯源树
样例
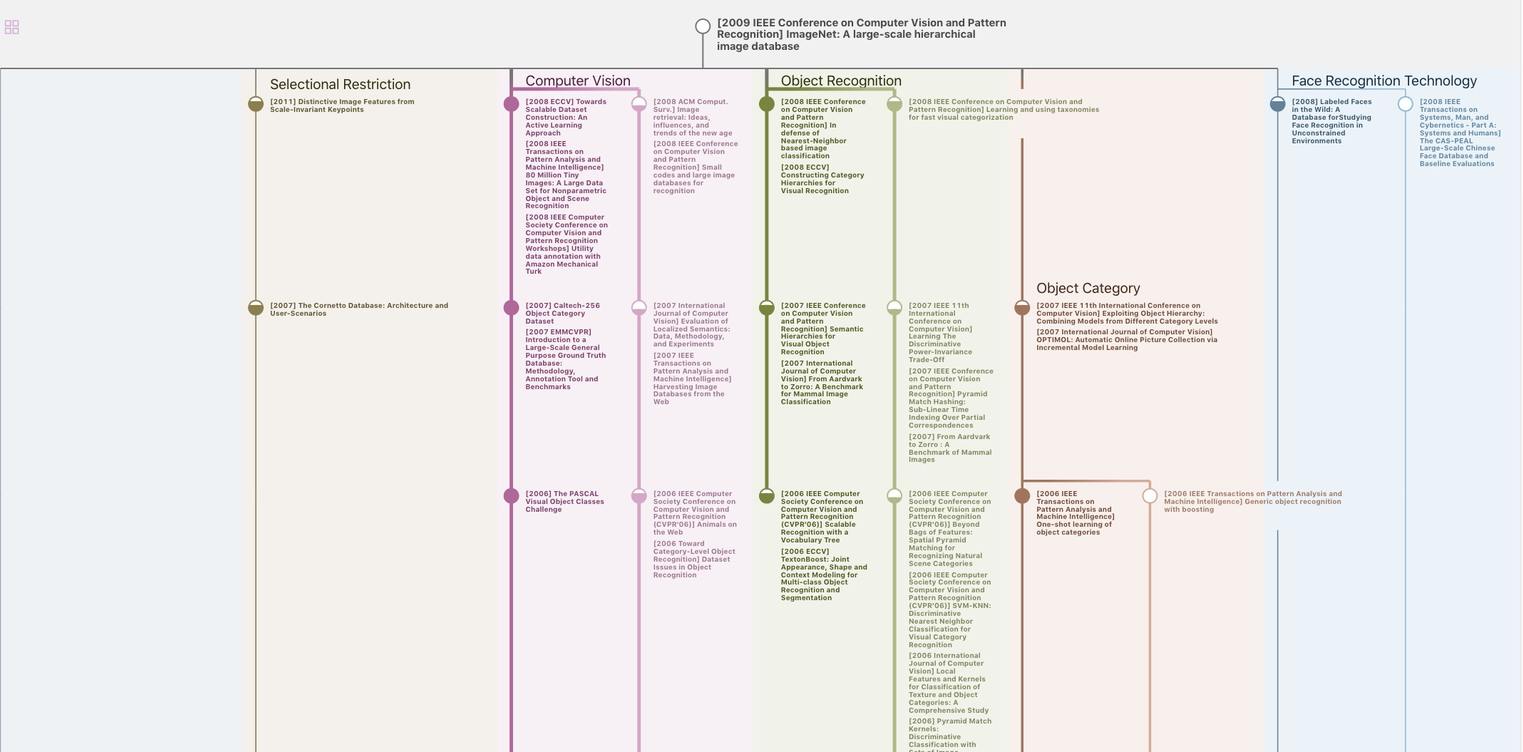
生成溯源树,研究论文发展脉络
Chat Paper
正在生成论文摘要