Effect Of Interaction Design Of Reinforcement Learning Agents On Human Satisfaction In Partially Observable Domains
HUCAPP: PROCEEDINGS OF THE 16TH INTERNATIONAL JOINT CONFERENCE ON COMPUTER VISION, IMAGING AND COMPUTER GRAPHICS THEORY AND APPLICATIONS - VOL. 2: HUCAPP(2021)
摘要
Interactive machine learning involves humans teaching with agents during their learning process. As this field grows, it is pertinent that laymen teachers, i.e. those without programming or extensive ML experience, are able to easily and effectively teach the agents. Previous work has investigated which factors contribute to the teacher's experience when training agents in a fully observable domain. In this paper, we investigate how four different interaction methods affect agent performance and teacher experience in partially observable domains. As the domain in which the agent is learning becomes more complex, it accumulates less reward overall and needs more advice from the teacher. It is found that the most salient features that affect teacher satisfaction are agent compliance to advice, response speed, instruction quantity required, and reliability in agent response. It is suggested that machine learning algorithms incorporate a short time delay in the agent's response and maximize the agent's adherence to advice to increase reliability of the agent's behavior. The need to generalize advice over time to reduce the amount of instruction needed varies depending on the presence of penalties in the environment.
更多查看译文
关键词
Human-robot Interaction, Interactive Reinforcement Learning, Interactive Machine Learning, Algorithm Design, User Experience
AI 理解论文
溯源树
样例
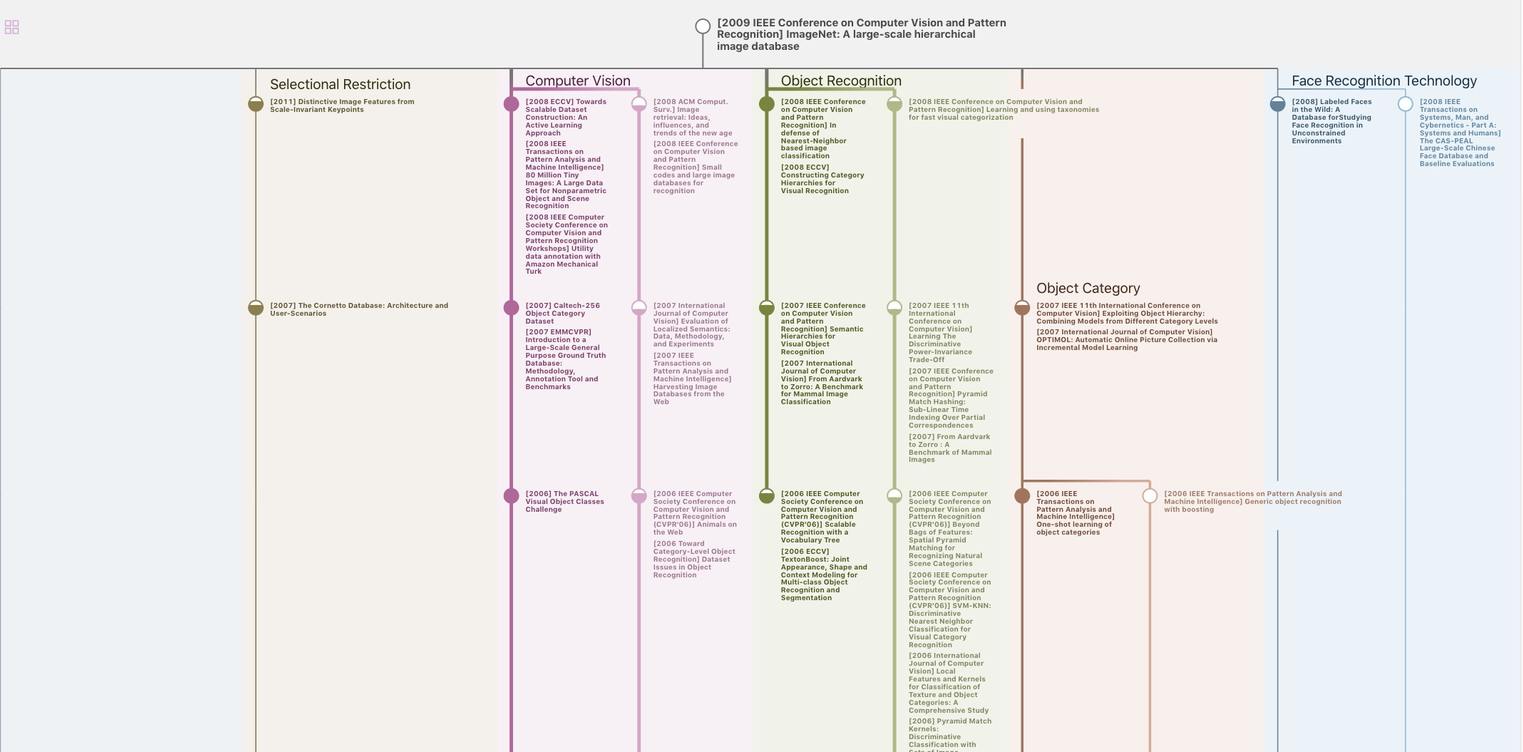
生成溯源树,研究论文发展脉络
Chat Paper
正在生成论文摘要