Forecasting of Ionospheric Critical Frequency Using Neural Networks
CHINESE JOURNAL OF SPACE SCIENCE(2005)
摘要
The use of feed-forward back propagation neural networks to predict ionospheric F2 layer critical frequency, f0F2, 24 h ahead, have been examined. The data we used are from Wuhan ionospheric station, China, and Hobart ionospheric station, Australia. The data period is from 1970 to 1990 at Wuhan and from 1962 to 1990 at Hobart. The five day's measurements of f0F2 before the day that need forecast are reduced to five coefficients. The inputs used for the BP neural network are the coefficients, the solar 10.7cm flux index, and the outputs are the day's 24 h observed f0F2 data. The trained net then can forecast f0F2 24 h advance. The result indicates the predicted f0F2 using NN has good agreement with observed data. Comparison with IRI model suggests that NN method is more accurate than IRI. In addition, the error analysis indicates that predicted f0F2's Root-Mean-Square Error (RMSE) is smaller in Hobart than in Wuhan, smaller in low solar activity than in high solar activity, smaller in winter and summer than in spring and autumn. In conclusion, using neural network to predict ionospheric parameters is a feasible method.
更多查看译文
AI 理解论文
溯源树
样例
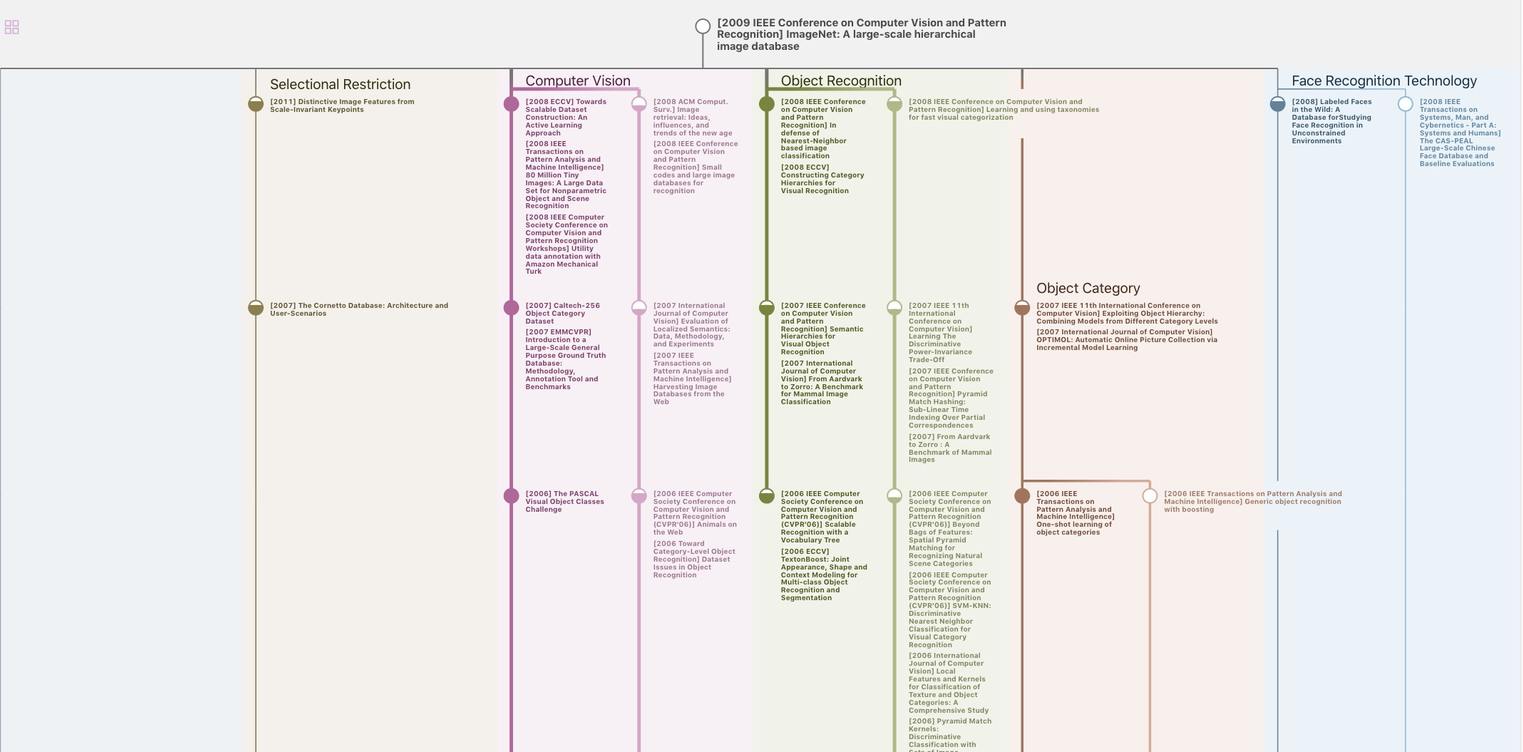
生成溯源树,研究论文发展脉络
Chat Paper
正在生成论文摘要