Bayesian Model For Pedestrian'S Behavior Analysis Based On Image And Video Processing
JOURNAL OF ELECTRONIC IMAGING(2021)
摘要
Road accidents continue to increase and cause intense fatalities. Studies based on manual and/or semiautomatic methods remain unable to reliably track the random behavior of pedestrians. Hence, we fill this gap by developing an automatic Bayesian and cognitive model (ABC model) for pedestrian behavior analysis. We propose a full and complete computer vision process composed of two images processing levels: (i) low-level for pedestrian and traffic features extraction to characterize crossing the street scenarios. (ii) High-level for data correlation and behavior recognition based on Bayesian model. Since computer vision sensors are usually unsure, we propose an innovative metric to introduce the detection uncertainty as Bayesian evidence in the Bayesian network decision level. Results highlight computer vision techniques' potential to collect random and reliable road user's data at a degree of automation and accuracy that cannot be feasibly achieved by manual or semiautomated techniques during the first time. During the second time, the Bayesian network structure provides very reliable decisions that can more completely characterize a person's random behavior. In addition to that, quantifying the uncertainty of computer vision's sensors as Bayesian evidence is an important contribution. In fact, the proposed tool will be an important contribution to deal with pedestrian behavior. Hence, the proposed process reliably addresses a person's Bayes behavior and illustrates the pedestrian/environment causal relationship. The proposed ABC model is validated based on cross street sequences integrating different scenarios and pedestrians' behaviors every 1/10 time slice. (c) 2021 SPIE and IS&T [DOI: 10.1117/1.JEI.30.1.013019]
更多查看译文
关键词
vision-based data collection, pedestrian behavior, Bayesian network, confidence degree, evidence generation
AI 理解论文
溯源树
样例
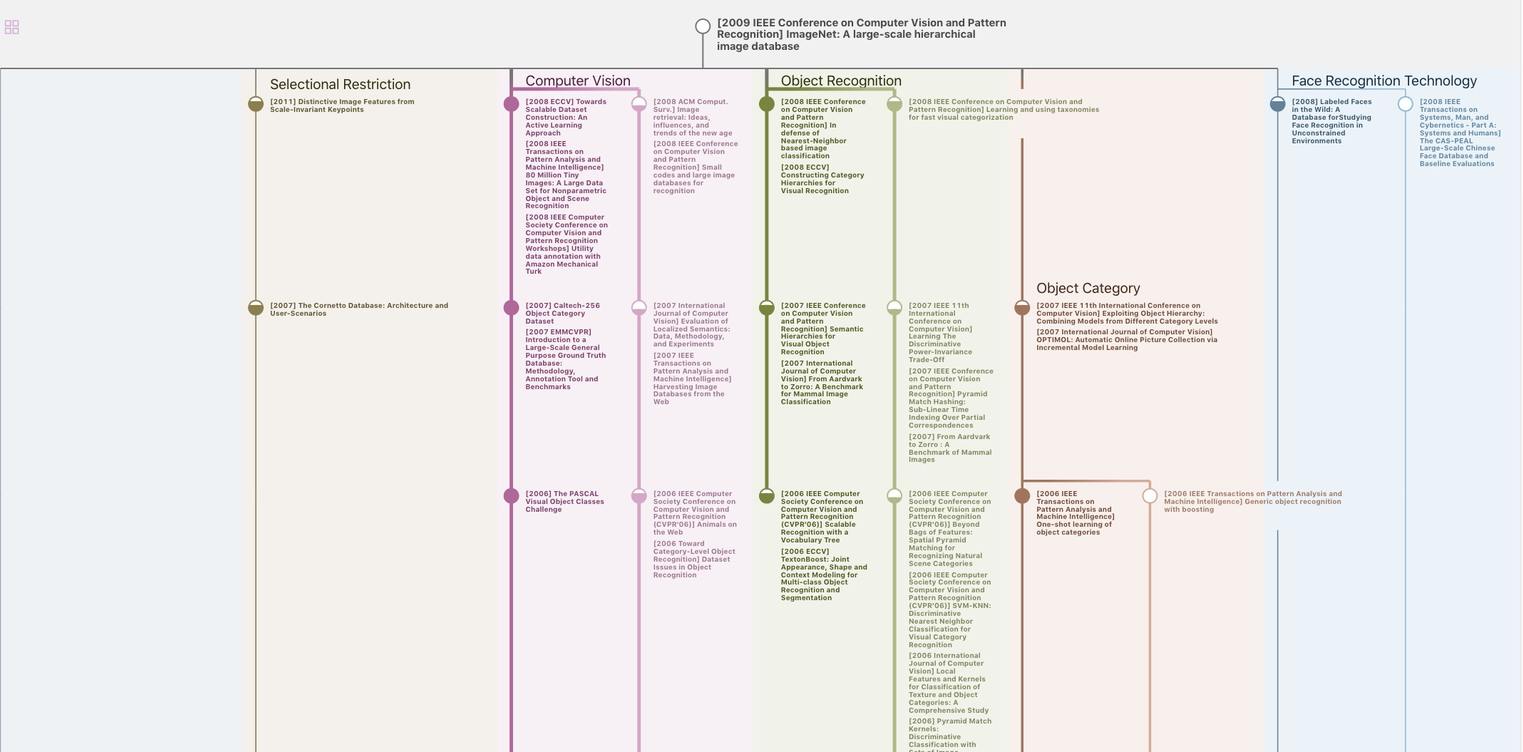
生成溯源树,研究论文发展脉络
Chat Paper
正在生成论文摘要