Sequential Transfer Learning for Event Detection and Key Sentence Extraction.
ICMLA(2020)
摘要
Sequential transfer learning (STL) techniques aim to improve learning in the target task by leveraging knowledge from a related domain using pre-trained representations. Approaches based on these techniques have achieved state-of-the-art results on a wide range of natural language processing (NLP) tasks. In the context of event detection and key sentence extraction, we propose to explore STL-based techniques using the last generation of pre-trained language representations, namely, ALBERT, BERT, DistilBERT, ELECTRA, OpenAI GPT2, RoBERTa and, XLNet. Experiments are conducted as a part of our contribution to the CLEF 2019 ProtestNews Track, which aims to classify and identify protest events in English-language news from India and China. Averaged results show that a STL-based method with OpenAI GPT2 outperforms prevailing methods in this domain by achieving better performance across event detection and key sentence extraction tasks. In addition, OpenAI GPT2 also obtains the best results on the majority of datasets tested in comparison to the best system presented during the CLEF 2019 ProtestNews challenge.
更多查看译文
关键词
transfer learning,pre-trained language models,neural networks,event detection
AI 理解论文
溯源树
样例
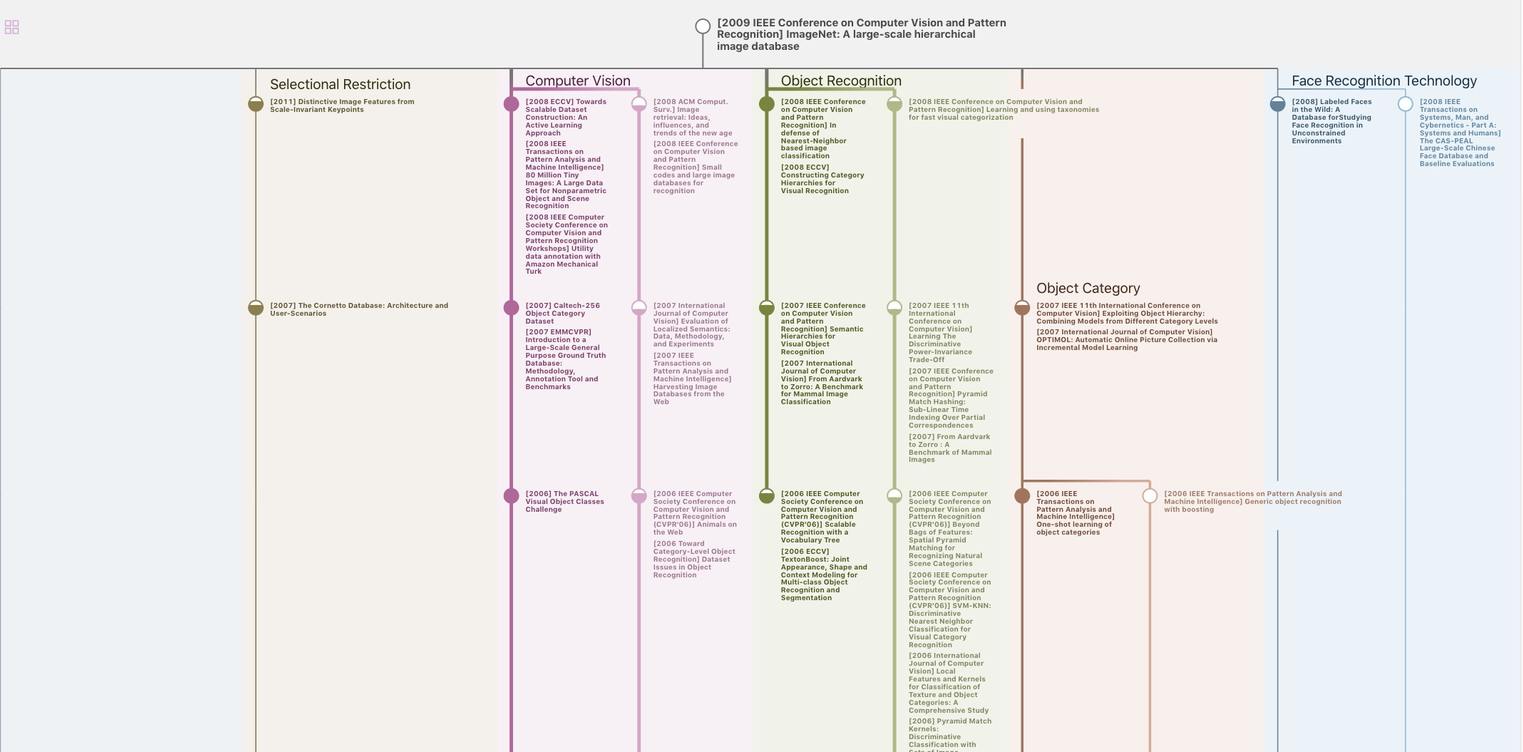
生成溯源树,研究论文发展脉络
Chat Paper
正在生成论文摘要