Recovering Surface Normal And Arbitrary Images: A Dual Regression Network For Photometric Stereo
IEEE TRANSACTIONS ON IMAGE PROCESSING(2021)
摘要
Photometric stereo recovers three-dimensional (3D) object surface normal from multiple images under different illumination directions. Traditional photometric stereo methods suffer from the problem of non-Lambertian surfaces with general reflectance. By leveraging deep neural networks, learning-based methods are capable of improving the surface normal estimation under general non-Lambertian surfaces. These state-of-the-art learning-based methods however do not associate surface normal with reconstructed images and, therefore, they cannot explore the beneficial effect of such association on the estimation of the surface normal. In this paper, we specifically exploit the positive impact of this association and propose a novel dual regression network for both fine surface normals and arbitrary reconstructed images in calibrated photometric stereo. Our work unifies the 3D reconstruction and rendering tasks in a deep learning framework, with the explorations including: 1. generating specified reconstructed images under arbitrary illumination directions, which provides more intuitive perception of the reflectance and is extremely useful for visual applications, such as virtual reality, and 2. our dual regression scheme introduces an additional constraint on observed images and reconstructed images, which forms a closed-loop to provide additional supervision. Experiments show that our proposed method achieves accurate reconstructed images under arbitrarily specified illumination directions and it significantly outperforms the state-of-the-art learning-based single regression methods in calibrated photometric stereo.
更多查看译文
关键词
Surface reconstruction, Image reconstruction, Lighting, Task analysis, Three-dimensional displays, Surface treatment, Estimation, Photometric stereo, surface normal estimation, 3D reconstruction, deep neural networks, dual regression
AI 理解论文
溯源树
样例
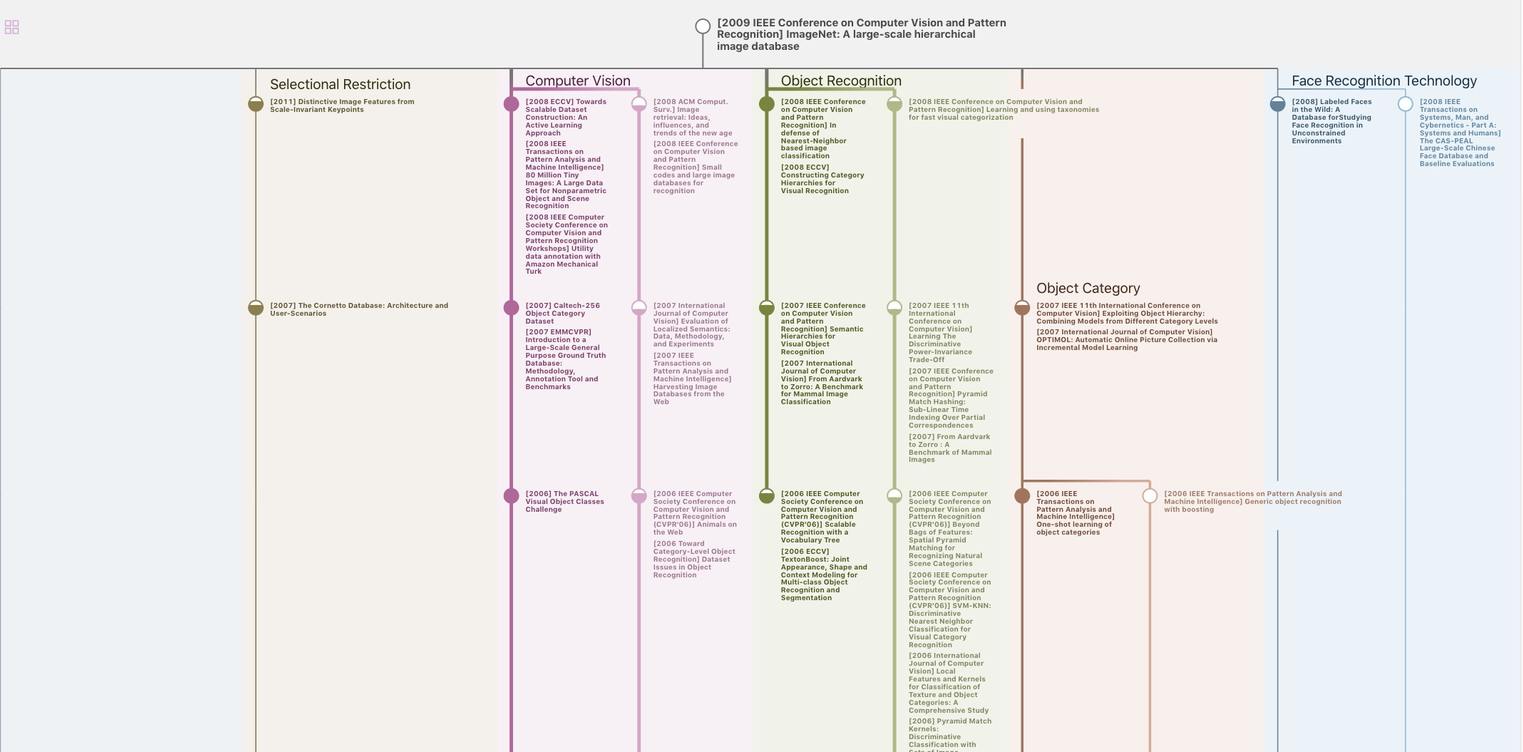
生成溯源树,研究论文发展脉络
Chat Paper
正在生成论文摘要