A Regularized Tensor Completion Approach for PMU Data Recovery
IEEE Transactions on Smart Grid(2021)
摘要
This article presents a novel data recovery framework for missing synchrophasor measurements. The imputation accuracy for the existing data recovery methods is significantly reduced when there are consecutive data losses across multiple data streams. Besides, the recovered data do not necessarily meet the physical constraints of the power grid. To tackle these issues, a regularized low rank tensor completion (LRTC) method is proposed to incorporate the domain knowledge (e.g., Kirchhoff's voltage and current laws, and three phase circuit relationships) as the regularization terms in order to efficiently exploit the data inter-dependencies for a better recovery. We leverage the tensor decomposition and completion as powerful tools to extract the latent structures of phasor measurement unit (PMU) data for the recovery process. Specifically, we first construct the tensor model of the PMU data and then formulate the LRTC problem as a rank minimization by leveraging the low rank property of the PMU measurements and adding the regularization terms into the LRTC problem in order to improve the imputation accuracy. An efficient algorithm based on alternating direction method of multipliers (ADMM) is developed to solve the regularized LRTC problem. The experiments using the real PMU dataset show that the proposed approach exhibits better imputation accuracy, compared with the conventional matrix completion methods.
更多查看译文
关键词
Missing data recovery,phasor measurement unit,tensor decomposition,regularized tensor completion
AI 理解论文
溯源树
样例
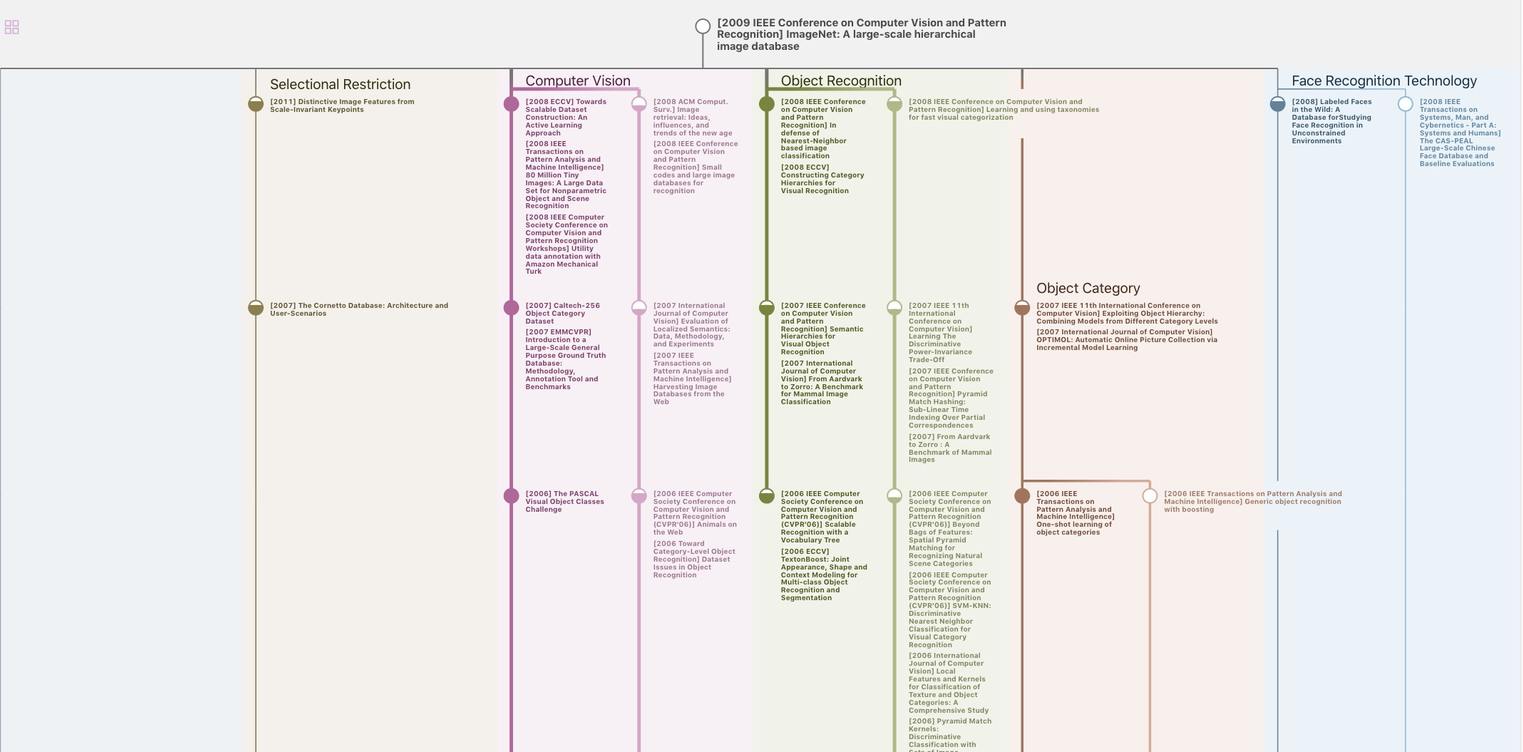
生成溯源树,研究论文发展脉络
Chat Paper
正在生成论文摘要