Monotonic Alpha-divergence Minimisation for Variational Inference
JOURNAL OF MACHINE LEARNING RESEARCH(2023)
摘要
In this paper, we introduce a novel family of iterative algorithms which carry out alpha-divergence minimisation in a Variational Inference context. They do so by ensuring a systematic decrease at each step in the alpha-divergence between the variational and the posterior distributions. In its most general form, the variational distribution is a mixture model and our framework allows us to simultaneously optimise the weights and components parameters of this mixture model. Our approach permits us to build on various methods previously proposed for alpha-divergence minimisation such as Gradient or Power Descent schemes and we also shed a new light on an integrated Expectation Maximization algorithm. Lastly, we provide empirical evidence that our methodology yields improved results on several multimodal target distributions and on a real data example.
更多查看译文
关键词
Variational Inference,Kullback-Leibler,Alpha-Divergence,Mixture Models,Bayesian Inference
AI 理解论文
溯源树
样例
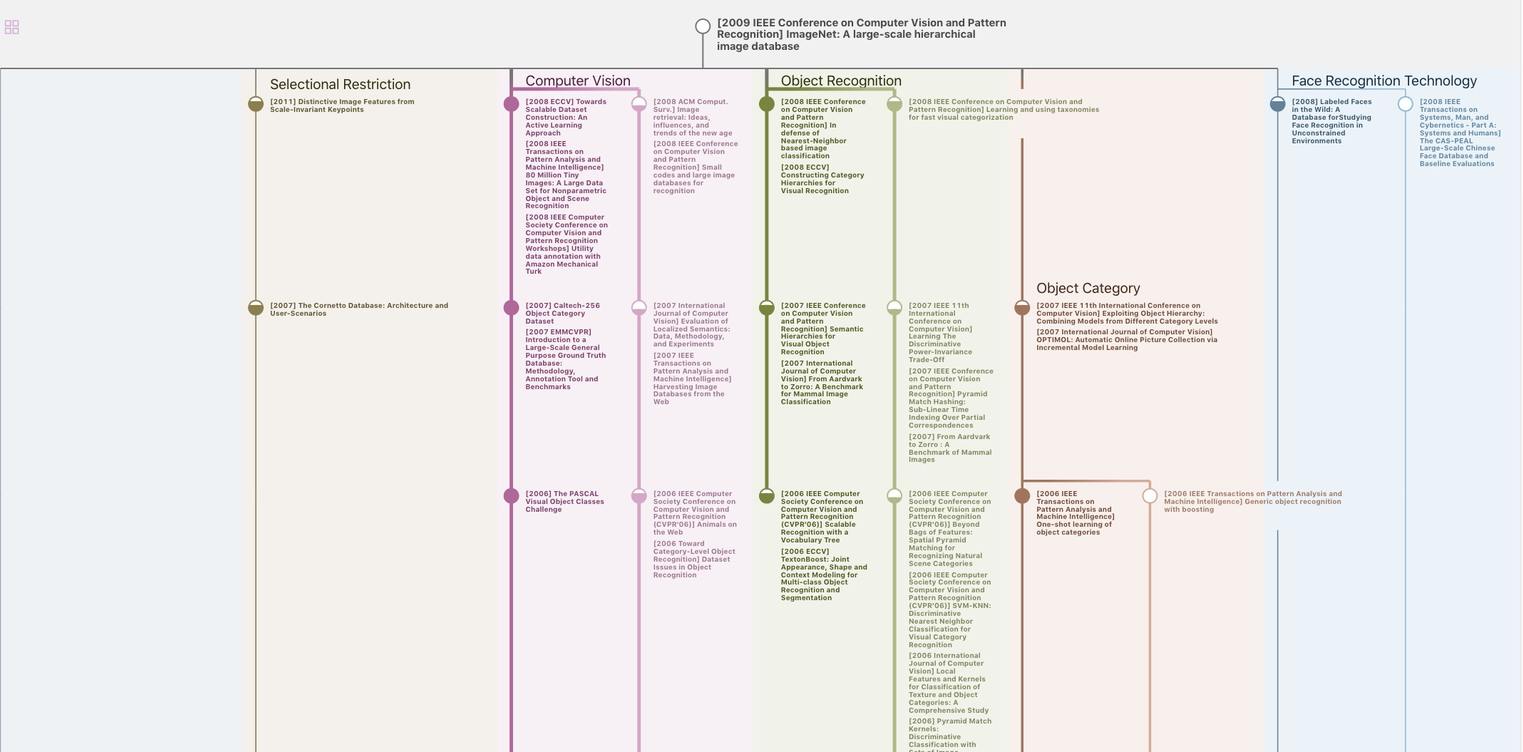
生成溯源树,研究论文发展脉络
Chat Paper
正在生成论文摘要