Discovering diverse solutions in deep reinforcement learning by maximizing state-action-based mutual information
NEURAL NETWORKS(2022)
摘要
Reinforcement learning algorithms are typically limited to learning a single solution for a specified task, even though diverse solutions often exist. Recent studies showed that learning a set of diverse solutions is beneficial because diversity enables robust few-shot adaptation. Although existing methods learn diverse solutions by using the mutual information as unsupervised rewards, such an approach often suffers from the bias of the gradient estimator induced by value function approximation. In this study, we propose a novel method that can learn diverse solutions without suffering the bias problem. In our method, a policy conditioned on a continuous or discrete latent variable is trained by directly maximizing the variational lower bound of the mutual information, instead of using the mutual information as unsupervised rewards as in previous studies. Through extensive experiments on robot locomotion tasks, we demonstrate that the proposed method successfully learns an infinite set of diverse solutions by learning continuous latent variables, which is more challenging than learning a finite number of solutions. Subsequently, we show that our method enables more effective few-shot adaptation compared with existing methods. (C) 2022 Elsevier Ltd. All rights reserved.
更多查看译文
关键词
Reinforcement learning,Robot learning,Representation learning
AI 理解论文
溯源树
样例
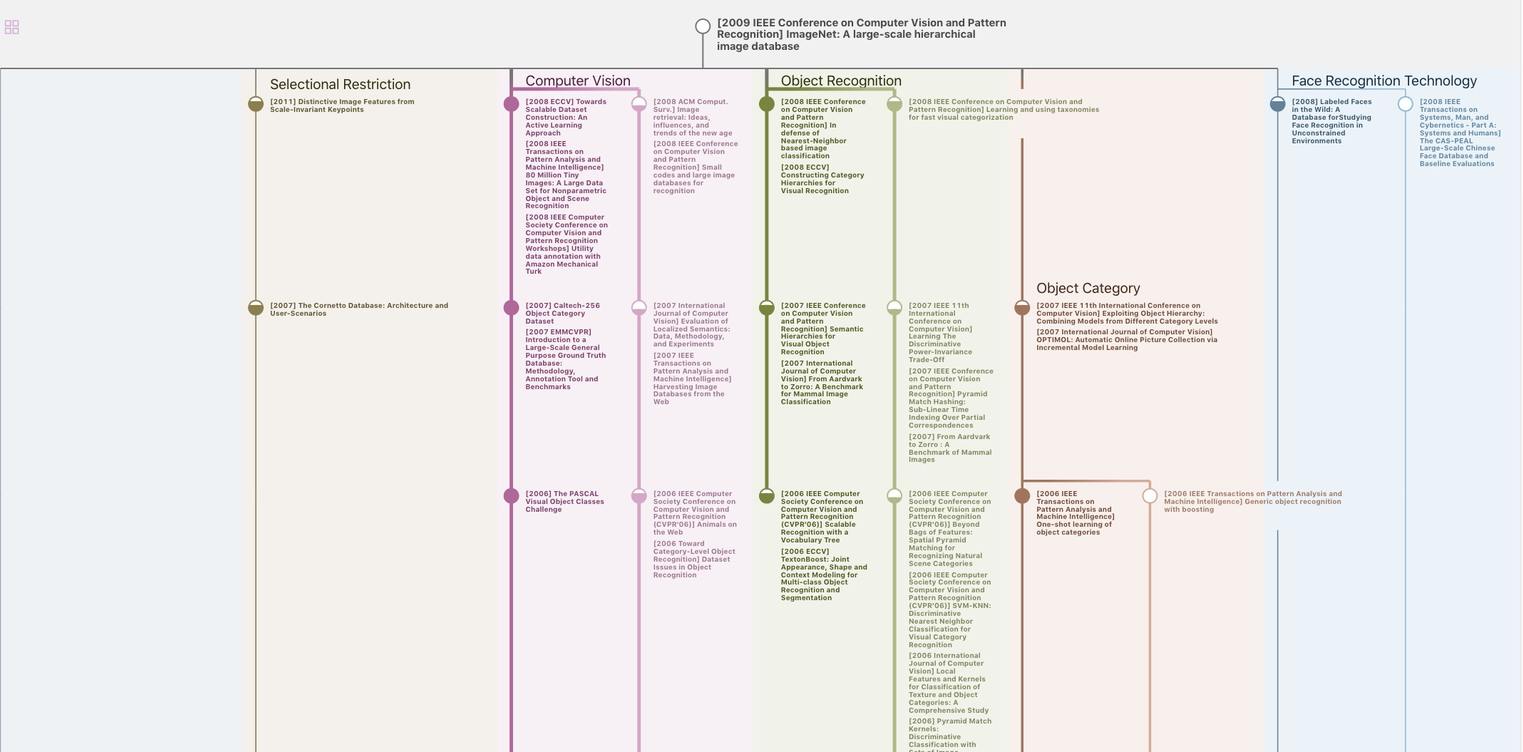
生成溯源树,研究论文发展脉络
Chat Paper
正在生成论文摘要