DeepTag: An Unsupervised Deep Learning Method for Motion Tracking on Cardiac Tagging Magnetic Resonance Images
2021 IEEE/CVF CONFERENCE ON COMPUTER VISION AND PATTERN RECOGNITION, CVPR 2021(2021)
摘要
Cardiac tagging magnetic resonance imaging (t-MRI) is the gold standard for regional myocardium deformation and cardiac strain estimation. However, this technique has not been widely used in clinical diagnosis, as a result of the difficulty of motion tracking encountered with t-MRI images. In this paper, we propose a novel deep learning-based fully unsupervised method for in vivo motion tracking on t-MRI images. We first estimate the motion field (INF) between any two consecutive t-MRI frames by a bi-directional generative diffeomorphic registration neural network. Using this result, we then estimate the Lagrangian motion field between the reference frame and any other frame through a differentiable composition layer. By utilizing temporal information to perform reasonable estimations on spatiotemporal motion fields, this novel method provides a useful solution for motion tracking and image registration in dynamic medical imaging. Our method has been validated on a representative clinical t-MRI dataset; the experimental results show that our method is superior to conventional motion tracking methods in terms of landmark tracking accuracy and inference efficiency. Project page is at: https://github.com/DeepTag/cardiac_tagging_motion_estimation.
更多查看译文
关键词
motion tracking,unsupervised deeptag learning method,deeptag learning
AI 理解论文
溯源树
样例
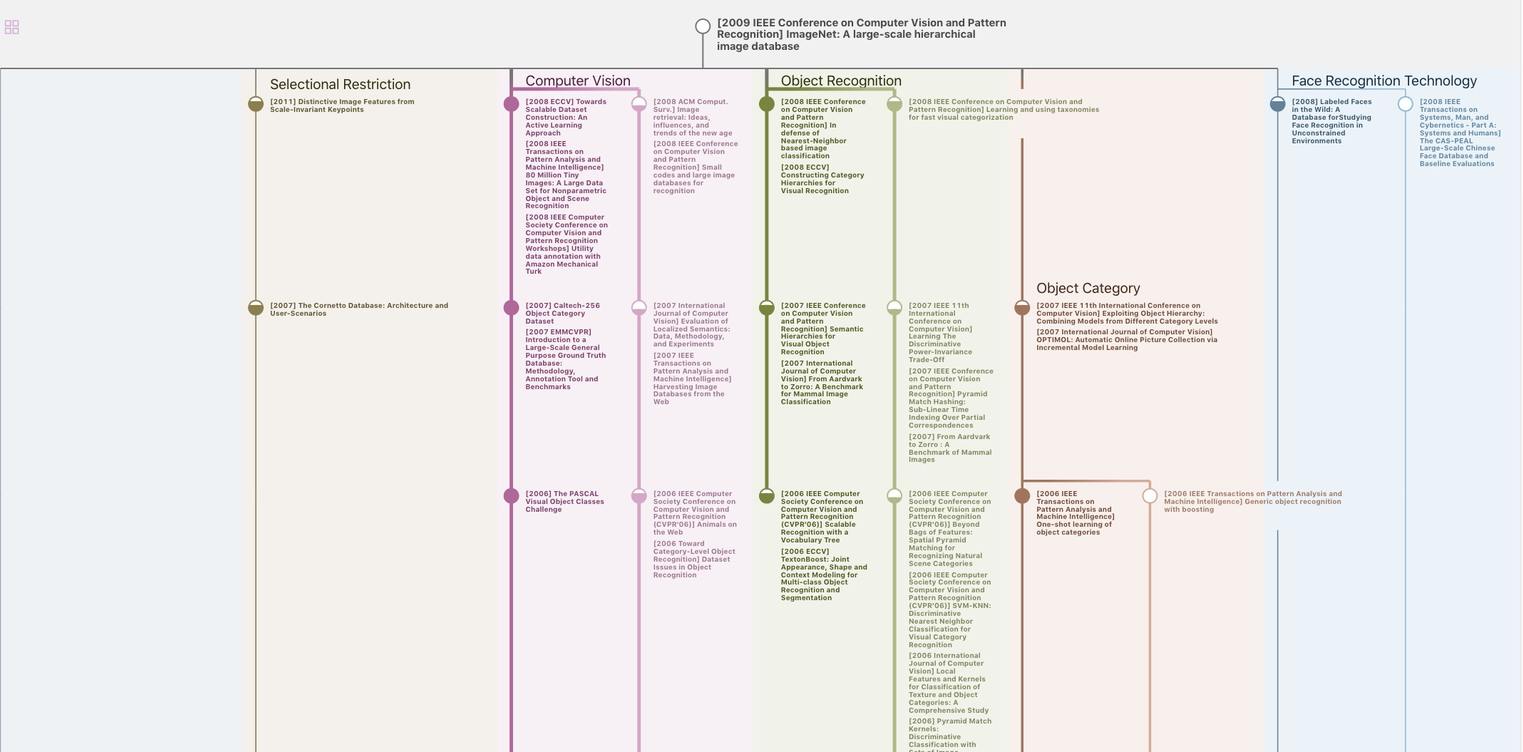
生成溯源树,研究论文发展脉络
Chat Paper
正在生成论文摘要