IBAFormer: Intra-batch Attention Transformer for Domain Generalized Semantic Segmentation
CoRR(2023)
摘要
Domain generalized semantic segmentation (DGSS) is a critical yet challenging task, where the model is trained only on source data without access to any target data. Despite the proposal of numerous DGSS strategies, the generalization capability remains limited in CNN architectures. Though some Transformer-based segmentation models show promising performance, they primarily focus on capturing intra-sample attentive relationships, disregarding inter-sample correlations which can potentially benefit DGSS. To this end, we enhance the attention modules in Transformer networks for improving DGSS by incorporating information from other independent samples in the same batch, enriching contextual information, and diversifying the training data for each attention block. Specifically, we propose two alternative intra-batch attention mechanisms, namely mean-based intra-batch attention (MIBA) and element-wise intra-batch attention (EIBA), to capture correlations between different samples, enhancing feature representation and generalization capabilities. Building upon intra-batch attention, we introduce IBAFormer, which integrates self-attention modules with the proposed intra-batch attention for DGSS. Extensive experiments demonstrate that IBAFormer achieves SOTA performance in DGSS, and ablation studies further confirm the effectiveness of each introduced component.
更多查看译文
关键词
semantic segmentation,attention,intra-batch
AI 理解论文
溯源树
样例
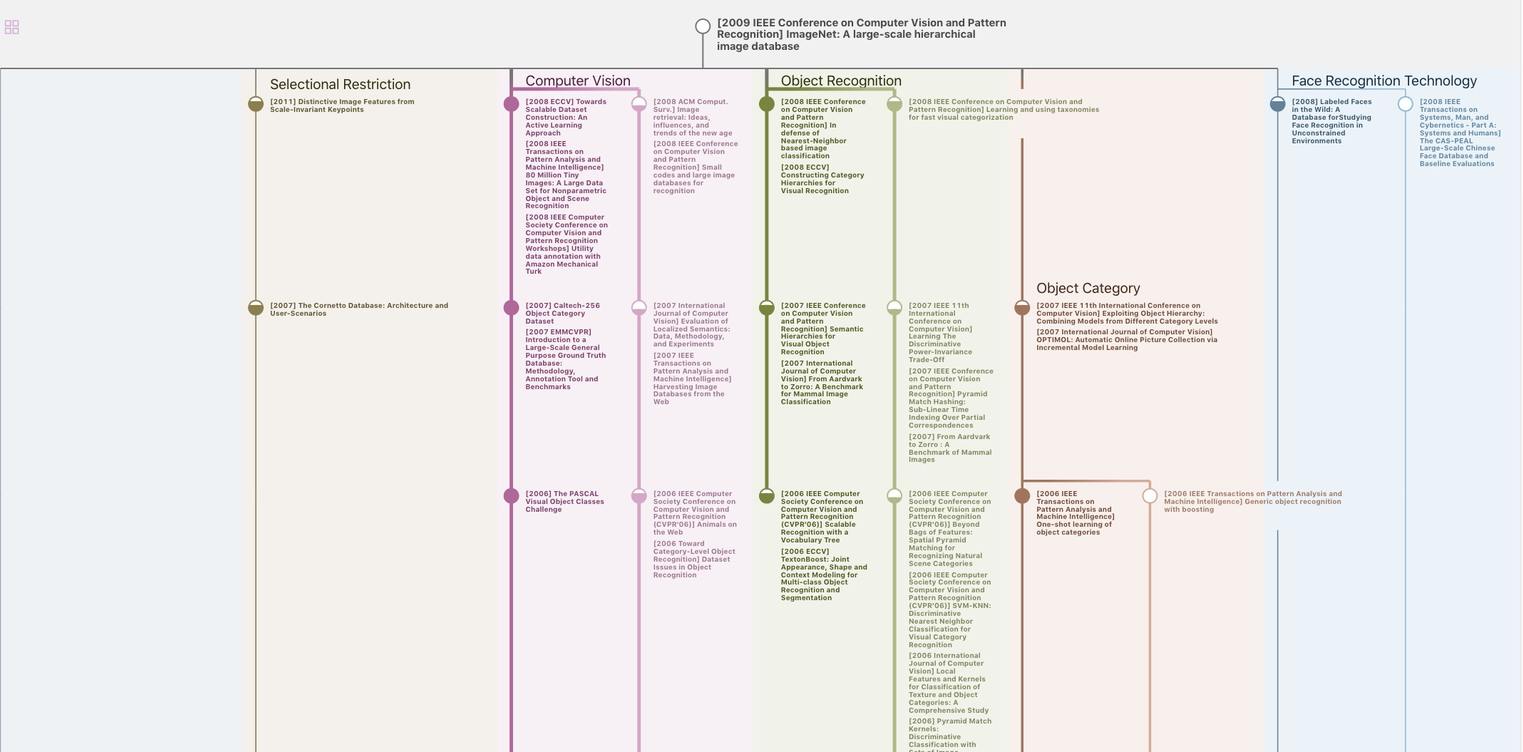
生成溯源树,研究论文发展脉络
Chat Paper
正在生成论文摘要