A Multiscale Interactive Recurrent Network for Time-Series Forecasting
IEEE Transactions on Cybernetics(2022)
摘要
Time-series forecasting is a key component in the automation and optimization of intelligent applications. It is not a trivial task, as there are various short-term and/or long-term temporal dependencies. Multiscale modeling has been considered as a promising strategy to solve this problem. However, the existing multiscale models either apply an implicit way to model the temporal dependencies or ignore the interrelationships between multiscale subseries. In this article, we propose a multiscale interactive recurrent network (MiRNN) to jointly capture multiscale patterns. MiRNN employs a deep wavelet decomposition network to decompose the raw time series into multiscale subseries. MiRNN introduces three key strategies (truncation, initialization, and message passing) to model the inherent interrelationships between multiscale subseries, as well as a dual-stage attention mechanism to capture multiscale temporal dependencies. Experiments on four real-world datasets demonstrate that our model achieves promising performance compared with the state-of-the-art methods.
更多查看译文
关键词
Forecasting,Time Factors
AI 理解论文
溯源树
样例
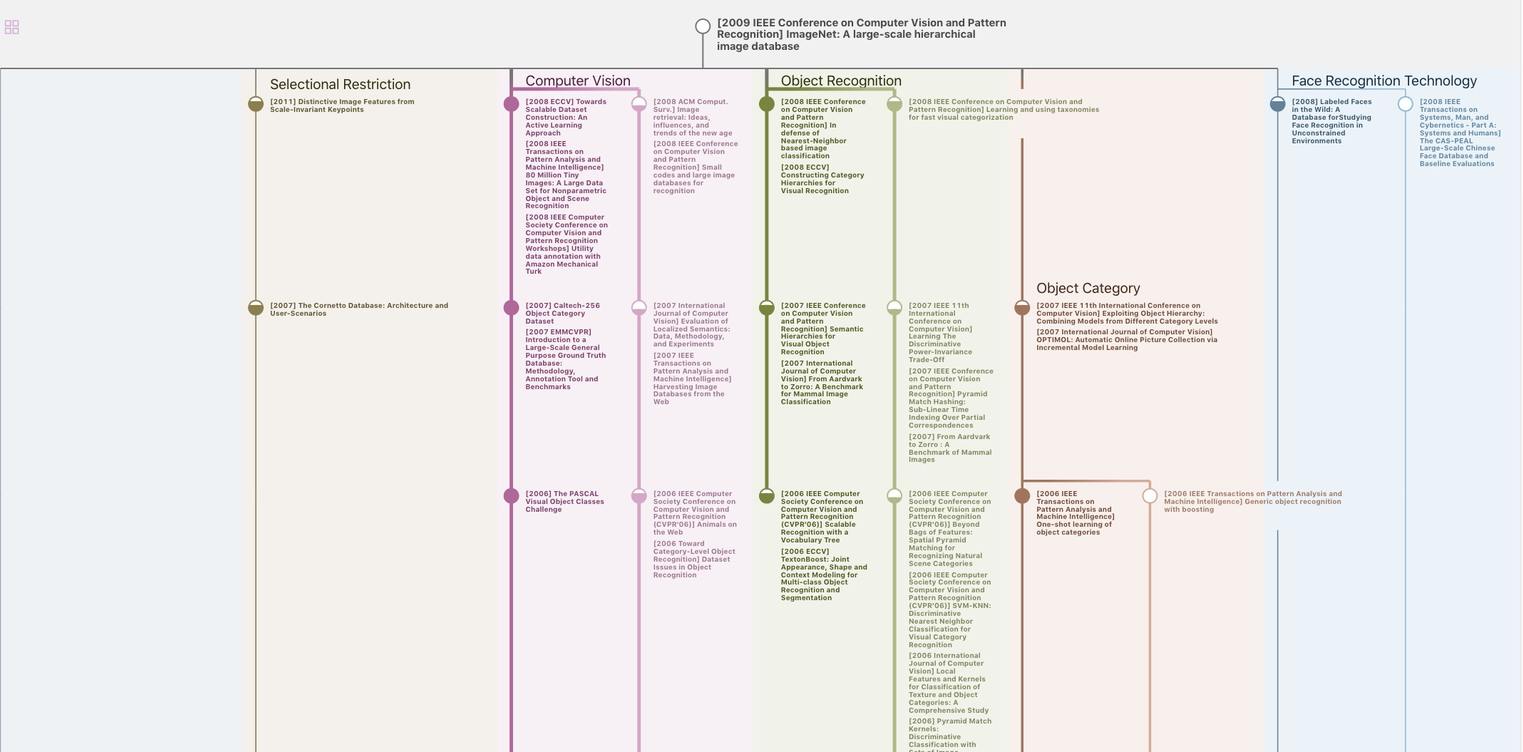
生成溯源树,研究论文发展脉络
Chat Paper
正在生成论文摘要