Proactive Content Caching for Internet-of-Vehicles based on Peer-to-Peer Federated Learning
2020 IEEE 26th International Conference on Parallel and Distributed Systems (ICPADS)(2020)
摘要
To cope with the increasing content requests from emerging vehicular applications, caching contents at edge nodes is imperative to reduce service latency and network traffic on the Internet-of-Vehicles (IoV). However, the inherent characteristics of IoV, including the high mobility of vehicles and restricted storage capability of edge nodes, cause many difficulties in the design of caching schemes. Driven by the recent advancements in machine learning, learning-based proactive caching schemes are able to accurately predict content popularity and improve cache efficiency, but they need gather and analyse users' content retrieval history and personal data, leading to privacy concerns. To address the above challenge, we propose a new proactive caching scheme based on peer-to-peer federated deep learning, where the global prediction model is trained from data scattered at vehicles to mitigate the privacy risks. In our proposed scheme, a vehicle acts as a parameter server to aggregate the updated global model from peers, instead of an edge node. A dual-weighted aggregation scheme is designed to achieve high global model accuracy. Moreover, to enhance the caching performance, a Collaborative Filtering based Variational AutoEncoder model is developed to predict the content popularity. The experimental results demonstrate that our proposed caching scheme largely outperforms typical baselines, such as Greedy and Most Recently Used caching.
更多查看译文
关键词
Federated Learning,Deep Learning,Internet of Vehicles,Edge Caching,AutoEncoder
AI 理解论文
溯源树
样例
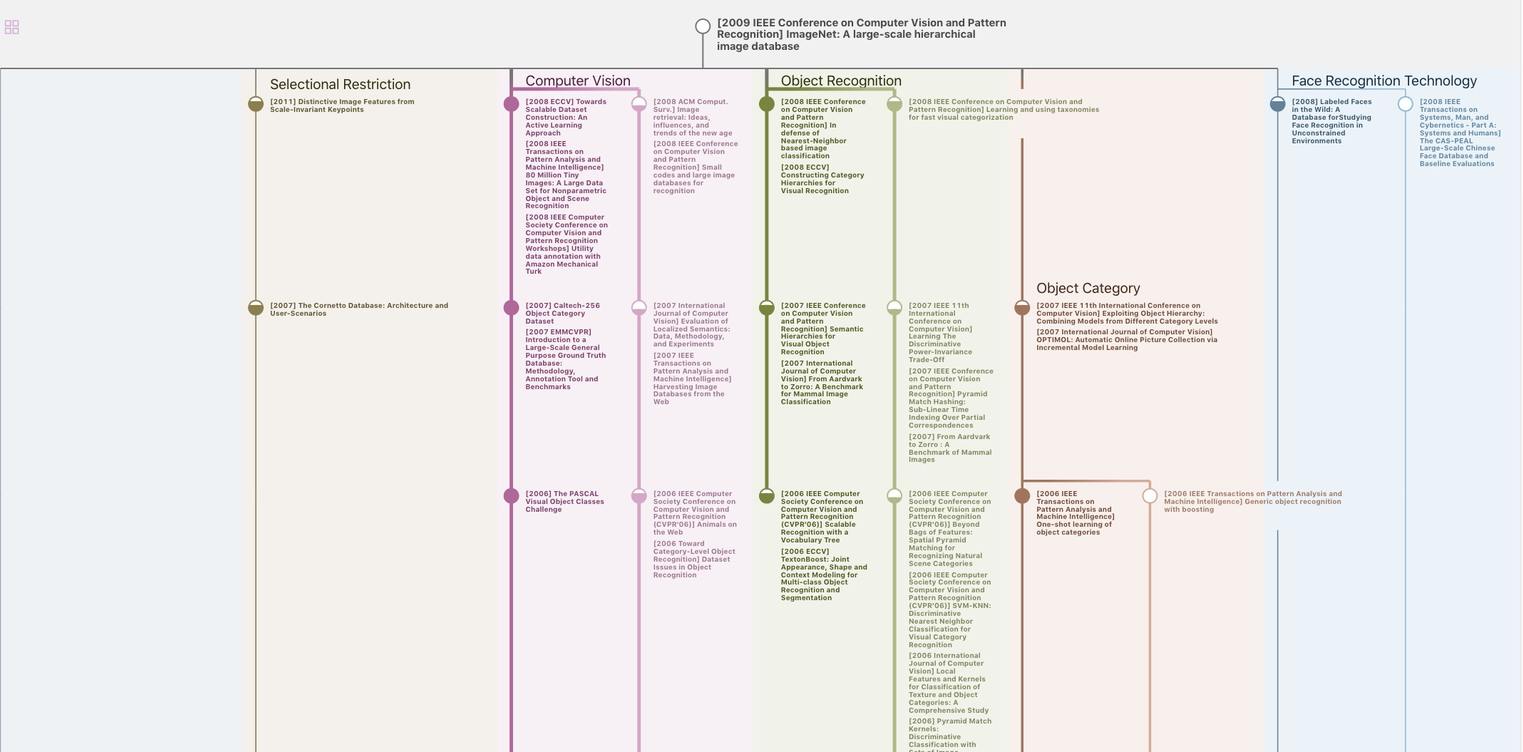
生成溯源树,研究论文发展脉络
Chat Paper
正在生成论文摘要