Deep Chestx-Ray: Detection And Classification Of Lesions Based On Deep Convolutional Neural Networks
INTERNATIONAL JOURNAL OF IMAGING SYSTEMS AND TECHNOLOGY(2021)
摘要
We investigated whether a convolutional neural network (CNN) can enhance the usability of computer-aided detection (CAD) of chest radiographs for various pulmonary abnormal lesions. The numbers of normal and abnormal patients were 6055 and 3463, respectively. Two radiologists delineated regions of interest for lesions and labeled the disease types as ground truths. The datasets were split into training, tuning, and testing as 7:1: 2. Total test sets were randomly selected in 1214 normal and 690 abnormal. A 5-fold, cross-validation was performed on our datasets. For the classification of normal and abnormal, we developed a CNN based on DenseNet169; for abnormal detection, The You Only Look Once (YOLO) v2 with DenseNet was used. Detection and classification of normal and five classes of diseases (nodule[s], consolidation, interstitial opacity, pleural effusion, and pneumothorax) on chest radiographs were analyzed. Our CNN model classified chest radiographs as normal or abnormal with an accuracy of 97.8%. For the results of the abnormal, F1 score, was 75.2 +/- 2.28% for nodules, 55.0 +/- 4.3% for consolidation, 78.2 +/- 7.85% for interstitial opacity, 81.6 +/- 2.07% for pleural effusion, and 70.0 +/- 7.97% for pneumothorax, respectively. In addition, we conducted the experiments between our method and RetinaNet with only nodules. The results of our method and RetinaNet at cutoff-0.5 in the free response operating characteristic curve were 83.45% and 80.55%, respectively. Our algorithm demonstrated viable detection and disease classification capacity and could be used for CAD of lung diseases on chest radiographs.
更多查看译文
关键词
chest radiographs, computer-aided detection, deep learning, lung diseases, machine learning, radiography
AI 理解论文
溯源树
样例
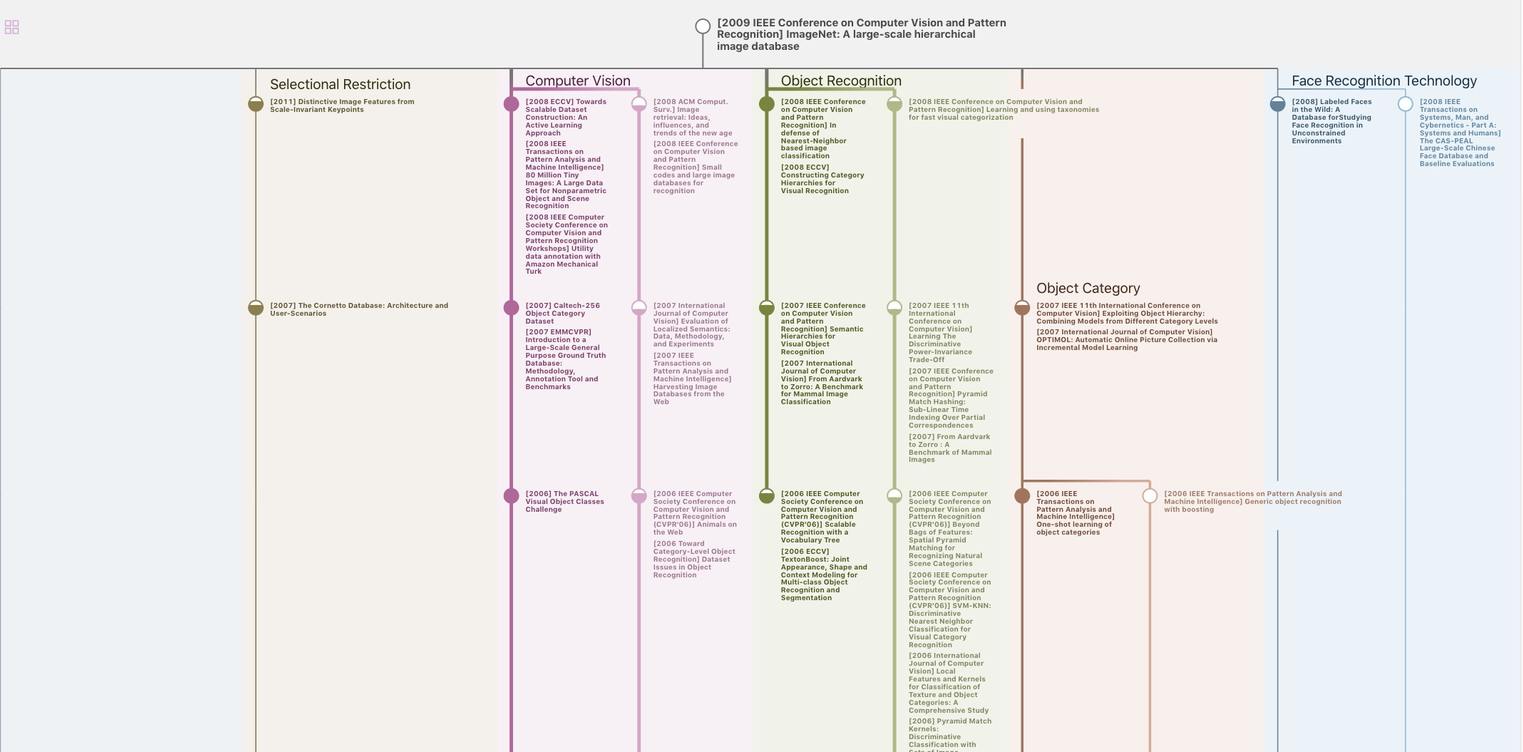
生成溯源树,研究论文发展脉络
Chat Paper
正在生成论文摘要