Sigrnn: Synthetic Minority Instances Generation In Imbalanced Datasets Using A Recurrent Neural Network
PROCEEDINGS OF THE 10TH INTERNATIONAL CONFERENCE ON PATTERN RECOGNITION APPLICATIONS AND METHODS (ICPRAM)(2021)
摘要
Machine learning models trained on imbalanced datasets tend to produce sub-optimal results. This happens because the learning of the minority classes is dominated by the learning of the majority class. Recommendations to overcome this obstacle include oversampling the minority class by synthesizing new instances and using different performance measures. We propose a novel approach to handle the imbalance in datasets by using a sequence-to-sequence recurrent neural network to synthesize minority class instances. The generative neural network is trained on the minority class instances to learn its data distribution; the generative neural network is then used to synthesize minority class instances; these instances are used to augment the original dataset and balance the minority class. We evaluate our proposed approach against several imbalanced datasets. We train Decision Tree models on the original and augmented datasets and compare their results against the Synthetic Minority Over-sampling TEchnique (SMOTE), Adaptive Synthetic sampling (ADASYN) and Synthetic Minority Over-sampling TEchnique-Nominal Continuous (SMOTE-NC). All results are an average of multiple runs and the results are compared across four different performance metrics. SIGRNN performs well compared to SMOTE and ADASYN, specifically in lower percentage increments to the minority class. Also, SIGRNN outperforms SMOTE-NC on datasets having nominal features.
更多查看译文
关键词
Synthetic Data, Balancing, Oversampling, Classification, Imbalanced Dataset
AI 理解论文
溯源树
样例
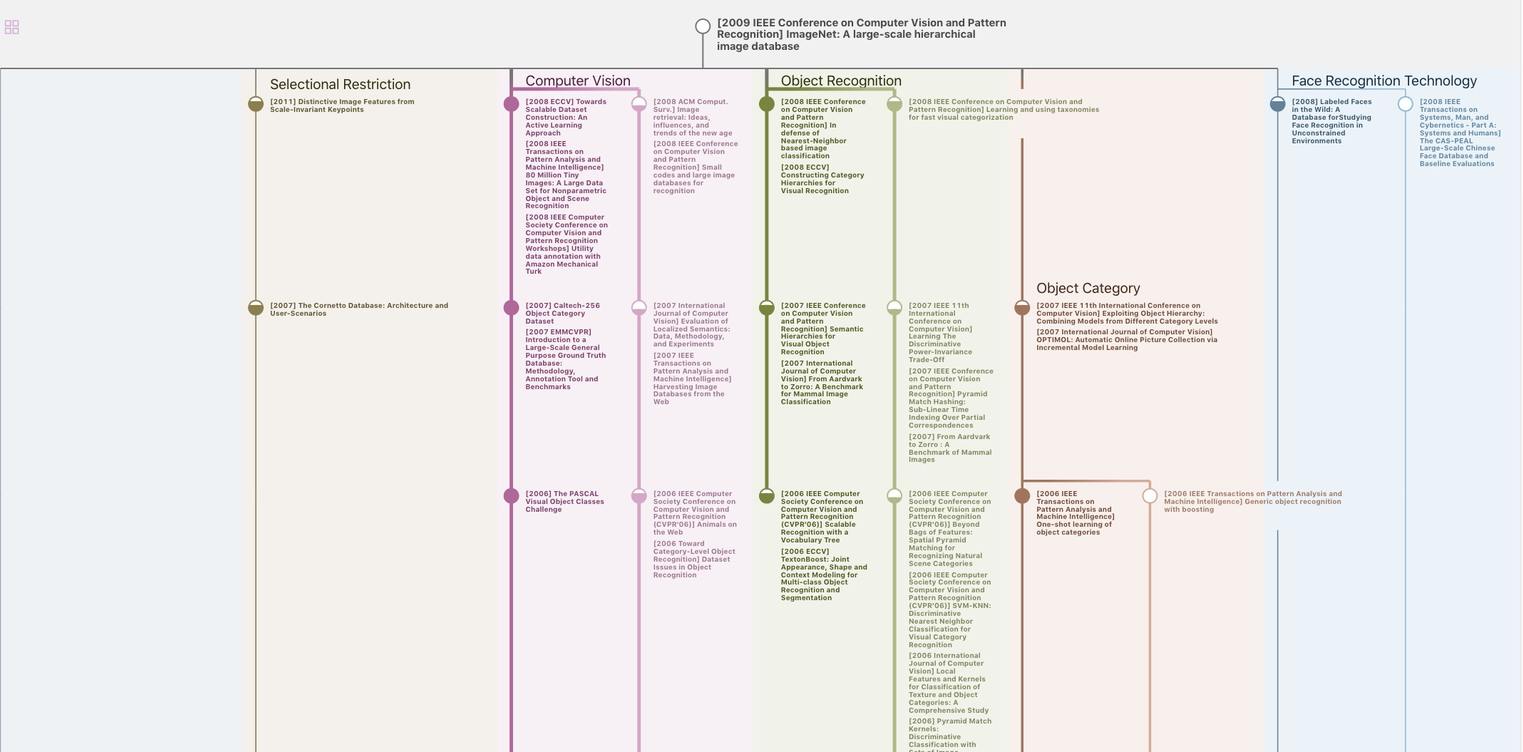
生成溯源树,研究论文发展脉络
Chat Paper
正在生成论文摘要