Time-First Tracking: An Efficient Multiple-Object Tracking Architecture For Dynamic Surveillance Environments
PROCEEDINGS OF THE 10TH INTERNATIONAL CONFERENCE ON PATTERN RECOGNITION APPLICATIONS AND METHODS (ICPRAM)(2021)
摘要
Given the countless hours of video that are generated in surveillance environments, real-time for multi-object tracking (MOT) is vastly insufficient. Current MOT methods prioritize tracking accuracy in crowded environments, with little concern for total computational expense, which has led to a reliance on expensive object detectors to perform tracking. Indiscriminate use of object detectors is not scalable for surveillance problems and ignores the inherent spatio-temporal variation in scene complexity in many real-world environments. A novel MOT method is proposed, termed "Time-First Tracking", which relies on "shallowly" processed motion with a new tracking method, leaving the use of expensive object detection methods to an "as-needed" basis. The resulting vast reduction in pixels-processed may yield orders of magnitude in cost savings, making MOT more tractable. Time-First Tracking is adaptable to spatio-temporal changes in tracking difficulty; videos are divided into spatio-temporal sub-volumes, rated with different tracking difficulties, that are subsequently processed with different object localization methods. New MOT metrics are proposed to account for cost along with code to create a synthetic MOT dataset for motion-based tracking.
更多查看译文
关键词
Multiple Object Tracking, DBSCAN, Background Subtraction, and Tracking by Detection
AI 理解论文
溯源树
样例
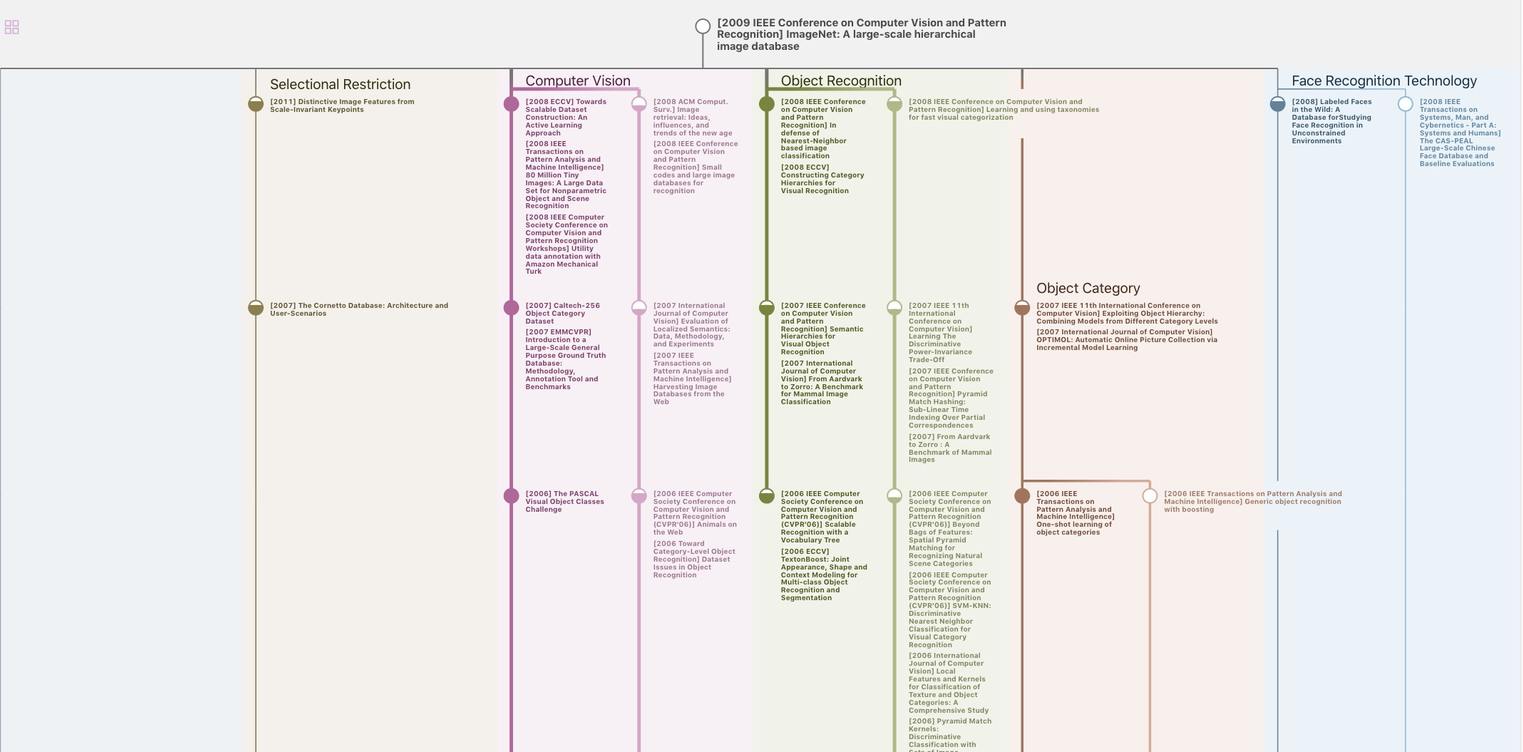
生成溯源树,研究论文发展脉络
Chat Paper
正在生成论文摘要