Semi-supervised Domain Adaptation based on Dual-level Domain Mixing for Semantic Segmentation
2021 IEEE/CVF CONFERENCE ON COMPUTER VISION AND PATTERN RECOGNITION, CVPR 2021(2021)
摘要
Data-driven based approaches, in spite of great success in many tasks, have poor generalization when applied to unseen image domains, and require expensive cost of annotation especially for dense pixel prediction tasks such as semantic segmentation. Recently, both unsupervised domain adaptation (UDA) from large amounts of synthetic data and semi-supervised learning (SSL) with small set of labeled data have been studied to alleviate this issue. However, there is still a large gap on performance compared to their supervised counterparts. We focus on a more practical setting of semi-supervised domain adaptation (SSDA) where both a small set of labeled target data and large amounts of labeled source data are available. To address the task of SSDA, a novel framework based on dual-level domain mixing is proposed. The proposed framework consists of three stages. First, two kinds of data mixing methods are proposed to reduce domain gap in both region-level and sample-level respectively. We can obtain two complementary domain-mixed teachers based on dual-level mixed data from holistic and partial views respectively. Then, a student model is learned by distilling knowledge from these two teachers. Finally, pseudo labels of unlabeled data are generated in a self-training manner for another few rounds of teachers training. Extensive experimental results have demonstrated the effectiveness of our proposed framework on synthetic-to-real semantic segmentation benchmarks.
更多查看译文
关键词
semantic segmentation,data-driven based approaches,unseen image domains,dense pixel prediction tasks,unsupervised domain adaptation,synthetic data,semisupervised learning,supervised counterparts,semisupervised domain adaptation,labeled target data,labeled source data,dual-level domain mixing,data mixing methods,domain gap,region-level,sample-level,complementary domain-mixed teachers,dual-level mixed data,unlabeled data
AI 理解论文
溯源树
样例
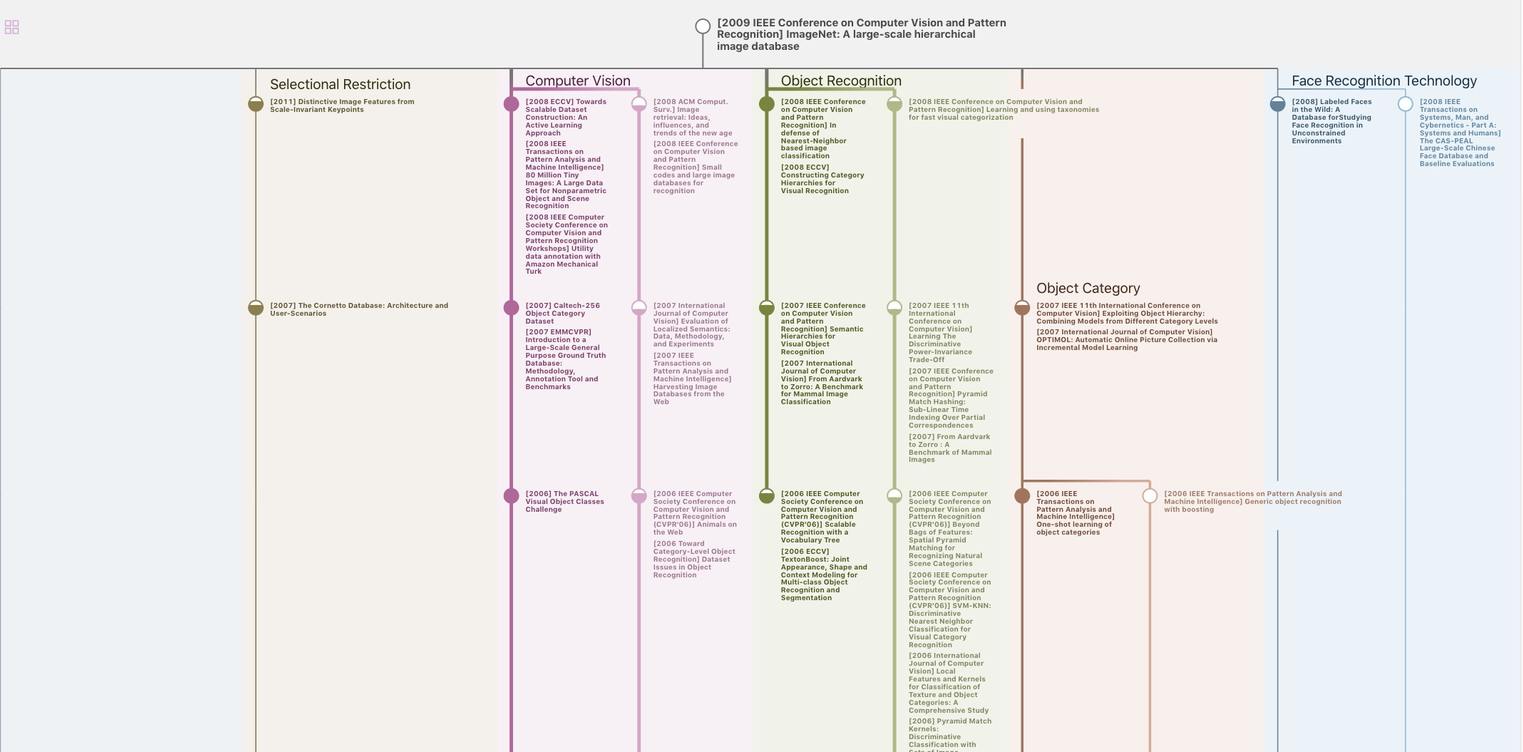
生成溯源树,研究论文发展脉络
Chat Paper
正在生成论文摘要