Changing The Geometry Of Representations: Alpha-Embeddings For Nlp Tasks
ENTROPY(2021)
摘要
Word embeddings based on a conditional model are commonly used in Natural Language Processing (NLP) tasks to embed the words of a dictionary in a low dimensional linear space. Their computation is based on the maximization of the likelihood of a conditional probability distribution for each word of the dictionary. These distributions form a Riemannian statistical manifold, where word embeddings can be interpreted as vectors in the tangent space of a specific reference measure on the manifold. A novel family of word embeddings, called alpha-embeddings have been recently introduced as deriving from the geometrical deformation of the simplex of probabilities through a parameter alpha, using notions from Information Geometry. After introducing the alpha-embeddings, we show how the deformation of the simplex, controlled by alpha, provides an extra handle to increase the performances of several intrinsic and extrinsic tasks in NLP. We test the alpha-embeddings on different tasks with models of increasing complexity, showing that the advantages associated with the use of alpha-embeddings are present also for models with a large number of parameters. Finally, we show that tuning alpha allows for higher performances compared to the use of larger models in which additionally a transformation of the embeddings is learned during training, as experimentally verified in attention models.
更多查看译文
关键词
word embeddings, alpha-embeddings, information geometry, attention mechanism
AI 理解论文
溯源树
样例
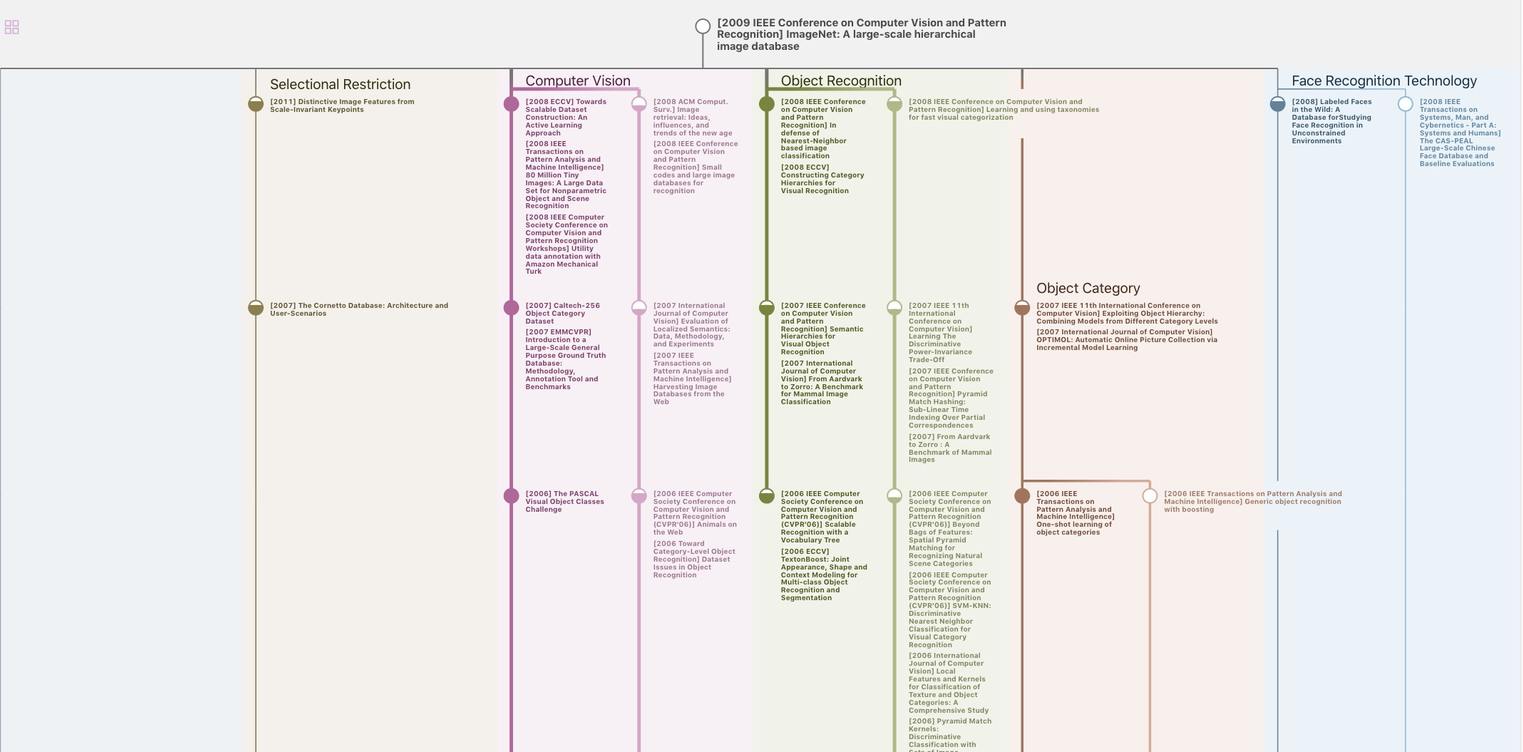
生成溯源树,研究论文发展脉络
Chat Paper
正在生成论文摘要