9.4 PIU: A 248GOPS/W Stream-Based Processor for Irregular Probabilistic Inference Networks Using Precision-Scalable Posit Arithmetic in 28nm
2021 IEEE International Solid- State Circuits Conference (ISSCC)(2021)
摘要
While deep neural networks have become an indispensable tool in today's smart devices, their usage is also criticized due to lack of explainability, inability to include domain knowledge, and a need for large volumes of training data. To overcome this, researchers are increasingly using probabilistic models as a part of the system [1] [2] [3] (Fig. 9.4.1). For example, Stelzner et al. [2] complements neural networks with probabilistic models for efficient unsupervised scene understanding robust to noise. Zheng et al. [3] uses a probabilistic model for end-to-end semantic environment mapping during robotic navigation. While sampling techniques are usually used for approximate inference with probabilistic models, fast exact inference is often tractable by using Sum-Product Networks (SPN, also called probabilistic circuits) [4]. This SPN-based inference is preferred over sampling techniques because it provides deterministic results, avoids error-accumulation, and can enable inference in discrete probabilistic programs.
更多查看译文
关键词
irregular probabilistic inference networks,precision-scalable posit arithmetic,deep neural networks,end-to-end semantic environment mapping,approximate inference,Sum-Product Networks,probabilistic circuits,SPN-based inference,discrete probabilistic programs
AI 理解论文
溯源树
样例
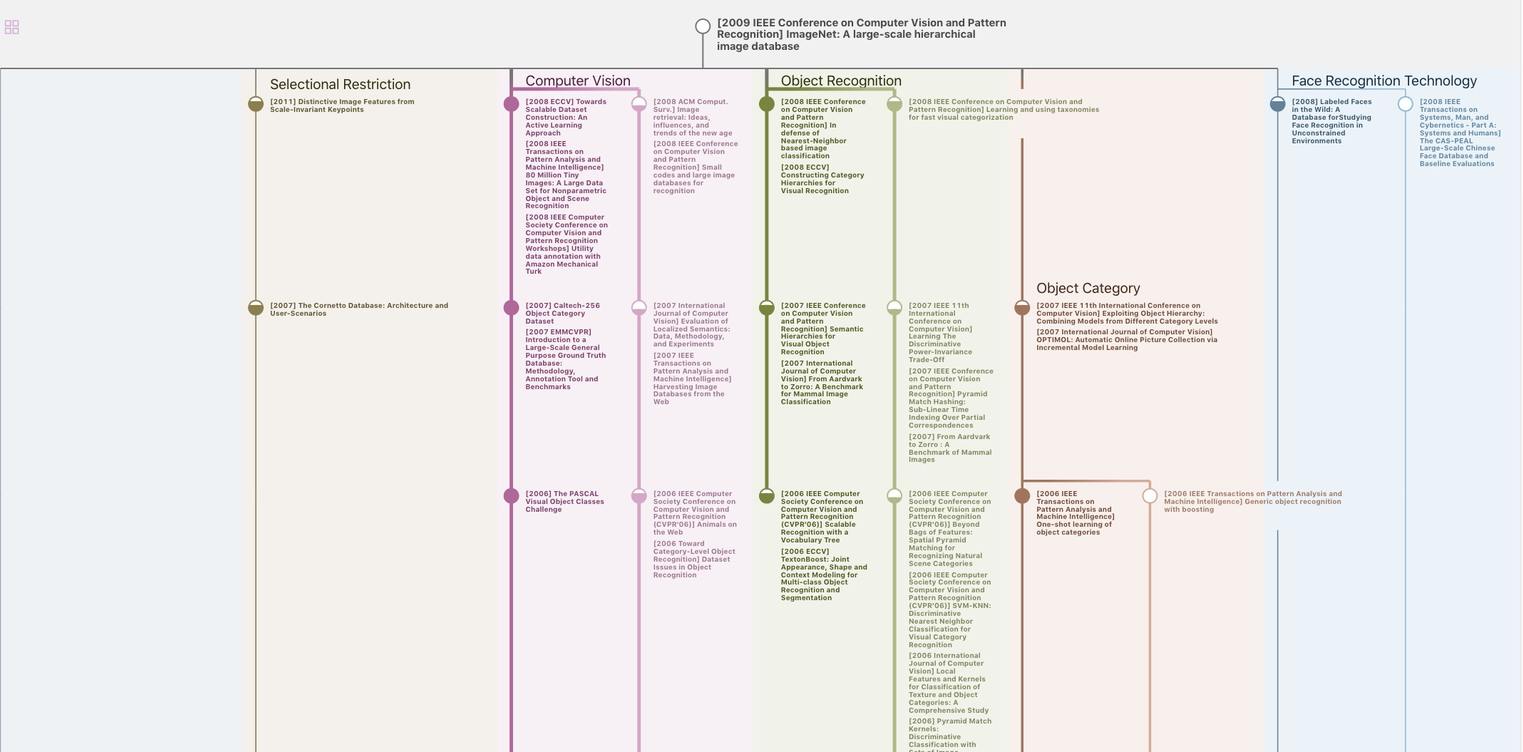
生成溯源树,研究论文发展脉络
Chat Paper
正在生成论文摘要