WordBias: An Interactive Visual Tool for Discovering Intersectional Biases Encoded in Word Embeddings
Conference on Human Factors in Computing Systems(2021)
摘要
BSTRACTIntersectional bias is a bias caused by an overlap of multiple social factors like gender, sexuality, race, disability, religion, etc. A recent study has shown that word embedding models can be laden with biases against intersectional groups like African American females, etc. The first step towards tackling intersectional biases is to identify them. However, discovering biases against different intersectional groups remains a challenging task. In this work, we present WordBias, an interactive visual tool designed to explore biases against intersectional groups encoded in static word embeddings. Given a pretrained static word embedding, WordBias computes the association of each word along different groups like race, age, etc. and then visualizes them using a novel interactive interface. Using a case study, we demonstrate how WordBias can help uncover biases against intersectional groups like Black Muslim Males, Poor Females, etc. encoded in word embedding. In addition, we also evaluate our tool using qualitative feedback from expert interviews. The source code for this tool can be publicly accessed for reproducibility at github.com/bhavyaghai/WordBias.
更多查看译文
关键词
Algorithmic Fairness, Visual Analytics, Word Embeddings
AI 理解论文
溯源树
样例
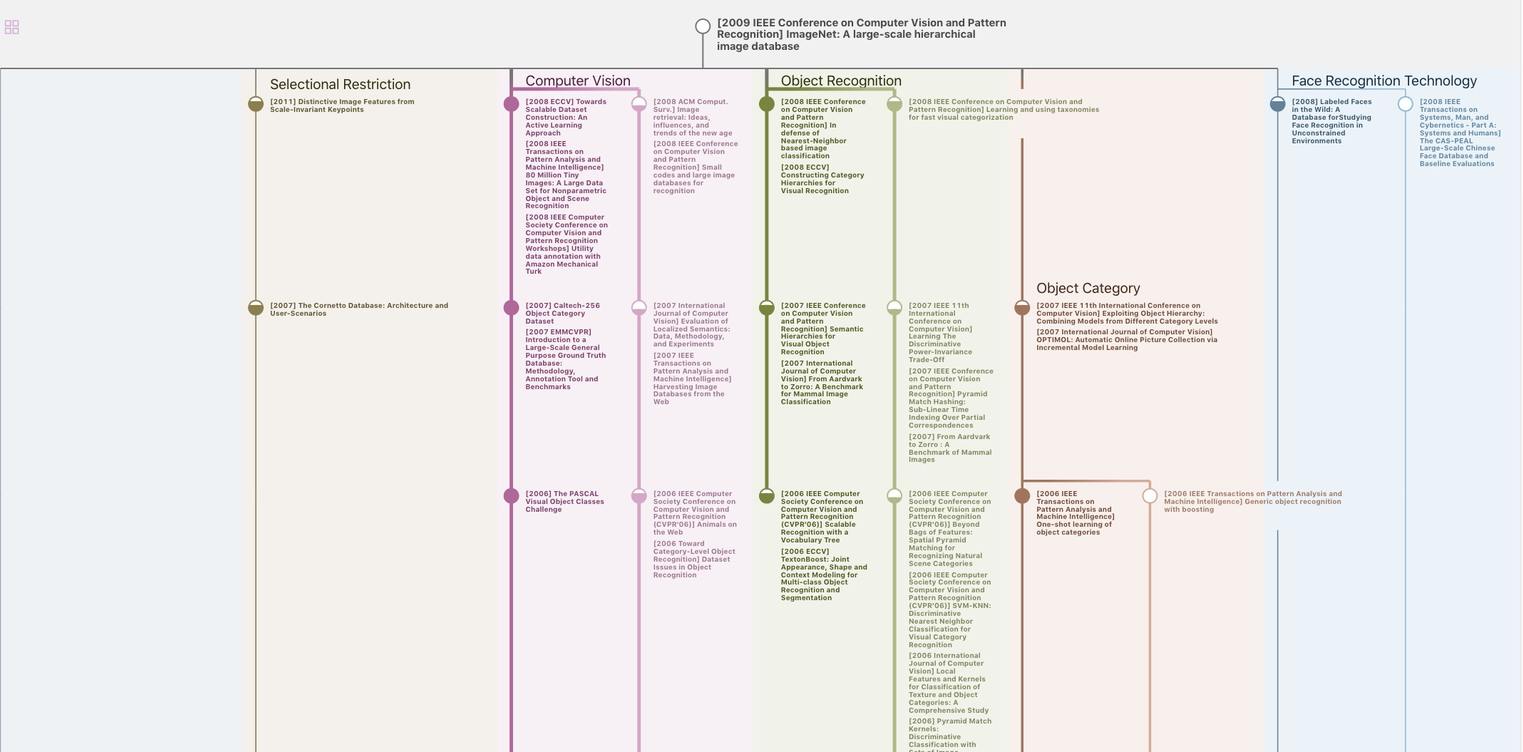
生成溯源树,研究论文发展脉络
Chat Paper
正在生成论文摘要