Risk Prediction Model For Early Outcomes Of Revascularization For Chronic Limb-Threatening Ischaemia
BRITISH JOURNAL OF SURGERY(2021)
摘要
Background: Quantifying the risks and benefits of revascularization for chronic limb-threatening ischaemia (CLTI) is important. The aim of this study was to create a risk prediction model for treatment outcomes 30 days after revascularization in patients with CLTI.Methods: Consecutive patients with CLTI who had undergone revascularization between 2013 and 2016 were collected from the JAPAN Critical Limb Ischemia Database (JCLIMB). The cohort was divided into a development and a validation cohort. In the development cohort, multivariable risk models were constructed to predict major amputation and/or death and major adverse limb events using least absolute shrinkage and selection operator logistic regression. This developed model was applied to the validation cohort and its performance was evaluated using c-statistic and calibration plots.Results: Some 2906 patients were included in the analysis. The major amputation and/or mortality rate within 30 days of arterial reconstruction was 5.0 per cent (144 of 2906), and strong predictors were abnormal white blood cell count, emergency procedure, congestive heart failure, body temperature of 38 degrees C or above, and hemodialysis. Conversely, moderate, low or no risk in the Geriatric Nutritional Risk Index (GNRI) and ambulatory status were associated with improved results. The c-statistic value was 0.82 with high prediction accuracy. The rate of major adverse limb events was 6.4 per cent (185 of 2906), and strong predictors were abnormal white blood cell count and body temperature of 38 degrees C or above. Moderate, low or no risk in the GNRI, and age greater than 84 years were associated with improved results. The c-statistic value was 0.79, with high prediction accuracy.Conclusion: This risk prediction model can help in deciding on the treatment strategy in patients with CLTI and serve as an index for evaluating the quality of each medical facility.
更多查看译文
AI 理解论文
溯源树
样例
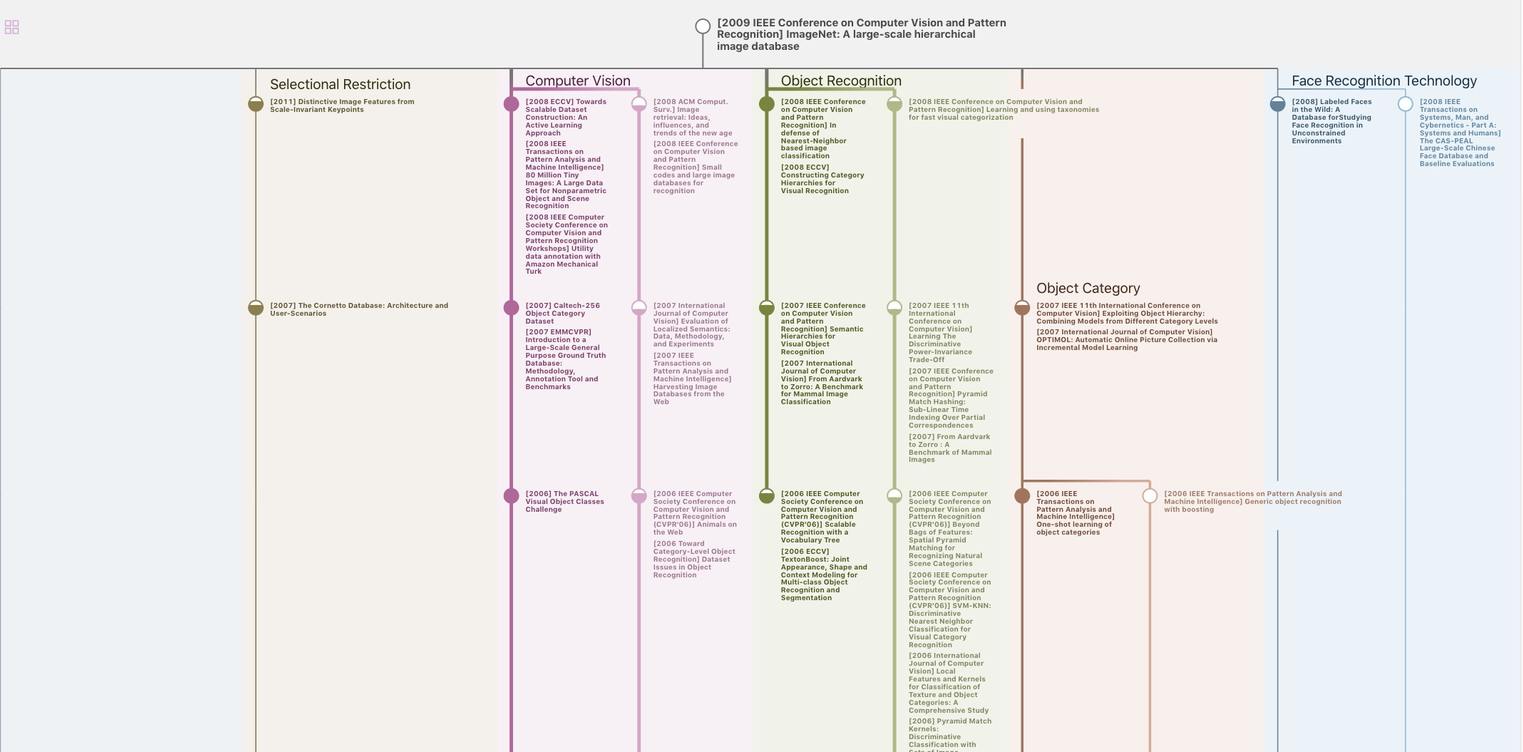
生成溯源树,研究论文发展脉络
Chat Paper
正在生成论文摘要