Generalization of iterative sampling in autoencoders.
ICMLA(2020)
摘要
Generative autoencoders are designed to model a target distribution with the aim of generating samples and it has also been shown that specific non-generative autoencoders (i.e. contractive and denoising autoencoders) can be turned into generative models using reinjections (i.e. iterative sampling). In this work, we provide mathematical evidence that any autoencoder reproducing the input data with a loss of information can sample from the training distribution using reinjections. More precisely, we prove that the property of modeling a given distribution and sampling from it not only applies to contractive and denoising autoencoders but also to all lossy autoencoders. In accordance with previous results, we emphasize that the reinjection sampling procedure in autoencoders improves the quality of the sampling. We experimentally illustrate the above property by generating synthetic data with non-generative autoencoders trained on standard datasets. We show that the learning curve of a classifier trained with synthetic data is similar to that of a classifier trained with original data.
更多查看译文
关键词
Iterative sampling,autoencoders,denoising au-toencoders,reinjection,sampling
AI 理解论文
溯源树
样例
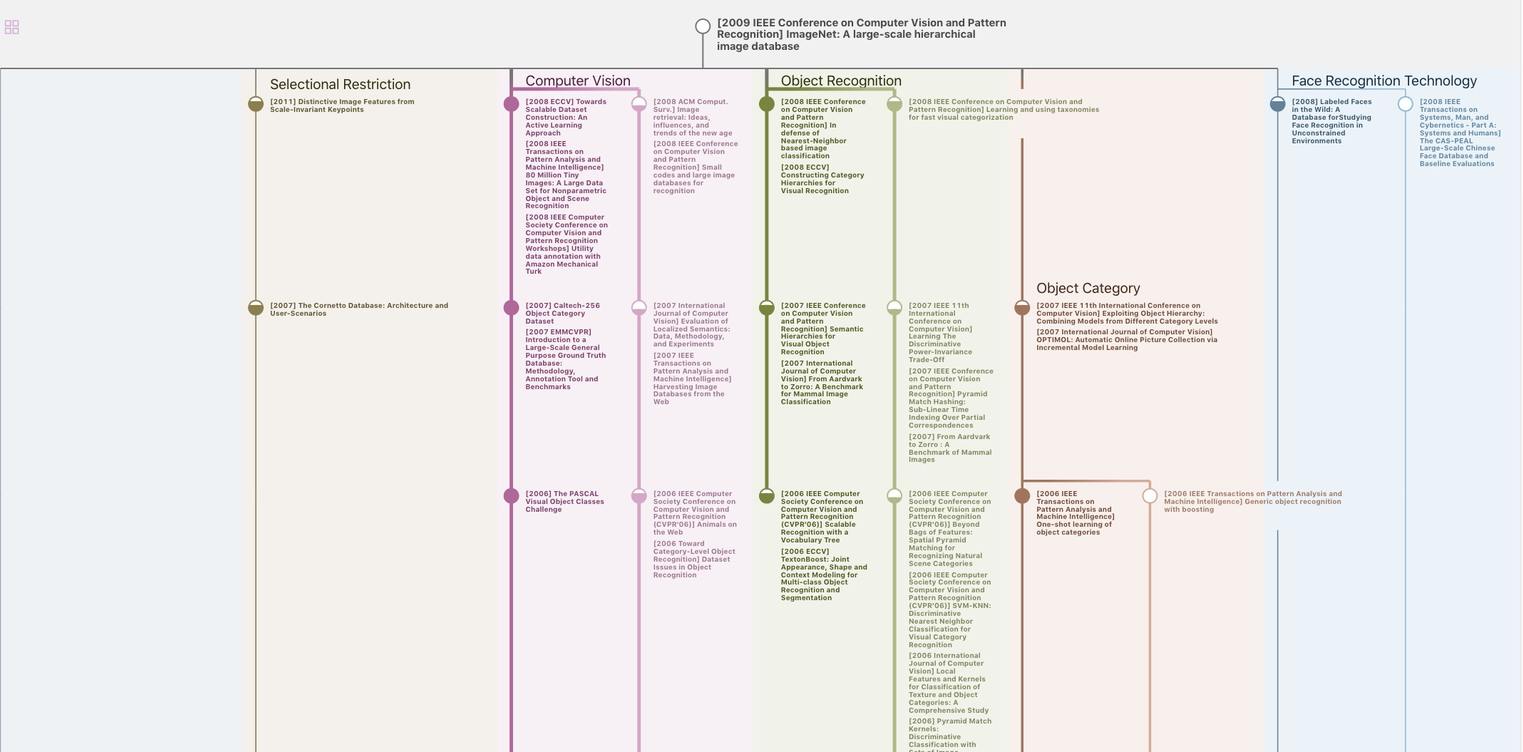
生成溯源树,研究论文发展脉络
Chat Paper
正在生成论文摘要