Exploiting Playbacks in Unsupervised Domain Adaptation for 3D Object Detection in Self-Driving Cars
user-5d8054e8530c708f9920ccce(2022)
摘要
Self-driving cars must detect other traffic participants like vehicles and pedestrians in 3D in order to plan safe routes and avoid collisions. State-of-the-art 3D object detectors, based on deep learning, have shown promising accuracy but are prone to over-fit domain idiosyncrasies, making them fail in new environments-a serious problem for the robustness of self-driving cars. In this paper, we propose a novel learning approach that reduces this gap by fine-tuning the detector on high-quality pseudo-labels in the target domain - pseudo-labels that are automatically generated after driving based on replays of previously recorded driving sequences. In these replays, object tracks are smoothed forward and backward in time, and detections are interpolated and extrapolated-crucially, leveraging future information to catch hard cases such as missed detections due to occlusions or far ranges. We show, across five autonomous driving datasets, that fine-tuning the object detector on these pseudo-labels substantially reduces the domain gap to new driving environments, yielding strong improvements detection reliability and accuracy.
更多查看译文
关键词
Object Detection,Segmentation and Categorization,Computer Vision for Automation,Transfer Learning,Deep Learning for Visual Perception
AI 理解论文
溯源树
样例
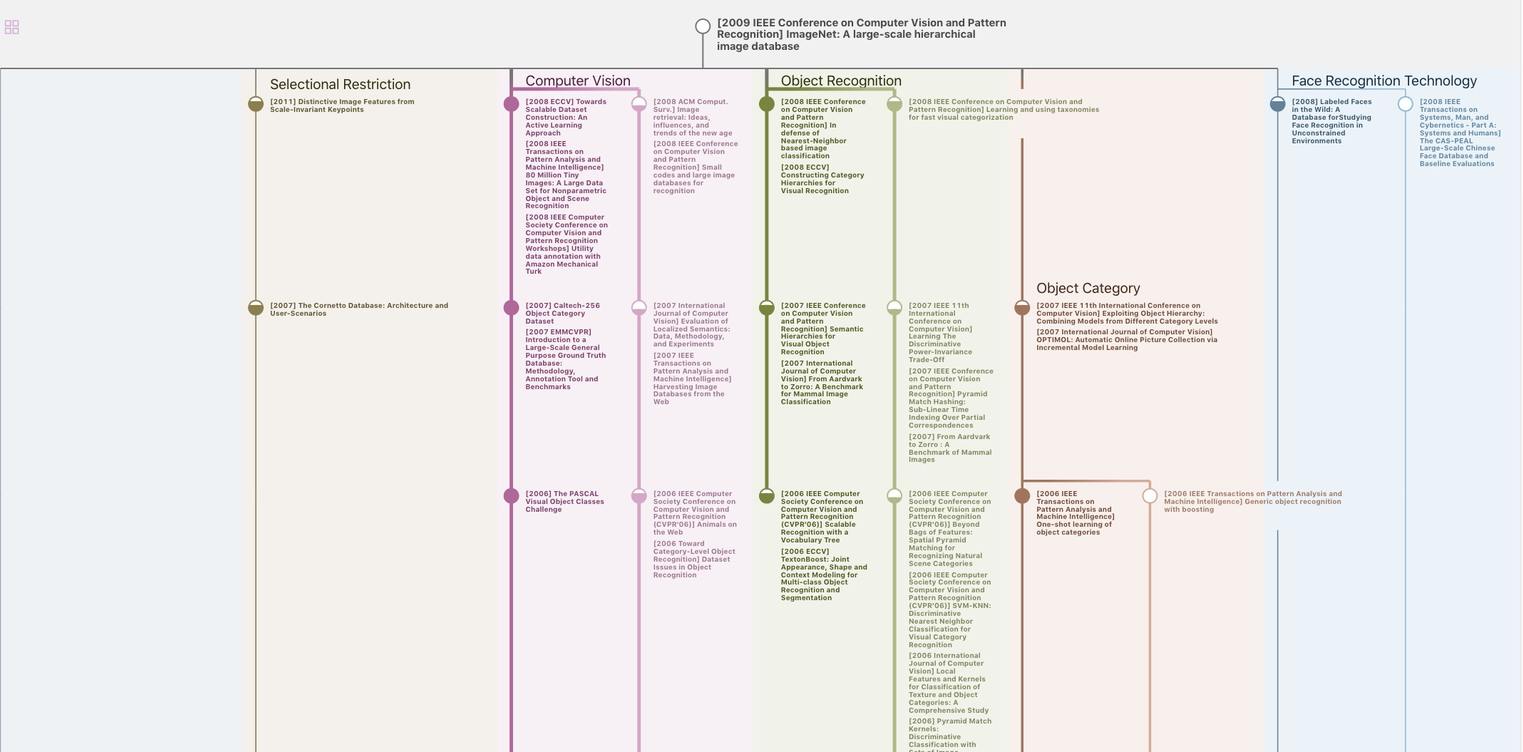
生成溯源树,研究论文发展脉络
Chat Paper
正在生成论文摘要