Vision Transformer for COVID-19 CXR Diagnosis using Chest X-ray Feature Corpus
arxiv(2021)
摘要
Under the global COVID-19 crisis, developing robust diagnosis algorithm for COVID-19 using CXR is hampered by the lack of the well-curated COVID-19 data set, although CXR data with other disease are abundant. This situation is suitable for vision transformer architecture that can exploit the abundant unlabeled data using pre-training. However, the direct use of existing vision transformer that uses the corpus generated by the ResNet is not optimal for correct feature embedding. To mitigate this problem, we propose a novel vision Transformer by using the low-level CXR feature corpus that are obtained to extract the abnormal CXR features. Specifically, the backbone network is trained using large public datasets to obtain the abnormal features in routine diagnosis such as consolidation, glass-grass opacity (GGO), etc. Then, the embedded features from the backbone network are used as corpus for vision transformer training. We examine our model on various external test datasets acquired from totally different institutions to assess the generalization ability. Our experiments demonstrate that our method achieved the state-of-art performance and has better generalization capability, which are crucial for a widespread deployment.
更多查看译文
关键词
vision transformer,diagnosis,x-ray
AI 理解论文
溯源树
样例
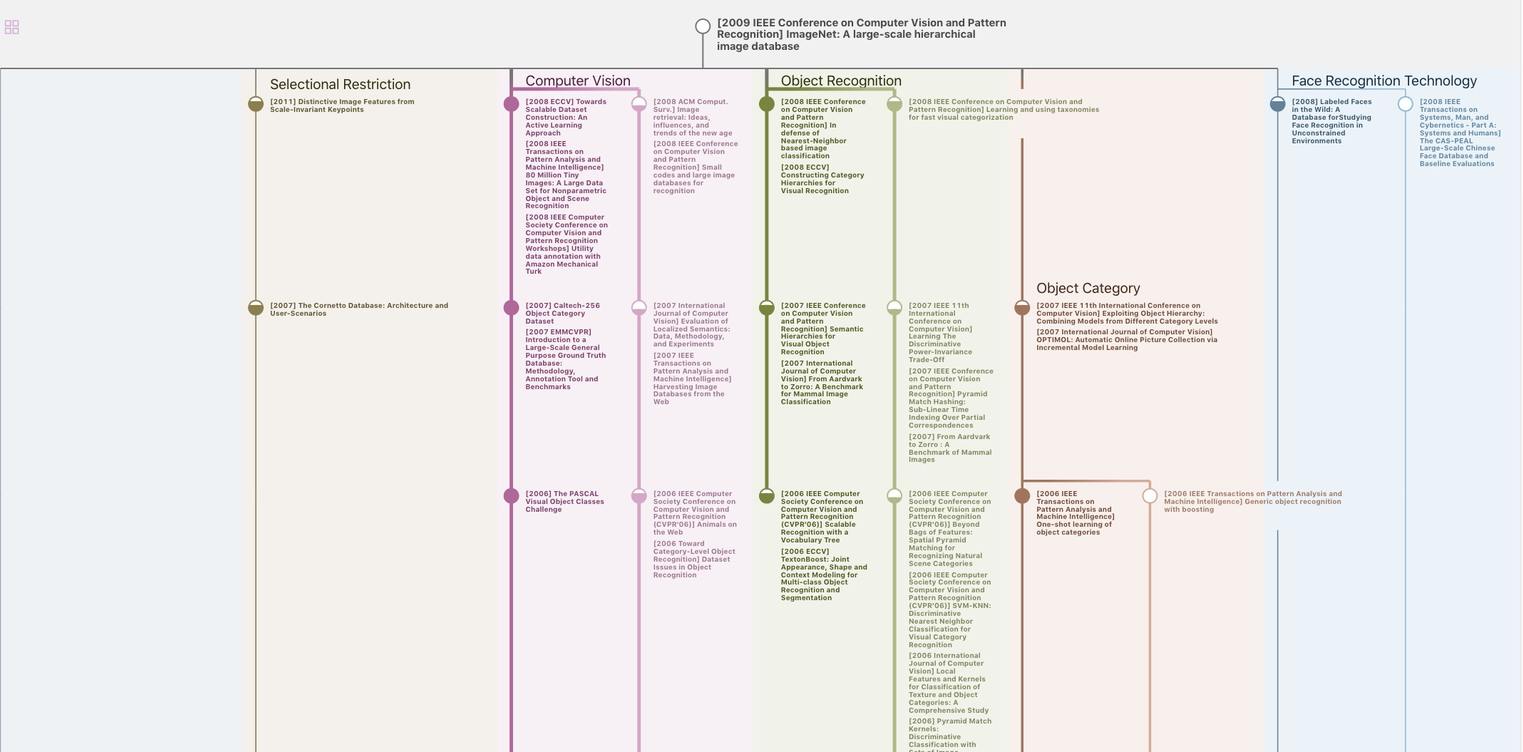
生成溯源树,研究论文发展脉络
Chat Paper
正在生成论文摘要