A LSTM-Based Channel Fingerprinting Method for Intrusion Detection
2021 IEEE 5th International Conference on Cryptography, Security and Privacy (CSP)(2021)
摘要
Intrusion detection is a crucial issue for 5th generation (5G) access networks to securely support various services. Traditional cryptographic key-based solutions are not suitable for severe resources-constrained networks, such as the Internet of Things (IoT). In this paper, we propose a lightweight intrusion detection mechanism by exploring physical layer attributes that are unique and difficult to impersonate. Specifically, a long-short memory network (LSTM) is employed as an intelligent classifier to distinguish different transmitters based on channel state information (CSI) features. Then we develop a comprehensive 5G NR channel detection model based on LSTM under dynamic channel conditions to identify malicious attacks by intelligently analyzing CSI. The simulation results demonstrate that the proposed solution improves detection accuracy and successfully prevent systems from spoofing attacks.
更多查看译文
关键词
LSTM,physical layer security,detection,5G,new radio
AI 理解论文
溯源树
样例
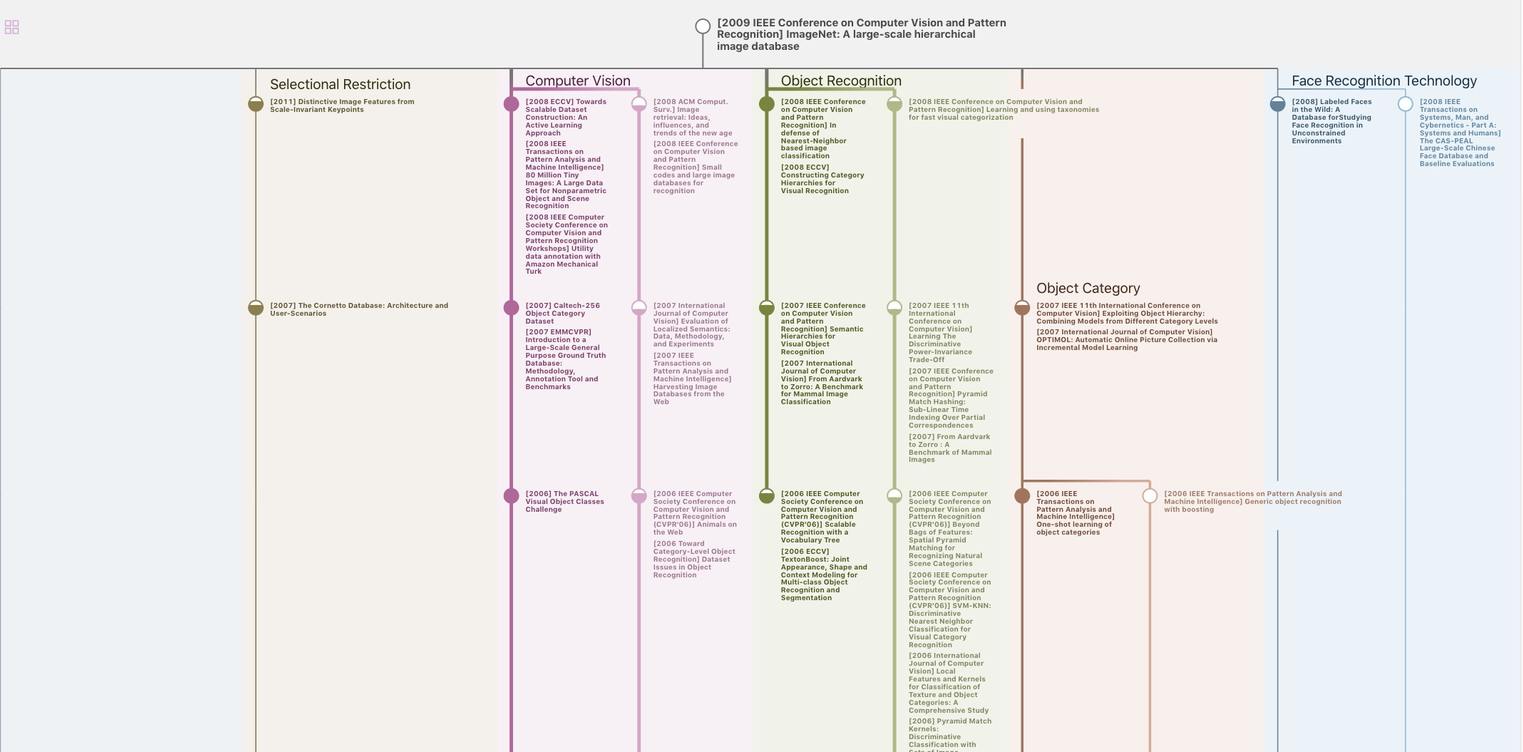
生成溯源树,研究论文发展脉络
Chat Paper
正在生成论文摘要