Learning active nematics one step at a time.
Proceedings of the National Academy of Sciences of the United States of America(2021)
摘要
Machine learning, ranging from regression to deep learning by neural networks, is a set of powerful data analysis tools. Deep learning is especially suited for data-rich but model-limited fields of study, where it has indeed proved to be remarkably successful in tasks such as object and speech recognition. In physics, which aims to describe natural phenomena in terms of universal laws, the application of machine learning tools has been slower, but is gaining traction in recent years (1). The study by Colen et al. (2) applies neural networks as an analysis tool for active-nematic systems, illustrating how these new approaches can provide experimental physicists with better tools to analyze their data. This can involve extracting unknown model parameters from data in systems that are sufficiently well understood, as well as entirely data-driven forecasting approaches that can be used even in the absence of a model.\n\nThe use of machine learning for the analysis and prediction of high dimensional spatiotemporal dynamics is an exciting prospect (3). This is nicely demonstrated by the application of deep neural networks to the study of active-nematic systems (2, 4). The machine learning-based approach is particularly beneficial in situations where a direct measurement of all the relevant degrees of freedom, or of the underlying parameters, is not possible. In the former case, if the information about other degrees of freedom is contained within the observed dynamics, it can be extracted and encoded by the neural network. For the inference of unknown parameters, neural networks can be thought of as nonlinear approximators, which smoothly map spatiotemporal patterns to the parameters of the model. More generally, neural networks effectively provide a dimensionally reduced approximation to the full dynamical system.\n\nApplying machine learning to data is more subtle than simply running a known algorithm, as the approximations involved … \n\n[↵][1]1To whom correspondence may be addressed. Email: kinneret{at}physics.technion.ac.il.\n\n [1]: #xref-corresp-1-1
更多查看译文
AI 理解论文
溯源树
样例
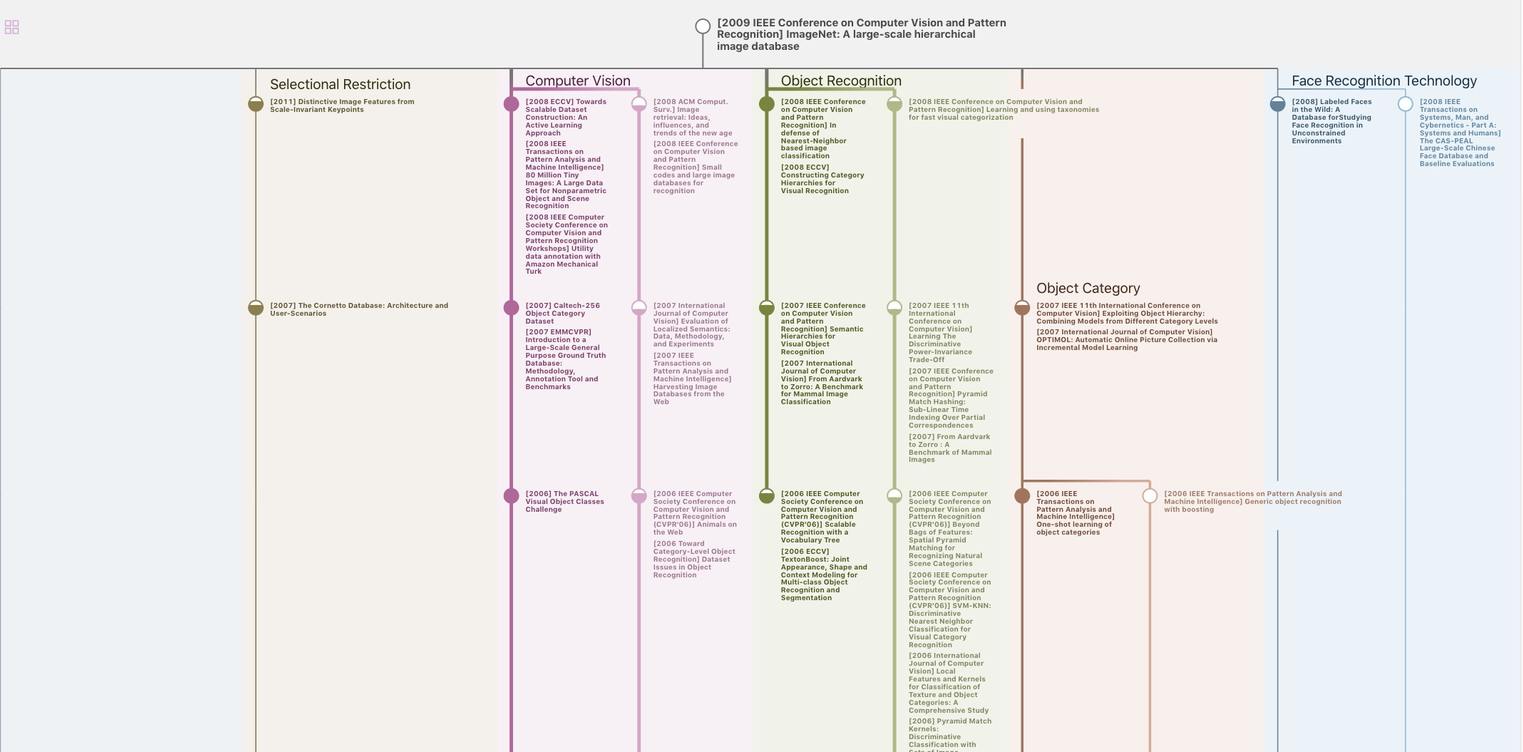
生成溯源树,研究论文发展脉络
Chat Paper
正在生成论文摘要